Open Access
ARTICLE
Improved Blending Attention Mechanism in Visual Question Answering
1 School of Automation, University of Electronic Science and Technology of China, Chengdu, 610054, China
2 College of Resource and Environment Engineering, Guizhou University, Guiyang, 550025, China
3 School of Data Science and Artificial Intelligence, Wenzhou University of Technology, Wenzhou, 325000, China
4 School of Public Affairs and Administration, University of Electronic Science and Technology of China, Chengdu, 611731, China
5 Department of Geography and Anthropology, Louisiana State University, Baton Rouge, 70803, LA, USA
* Corresponding Authors: Mingzhe Liu. Email: ; Wenfeng Zheng. Email:
Computer Systems Science and Engineering 2023, 47(1), 1149-1161. https://doi.org/10.32604/csse.2023.038598
Received 20 December 2022; Accepted 10 April 2023; Issue published 26 May 2023
Abstract
Visual question answering (VQA) has attracted more and more attention in computer vision and natural language processing. Scholars are committed to studying how to better integrate image features and text features to achieve better results in VQA tasks. Analysis of all features may cause information redundancy and heavy computational burden. Attention mechanism is a wise way to solve this problem. However, using single attention mechanism may cause incomplete concern of features. This paper improves the attention mechanism method and proposes a hybrid attention mechanism that combines the spatial attention mechanism method and the channel attention mechanism method. In the case that the attention mechanism will cause the loss of the original features, a small portion of image features were added as compensation. For the attention mechanism of text features, a self-attention mechanism was introduced, and the internal structural features of sentences were strengthened to improve the overall model. The results show that attention mechanism and feature compensation add 6.1% accuracy to multimodal low-rank bilinear pooling network.Keywords
Cite This Article
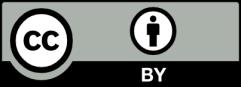