Open Access
ARTICLE
CT-NET: A Novel Convolutional Transformer-Based Network for Short-Term Solar Energy Forecasting Using Climatic Information
1 Department of Software Convergence, Sejong University, Seoul, 143-747, Korea
2 Department of Convergence Engineering for Intelligent Drone, Sejong University, Seoul, 143-747, Korea
* Corresponding Author: Sung Wook Baik. Email:
Computer Systems Science and Engineering 2023, 47(2), 1751-1773. https://doi.org/10.32604/csse.2023.038514
Received 15 December 2022; Accepted 20 April 2023; Issue published 28 July 2023
Abstract
Photovoltaic (PV) systems are environmentally friendly, generate green energy, and receive support from policies and organizations. However, weather fluctuations make large-scale PV power integration and management challenging despite the economic benefits. Existing PV forecasting techniques (sequential and convolutional neural networks (CNN)) are sensitive to environmental conditions, reducing energy distribution system performance. To handle these issues, this article proposes an efficient, weather-resilient convolutional-transformer-based network (CT-NET) for accurate and efficient PV power forecasting. The network consists of three main modules. First, the acquired PV generation data are forwarded to the pre-processing module for data refinement. Next, to carry out data encoding, a CNN-based multi-head attention (MHA) module is developed in which a single MHA is used to decode the encoded data. The encoder module is mainly composed of 1D convolutional and MHA layers, which extract local as well as contextual features, while the decoder part includes MHA and feedforward layers to generate the final prediction. Finally, the performance of the proposed network is evaluated using standard error metrics, including the mean squared error (MSE), root mean squared error (RMSE), and mean absolute percentage error (MAPE). An ablation study and comparative analysis with several competitive state-of-the-art approaches revealed a lower error rate in terms of MSE (0.0471), RMSE (0.2167), and MAPE (0.6135) over publicly available benchmark data. In addition, it is demonstrated that our proposed model is less complex, with the lowest number of parameters (0.0135 M), size (0.106 MB), and inference time (2 ms/step), suggesting that it is easy to integrate into the smart grid.Keywords
Cite This Article
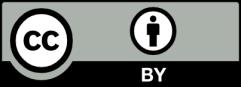