Open Access
ARTICLE
Stock Market Prediction Using Generative Adversarial Networks (GANs): Hybrid Intelligent Model
1 Department of Management Information Systems, Ataturk University, Erzurum, 25030, Turkey
2 Department of Management Information Systems, Karadeniz Technical University, Trabzon, 61080, Turkey
* Corresponding Author: Fares Abdulhafidh Dael. Email:
Computer Systems Science and Engineering 2023, 47(1), 19-35. https://doi.org/10.32604/csse.2023.037903
Received 21 November 2022; Accepted 09 February 2023; Issue published 26 May 2023
Abstract
The key indication of a nation’s economic development and strength is the stock market. Inflation and economic expansion affect the volatility of the stock market. Given the multitude of factors, predicting stock prices is intrinsically challenging. Predicting the movement of stock price indexes is a difficult component of predicting financial time series. Accurately predicting the price movement of stocks can result in financial advantages for investors. Due to the complexity of stock market data, it is extremely challenging to create accurate forecasting models. Using machine learning and other algorithms to anticipate stock prices is an interesting area. The purpose of this article is to forecast stock market values to assist investors to make better informed and precise investing decisions. Statistics, Machine Learning (ML), Natural language processing (NLP), and sentiment analysis will be used to accomplish the study’s objectives. Using both qualitative and quantitative information, the study developed a hybrid model. The hybrid model has been handled with GANs. Based on the model’s predictions, a buy-or-sell trading strategy is offered. The conclusions of this study will assist investors in selecting the ideal choice while selling, holding, or buying shares.Keywords
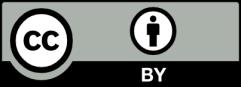