Open Access
ARTICLE
SRC: Superior Robustness of COVID-19 Detection from Noisy Cough Data Using GFCC
1 Department of Computer Science & Engineering, Government College of Engineering, Kalahandi, Bhawanipatna, 766002, India
2 Department of Computer Science and Information, Taibah University, Medina, 42353, Saudi Arabia
3 School of Information Technology and Engineering, VIT University Vellore, 632014, Tamil Nadu, India
4 Computer Science Department, College of Computer Science and Engineering, Taibah University, Medina, 42353, Saudi Arabia
* Corresponding Author: Mohammad Zubair Khan. Email:
Computer Systems Science and Engineering 2023, 46(2), 2337-2349. https://doi.org/10.32604/csse.2023.036192
Received 20 September 2022; Accepted 08 December 2022; Issue published 09 February 2023
Abstract
This research is focused on a highly effective and untapped feature called gammatone frequency cepstral coefficients (GFCC) for the detection of COVID-19 by using the nature-inspired meta-heuristic algorithm of deer hunting optimization and artificial neural network (DHO-ANN). The noisy crowdsourced cough datasets were collected from the public domain. This research work claimed that the GFCC yielded better results in terms of COVID-19 detection as compared to the widely used Mel-frequency cepstral coefficient in noisy crowdsourced speech corpora. The proposed algorithm's performance for detecting COVID-19 disease is rigorously validated using statistical measures, F1 score, confusion matrix, specificity, and sensitivity parameters. Besides, it is found that the proposed algorithm using GFCC performs well in terms of detecting the COVID-19 disease from the noisy crowdsourced cough dataset, COUGHVID. Moreover, the proposed algorithm and undertaken feature parameters have improved the detection of COVID-19 by 5% compared to the existing methods.Keywords
Cite This Article
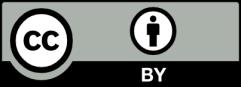