Open Access
ARTICLE
Hybridized Intelligent Neural Network Optimization Model for Forecasting Prices of Rubber in Malaysia
1 Faculty of Electrical and Electronic Engineering, Universiti Tun Hussein Onn Malaysia, Parit Raja, 86400, Malaysia
2 School of Mathematical Sciences, Universiti Sains Malaysia, Penang, 11800, Malaysia
3 School of General & Foundation Studies, AIMST University, Bedong, 08100, Malaysia
* Corresponding Author: Saratha Sathasivam. Email:
Computer Systems Science and Engineering 2023, 47(2), 1471-1491. https://doi.org/10.32604/csse.2023.037366
Received 01 November 2022; Accepted 02 February 2023; Issue published 28 July 2023
Abstract
Rubber producers, consumers, traders, and those who are involved in the rubber industry face major risks of rubber price fluctuations. As a result, decision-makers are required to make an accurate estimation of the price of rubber. This paper aims to propose hybrid intelligent models, which can be utilized to forecast the price of rubber in Malaysia by employing monthly Malaysia’s rubber pricing data, spanning from January 2016 to March 2021. The projected hybrid model consists of different algorithms with the symbolic Radial Basis Functions Neural Network k-Satisfiability Logic Mining (RBFNN-kSAT). These algorithms, including Grey Wolf Optimization Algorithm, Artificial Bee Colony Algorithm, and Particle Swarm Optimization Algorithm were utilized in the forecasting data analysis. Several factors, which affect the monthly price of rubber, such as rubber production, total exports of rubber, total imports of rubber, stocks of rubber, currency exchange rate, and crude oil prices were also considered in the analysis. To evaluate the results of the introduced model, a comparison has been conducted for each model to identify the most optimum model for forecasting the price of rubber. The findings showed that GWO with RBFNN-kSAT represents the most accurate and efficient model compared with ABC with RBFNN-kSAT and PSO with RBFNN-kSAT in forecasting the price of rubber. The GWO with RBFNN-kSAT obtained the greatest average accuracy (92%), with a better correlation coefficient R = 0.983871 than ABC with RBFNN-kSAT and PSO with RBFNN-kSAT. Furthermore, the empirical results of this study provided several directions for policymakers to make the right decision in terms of devising proper measures in the industry to address frequent price changes so that the Malaysian rubber industry maintains dominance in the international markets.Keywords
Cite This Article
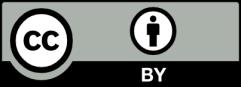