Open Access
ARTICLE
Sand Cat Swarm Optimization with Deep Transfer Learning for Skin Cancer Classification
1 Department of Electronics and Instrumentation Engineering, V. R. Siddhartha Engineering College, Vijayawada, 520007, India
2 Department of Software Engineering, College of Computer Science and Engineering, University of Jeddah, Jeddah, Saudi Arabia
3 Department of Computer Science and Engineering, University Institute of Engineering and Technology (UIET), Guru Nanak University, Hyderabad, India
4 Department of Computer Science and Engineering, Vignan’s Institute of Information Technology, Visakhapatnam, 530049, India
5 Department of Applied Data Science, Noroff University College, Kristiansand, Norway
6 Artificial Intelligence Research Center (AIRC), College of Engineering and Information Technology, Ajman University, Ajman, United Arab Emirates
7 Department of Electrical and Computer Engineering, Lebanese American University, Byblos, Lebanon
8 Department of Software, Kongju National University, Cheonan, 31080, Korea
* Corresponding Author: Jungeun Kim. Email:
Computer Systems Science and Engineering 2023, 47(2), 2079-2095. https://doi.org/10.32604/csse.2023.038322
Received 07 December 2022; Accepted 02 February 2023; Issue published 28 July 2023
Abstract
Skin cancer is one of the most dangerous cancer. Because of the high melanoma death rate, skin cancer is divided into non-melanoma and melanoma. The dermatologist finds it difficult to identify skin cancer from dermoscopy images of skin lesions. Sometimes, pathology and biopsy examinations are required for cancer diagnosis. Earlier studies have formulated computer-based systems for detecting skin cancer from skin lesion images. With recent advancements in hardware and software technologies, deep learning (DL) has developed as a potential technique for feature learning. Therefore, this study develops a new sand cat swarm optimization with a deep transfer learning method for skin cancer detection and classification (SCSODTL-SCC) technique. The major intention of the SCSODTL-SCC model lies in the recognition and classification of different types of skin cancer on dermoscopic images. Primarily, Dull razor approach-related hair removal and median filtering-based noise elimination are performed. Moreover, the U2Net segmentation approach is employed for detecting infected lesion regions in dermoscopic images. Furthermore, the NASNetLarge-based feature extractor with a hybrid deep belief network (DBN) model is used for classification. Finally, the classification performance can be improved by the SCSO algorithm for the hyperparameter tuning process, showing the novelty of the work. The simulation values of the SCSODTL-SCC model are scrutinized on the benchmark skin lesion dataset. The comparative results assured that the SCSODTL-SCC model had shown maximum skin cancer classification performance in different measures.Keywords
Cite This Article
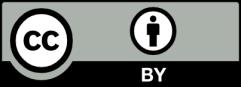