Open Access
ARTICLE
A Robust Approach for Detection and Classification of KOA Based on BILSTM Network
1 Computer Science Department, UET, Taxila, Pakistan
2 Department of Information Technology, College of Computer, Qassim University, Buraydah, Saudi Arabia
* Corresponding Author: Suliman Aladhadh. Email:
Computer Systems Science and Engineering 2023, 47(2), 1365-1384. https://doi.org/10.32604/csse.2023.037033
Received 20 October 2022; Accepted 24 February 2023; Issue published 28 July 2023
Abstract
A considerable portion of the population now experiences osteoarthritis of the knee, spine, and hip due to lifestyle changes. Therefore, early treatment, recognition and prevention are essential to reduce damage; nevertheless, this time-consuming activity necessitates a variety of tests and in-depth analysis by physicians. To overcome the existing challenges in the early detection of Knee Osteoarthritis (KOA), an effective automated technique, prompt recognition, and correct categorization are required. This work suggests a method based on an improved deep learning algorithm that makes use of data from the knee images after segmentation to detect KOA and its severity using the Kellgren-Lawrence (KL classification schemes, such as Class-I, Class-II, Class-III, and Class-IV. Utilizing ResNet to segregate knee pictures, we first collected features from these images before using the Bidirectional Long Short-Term Memory (BiLSTM) architecture to classify them. Given that the technique is a pre-trained network and doesn’t require a large training set, the Mendeley VI dataset has been utilized for the training of the proposed model. To evaluate the effectiveness of the suggested model, cross-validation has also been employed using the Osteoarthritis Initiative (OAI) dataset. Furthermore, our suggested technique is more resilient, which overcomes the challenge of imbalanced training data due to the hybrid architecture of our proposed model. The suggested algorithm is a cutting-edge and successful method for documenting the successful application of the timely identification and severity categorization of KOA. The algorithm showed a cross-validation accuracy of 78.57% and a testing accuracy of 84.09%. Numerous tests have been conducted to show that our suggested algorithm is more reliable and capable than the state-of-the-art at identifying and categorizing KOA disease.Keywords
Cite This Article
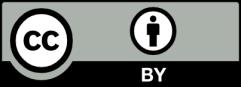