Open Access
ARTICLE
COVID TCL: A Joint Metric Loss Function for Diagnosing COVID-19 Patient in the Early and Incubation Period
1 Department of Electromechanical Engineering, Zhejiang Business Technology Institute, Ningbo, 315012, China
2 Engineering Research Center of Digital Forensics, Ministry of Education, Jiangsu Engineering Center of Network Monitoring, School of Computer and Software, Nanjing University of Information Science & Technology, Nanjing, 210044, China
3 Wuxi Research Institute, Nanjing University of Information Science & Technology, Wuxi, 214100, China
4 Jiangsu Collaborative Innovation Center of Atmospheric Environment and Equipment Technology (CICAEET), Nanjing University of Information Science & Technology, Nanjing, 210044, China
5 Department of Physics, Faculty of Science, Adani University, Ahmedabad, India
* Corresponding Author: Rui Wen. Email:
Computer Systems Science and Engineering 2023, 47(1), 187-204. https://doi.org/10.32604/csse.2023.037889
Received 20 November 2022; Accepted 17 February 2023; Issue published 26 May 2023
Abstract
Convolution Neural Networks (CNN) can quickly diagnose COVID-19 patients by analyzing computed tomography (CT) images of the lung, thereby effectively preventing the spread of COVID-19. However, the existing CNN-based COVID-19 diagnosis models do consider the problem that the lung images of COVID-19 patients in the early stage and incubation period are extremely similar to those of the non-COVID-19 population. Which reduces the model’s classification sensitivity, resulting in a higher probability of the model misdiagnosing COVID-19 patients as non-COVID-19 people. To solve the problem, this paper first attempts to apply triplet loss and center loss to the field of COVID-19 image classification, combining softmax loss to design a jointly supervised metric loss function COVID Triplet-Center Loss (COVID-TCL). Triplet loss can increase inter-class discreteness, and center loss can improve intra-class compactness. Therefore, COVID-TCL can help the CNN-based model to extract more discriminative features and strengthen the diagnostic capacity of COVID-19 patients in the early stage and incubation period. Meanwhile, we use the extreme gradient boosting (XGBoost) as a classifier to design a COVID-19 images classification model of CNN-XGBoost architecture, to further improve the CNN-based model’s classification effect and operation efficiency. The experiment shows that the classification accuracy of the model proposed in this paper is 97.41%, and the sensitivity is 97.61%, which is higher than the other 7 reference models. The COVID-TCL can effectively improve the classification sensitivity of the CNN-based model, the CNN-XGBoost architecture can further improve the CNN-based model’s classification effect.Keywords
Cite This Article
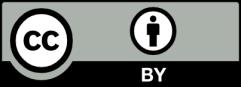