Open Access
ARTICLE
Effective Latent Representation for Prediction of Remaining Useful Life
Shanghai Jiaotong University, Shanghai, 200240, China
* Corresponding Author: Gang Wu. Email:
Computer Systems Science and Engineering 2021, 36(1), 225-237. https://doi.org/10.32604/csse.2021.014100
Received 31 August 2020; Accepted 19 October 2020; Issue published 23 December 2020
Abstract
AI approaches have been introduced to predict the remaining useful life (RUL) of a machine in modern industrial areas. To apply them well, challenges regarding the high dimension of the data space and noisy data should be met to improve model efficiency and accuracy. In this study, we propose an end-to-end model, termed ACB, for RUL predictions; it combines an autoencoder, convolutional neural network (CNN), and bidirectional long short-term memory. A new penalized root mean square error loss function is included to avoid an overestimation of the RUL. With the CNN-based autoencoder, a high-dimensional data space can be mapped into a lower-dimensional latent space, and the noisy data can be greatly reduced. We compared ACB with five state-of-the-art models on the Commercial Modular Aero-Propulsion System Simulation dataset. Our model achieved the lowest score value on all four sub-datasets. The robustness of our model to noise is also supported by the experiments.Keywords
Cite This Article
Citations
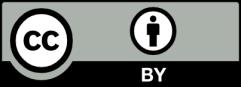