Open Access
ARTICLE
Classification of Gastric Lesions Using Gabor Block Local Binary Patterns
1 College of Computing and Informatics, Saudi Electronic University, Riyadh, 11673, Saudi Arabia
2 College of Electrical and Mechanical Engineering, National University of Sciences and Technology, Islamabad, Pakistan
3 Department of Computer Science, National University of Sciences and Technology, Balochistan Campus, Quetta, Pakistan
* Corresponding Author: Muhammad Tahir. Email:
Computer Systems Science and Engineering 2023, 46(3), 4007-4022. https://doi.org/10.32604/csse.2023.032359
Received 15 May 2022; Accepted 04 August 2022; Issue published 03 April 2023
Abstract
The identification of cancer tissues in Gastroenterology imaging poses novel challenges to the computer vision community in designing generic decision support systems. This generic nature demands the image descriptors to be invariant to illumination gradients, scaling, homogeneous illumination, and rotation. In this article, we devise a novel feature extraction methodology, which explores the effectiveness of Gabor filters coupled with Block Local Binary Patterns in designing such descriptors. We effectively exploit the illumination invariance properties of Block Local Binary Patterns and the inherent capability of convolutional neural networks to construct novel rotation, scale and illumination invariant features. The invariance characteristics of the proposed Gabor Block Local Binary Patterns (GBLBP) are demonstrated using a publicly available texture dataset. We use the proposed feature extraction methodology to extract texture features from Chromoendoscopy (CH) images for the classification of cancer lesions. The proposed feature set is later used in conjuncture with convolutional neural networks to classify the CH images. The proposed convolutional neural network is a shallow network comprising of fewer parameters in contrast to other state-of-the-art networks exhibiting millions of parameters required for effective training. The obtained results reveal that the proposed GBLBP performs favorably to several other state-of-the-art methods including both hand crafted and convolutional neural networks-based features.Keywords
Cite This Article
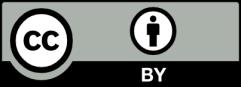