Open Access
ARTICLE
Business Blockchain Suitability Determinants: Decision-Making through an Intuitionistic Fuzzy Method
1 Department of Information Systems, College of Computer and Information Sciences, King Saud University, Riyadh, 11451, Saudi Arabia
2 Department of Quantitative Methods, School of Business, King Faisal University, Al-Ahsa, 31982, Saudi Arabia
3 Faculty of Computer Science and Engineering, King Salman International University, South Sinai, Egypt
* Corresponding Author: Tomader Almeshal. Email:
Computer Systems Science and Engineering 2023, 47(2), 1665-1690. https://doi.org/10.32604/csse.2023.038871
Received 01 January 2023; Accepted 10 April 2023; Issue published 28 July 2023
Abstract
Blockchain is one of the innovative and disruptive technologies that has a wide range of applications in multiple industries beyond cryptocurrency. The widespread adoption of blockchain technology in various industries has shown its potential to solve challenging business problems, as well as the possibility to create new business models which can increase a firm’s competitiveness. Due to the novelty of the technology, whereby many companies are still exploring potential use cases, and considering the complexity of blockchain technology, which may require huge changes to a company’s existing systems and processes, it is important for companies to carefully evaluate suitable use cases and determine if blockchain technology is the best solution for their specific needs. This research aims to provide an evaluation framework that determines the important dimensions of blockchain suitability assessment by identifying the key determinants of suitable use cases in a business context. In this paper, a novel approach that utilizes both qualitative (Delphi method) and quantitative (fuzzy set theory) methods has been proposed to objectively account for the uncertainty associated with data collection and the vagueness of subjective judgments. This work started by scanning available literature to identify major suitability dimensions and collected a range of criteria, indicators, and factors that had been previously identified for related purposes. Expert opinions were then gathered using a questionnaire to rank the importance and relevance of these elements to suitability decisions. Subsequently, the data were analyzed and we proceeded to integrate multi-criteria group decision-making (MCGDM) and intuitionistic fuzzy set (IFS) theory. The findings demonstrated a high level of agreement among experts, with the model being extremely sensitive to variances in expert assessments. Furthermore, the results helped to refine and select the most relevant suitability determinants under three important dimensions: functional suitability of the use case, organizational applicability, and ecosystem readiness.Keywords
Among emerging technologies that have recently attracted attention, blockchain, or distributed ledger technology (DLT), is considered a powerful business enhancer that has the potential to disrupt many business sectors with game-changing effects in different industries [1,2]. It has significantly impacted industries other than financial services, as various applications have also evolved in logistics and supply chain management, insurance, government, education, energy, agriculture and healthcare [3,4]. Applications built on blockchain technology benefit from secure transactions, fair access, transparency, immutability, and other features that help address hotspots in new business technologies as a result of blockchain’s excellent reliability. The security and trustworthiness of blockchain have encouraged business owners and practitioners to explore blockchain opportunities beyond cryptocurrency, motivating them to investigate how they can benefit from the technology.
Due to the distinctive properties of blockchain technology, extravagant claims have developed around new potential business applications. As a result, according to the Deloitte report [5], only 11% of blockchain projects were able to move from the proof-of-concept stage to achieve implementation, and only 8% of blockchain projects were successfully running [6,7]. This implies that blockchain may not be suitable or beneficial in all business cases. It indicates that the decision to adopt such a disruptive technology needs deep analysis and that multiple factors should be taken into consideration. In addition, it is a time-consuming decision-making process due to the lack of knowledge and experience associated with the novelty of this technology [8]. The findings from available studies indicate that there is a shortage in blockchain suitability frameworks as most of the existing methodologies have been designed to assess the applicability of blockchain for predetermined industries or are focused on a single point of view [9]. In addition, researchers have often stated that blockchain technology implementation has not yet reached maturity and more research is still required [10,11]. All of the aforementioned findings have motivated this work to develop a holistic framework to help evaluate the suitability of blockchain for certain business cases. Thus, this paper proposes an approach that can identify the most important determinants of suitable blockchain use cases and seeks to address two critical questions:
(1) What are the main dimensions that impact blockchain suitability decisions?
(2) What are the key determinants falling under each dimension that can effectively evaluate the suitability of a use case for a blockchain solution?
To answer these questions, this paper contributes to the knowledge base by achieving a number of objectives:
■ Identifying a broad range of evaluation criteria by scanning the available literature to collect previously identified criteria. These criteria were then revised and divided into three groups, each of which was used for a distinct part of the evaluation.
■ Proposing a novel Suitability Evaluation Framework for Enterprise Blockchain (SEF-EB), which combines the three groups of criteria as the framework’s main dimensions. Each dimension incorporates multiple determinants that are used to evaluate use case suitability with respect to (1) functional requirements, (2) organizational applicability, and (3) ecosystem readiness.
■ Designing a decision-making approach that integrates MCGDM with IFS theory through a fuzzy Delphi methodology. This approach aims to select the most important determinants for blockchain suitability and eliminate irrelevant ones.
■ Addressing both the uncertainty of group decision-making and the ambiguity that experts may encounter when selecting from a large list of determinants due to the aggregation technique of experts’ judgments that are simultaneously handled with fuzzy methodology.
The findings of this research may enable decision-makers in government, business enterprises, and technology consulting firms to make more informed decisions when blockchain adoption is under assessment. Indeed, there is still no agreement between practitioners and scholars on the conditions for suitable blockchain use cases. Hence, to the best of our knowledge, this work offers a novel approach for evaluating blockchain suitability by determining the key elements that directly affect decisions. This approach adopts a generic lens applicable to various industries and offers a holistic evaluation of the main dimensions of the problem. In addition, the adapted methodology considers the impact of knowledge diversification among experts and derives a methodology that aggregates experts’ opinions and their respective weights. This paper is organized to provide an overview of the literature on blockchain suitability evaluation. Then, it introduces the proposed conceptual framework SEF-EB. After that, our methodology for developing the decision model is described in detail and supported with a real methodology application. For validation purposes, we provide a comparative analysis with a group decision-making approach that utilizes Triangular Fuzzy Numbers (TFNs) and neglects the experts’ weights. Finally, we discuss the business implications and propose expanded ideas for future research.
This paper is part of ongoing research that aims to develop a knowledge-based framework for evaluating the suitability of blockchain technology for business cases. In the early stages of the project, we conducted a scoping review [9] as a primary step to explore state-of-the-art suitability evaluations concerned with blockchain technology. The scoping review was carried out to discover how the suitability of blockchain is measured or evaluated and to gather evaluation criteria to utilize for the assessments through the investigation of both research papers and gray literature. This step resulted in the identification of many evaluation approaches and the collection of several criteria, indicators, and factors for suitability evaluations. Thus, we were able to identify various methodologies for evaluating the applicability of blockchain, which further enabled us to determine the major dimensions of effective evaluations. In the following sections, we summarize our observations of previous literature, and we highlight the identified research gaps.
The number of studies addressing the evaluation and applicability of blockchain, or assessing blockchain’s feasibility in a business context, is increasing, as reported in [9]. The authors claim that, since 2019, there has been a noticeable increase in publications addressing the issue of blockchain suitability evaluations, which reflects the interests of business and industry in researching blockchain applications. We notice that most earlier publications tend to focus on proposing evaluation criteria that are applicable for use as decision tree nodes or flowchart for example, the flowcharts proposed in [1,12–19]. Others proposed multi-layered frameworks that consider the capabilities and attributes of blockchain technology [20,21] or consider technical and managerial issues [22–24]. In addition, the most recently proposed methodologies recommend approaching the issue as a multi-criteria decision-making problem (MCDM). Thus, different mathematical models and rank-weight methods have been used intensively in [25–34]. As a result, several decision-making criteria have been suggested. These criteria include elements from the technological, business, regulatory, social, and usability domains [35–37].
However, substantial limitations have been reported in the literature, including a lack of consensus between researchers and professionals over what constitutes “suitability” and how to identify suitability measurement criteria. The majority of suggested frameworks consider the assessment from a single point of view; that is, they either address the applicability of blockchain technology with use case functional requirements [27,38–41], assess the potential of adopting blockchain technology within an organization [42–45] or evaluate the feasibility of utilizing blockchain solutions within an intended ecosystem [28,46–49]. Moreover, there is an absence of a generic evaluation framework that addresses these issues regardless of the application area or industry. However, the sector-focused evaluation frameworks in place have constrained the effects and advantages of the proposed solutions. Therefore, we have worked to fill this gap in the literature and offer a unified generic blockchain suitability framework that addresses the topic holistically by handling both the social and technical dimensions and is unrestricted by industry-specific requirements.
Additional limitations have arisen due to the methodologies utilized to generate evaluation criteria or to implement decision models such as MCDM. Indeed, the terms and concepts extracted from the wide range of literature create ambiguity because each resource has utilized its own terminology and explanations. This problem was reduced by expert validation and consultation, but this approach introduced additional methodological challenges: i) how to deal with the background and experience of experts with different perspectives, and ii) how to deal with the haziness of human judgments, which are inherently surrounded by doubt.
In this study, we address the gaps mentioned above by offering the SEF-EB framework. As stated above, a scoping review was conducted to analyze the methodologies of available suitability evaluation frameworks and elicit and extract vast sets of evaluation determinants (criteria, factors, and indicators). These determinants were then categorized and clustered into three suitability dimensions, as shown in the following section. We then developed an MCGDM technique that assisted in identifying the most important determinants that could impact how effectively blockchain suitability decisions are made. Additionally, we attempted to handle the fuzziness and uncertainty presented by the novelty of blockchain technology by adopting a fuzzy Delphi methodology that integrates MCGDM and IFS theory.
3 The Proposed Suitability Evaluation Framework for Enterprise Blockchain
In this section, we provide an overview of the proposed conceptual framework for blockchain suitability with an illustration of its main dimensions. Our conceptualization of the blockchain suitability evaluation framework for business use cases comprises three main dimensions as shown in Fig. 1:
1) The suitability of blockchain for the functional requirements of a use case, which includes an assessment of how blockchain can serve to optimize use case elements, such as data processing, transaction management, and users’ interactions.
2) The suitability of the organization proposing to adopt a blockchain solution, which involves an assessment of different aspects of its infrastructure, such as management support, financial strength, technical capabilities, and other factors influencing successful adoption.
3) The readiness of the ecosystem surrounding the organization, which involves an assessment of the socio-technical factors that could impact the decision to adopt blockchain solutions. This includes the capabilities of participants, the regulatory and legal environment, and the availability of solution providers.
Figure 1: The conceptual framework for blockchain suitability evaluation in an enterprise context
Accordingly, we refined and restructured the collected evaluation determinants to propose a definition of business blockchain suitability. This step involved creating groups of related determinants and clustering them into main components and sub-components before assigning them to the relevant suitability dimension. Additionally, this step involved redefining the meaning of determinant and assigning new terminologies to reflect the goal of a particular dimension. The new definitions are provided in suppl. A1 and these terminologies were presented to an expert to ensure their relevance and competence. The suitability dimensions with their associated group of determinants are presented in the following sub-sections.
3.1 Use Case Functional Suitability
Several of the collected items were proposed to assure that the primary use case requirements could be implemented through blockchain technology, and that blockchain had sufficient capabilities to meet the required functionalities. We were able to identify 19 determinants, or criteria, that were used to check functional suitability. These criteria were classified into three categories Fig. 2. The first group consists of criteria related to use case participants and their relationships. The second group addresses the required properties of use case data and assets. Finally, the third group of criteria tackles use case transactions and ensures that blockchain can meet the needs that have been identified.
Figure 2: Functional suitability dimension
3.2 Organizational Applicability
The second dimension of blockchain suitability evaluation involves evaluating the applicability of an organization that plans to implement a blockchain solution. In this dimension, we identify 23 determinants, or indicators, related to organizational characteristics and then classify them into five categories: management acceptance, technical and financial capabilities, organizational behavior, and legislation boundaries [50,51]. These indicators are presented in Fig. 3.
Figure 3: Organizational applicability dimension
In the last dimension of blockchain suitability evaluation, we grouped those items that address the ecosystem and are used to assess its readiness for supporting business entities that decide to adopt blockchain solutions. We collected 13 factors, which were clustered into four categories related to governments, business entities, solution providers, and finally, end users. Fig. 4 illustrates these categories and show their main factors.
Figure 4: Ecosystem readiness dimension
As previously mentioned, our conceptual framework incorporates three dimensions, each of which contributes to the process of blockchain suitability assessment. Although the determinants under each dimension were extracted from the available research, we needed to validate our selections and make sure that these determinants (criteria, indicators, and factors), were relevant to our suitability decision problem. To that end, we addressed the problem of blockchain suitability evaluation as an MCGDM problem, comprising three dimensions which form the components of the suitability conceptual framework. Then, we propose an the intuitionistic fuzzy weighted average (IFWA) with the Delphi to select the most important components. In the following section, we provide a description of our methodology.
To identify the most important components under each blockchain suitability dimension, we used the identified criteria, indicators, and factors as decision items to generate a practical decision model. We proposed an MCGDM approach that utilizes a Delphi method [52] to identify the significant decision items. In order to address the vagueness and uncertainty surrounding the topic and to account for the fuzziness in group decision-making, we propose a novel methodology that combines IFWA with the Delphi method. This methodology is novel, and to the best of our knowledge, no previous work has addressed the evaluation of blockchain suitability for business cases with a similar approach. Our proposed methodology comprises eight steps, beginning by developing and constructing the decision model instruments, which have already been derived from the conceptual framework. Then, it determines the set of linguistic variables, identifies the group of experts, and assigns their weights. Subsequently, we move to the data collection process where experts’ opinions are gathered then to multiple calculation steps that are used to determine the importance of each criterion by aggregating experts’ inputs with their proficiency levels. The Delphi method and the intuitionistic fuzzy set (IFS) basic definitions, rules and arithmetic operations are firstly reviewed to clarify the methodology calculations.
The Delphi method was developed in the 1950s and early 1960s at the Rand Corporation [52]. The first applications of the Delphi method were in the field of science and technology forecasting. One of its earliest uses was to advise the U.S. Army Air Corps on future technological capabilities that might be employed by the military [53]. The Delphi method is defined as a decision-making approach that obtains consensus among a panel of experts on a specific issue by acquiring a consistent flow of answers via questionnaires with controlled feedback on input from the experts [54–56,57]. However, several modifications and improvements have been proposed to enhance the Delphi method [58,59]. One of the major improvements to the traditional Delphi method is the introduction of linguistic variable concepts as a base for fuzzy set (FS) theory and intuitionistic fuzzy set (IFS) theory. These theories have helped to initiate different approaches to the fuzzy Delphi method that have attempted to interpret the uncertainty involved in experts’ opinions [60]. Moreover, the traditional Delphi method has obvious weaknesses including its subjectivity and time-consuming nature [56]. That because the traditional Delphi method needs repetitive surveys to achieve consensus among experts with differing opinions, and the experts’ judgments cannot be appropriately reflected the fuzziness associated with the human cognitive process [55,56]. Therefore, The Fuzzy Delphi method developed by Ishikawa (1993) [61] strengthens the traditional Delphi method by reducing the number of rounds required to carry out the study, and it better supports modeling the uncertainty associated with human judgments [56].
4.2 Fuzzy Set and Intuitionistic Fuzzy Set Theories
In any decision-making process, a critical problem is how to express expert judgments as preference values. Due to the increasing complexity of the world and the subjective nature of human thinking, it is difficult for decision-makers (experts) to provide exact decisions, as there is always imprecise, vague, or uncertain information. To deal with this, Zadeh (1965) introduced fuzzy set (FS) theory [62] in which preferences are expressed as LV and transformed into fuzzy numbers. This allows for the calculation of degrees of membership more easily than a crisp number. For example, fuzzy set
4.3 The Proposed Methodology Steps
Step 1: Construct and validate the fuzzy Delphi instruments
The criteria defined in our conceptual framework are formulated as a questionnaire with four parts. The first part is an introductory section that asks experts to provide geral information about their backgrounds and years of experience. This part is essential for obtaining experts’ weights. The three remaining parts are related to the three dimensions of our conceptual frameworks: functionaluitability, organizational applicability, and ecosystem readiness. This questionnaire is used to gather experts’ judgments regarding each criterion and determine how important it is to consider this element when a decisioabout the suitability of use cases is under assessment. After drafting, the survey was subjected to a validity test to eliminate potential problems and make them as concise as possible. This process improved the reliability of the questionnaire by eliminating ambiguous or difficult questions that may lead to unreliable answers.
Step 2: Identify the expert panel.
Collaborative decision-making is one of the most important sources of creativity and accuracy [66]. Therefore, the determination of the expert’s group is essential to guarantee that the selected specialist can provide a valid viewpoint within the context of the study. However, a large number of experts may increase the complexity of the fuzzy Delphi method. Thus, we tried to limit the number of participating experts to between 7–12, as this is the ideal number of decision-makers recommended by [52]. In this study, 8 experts
Step 3: Determine linguistic variables
In this step, we defined the linguistic variables and their corresponding intuitionistic fuzzy numbers (IFNs). Linguistic variables of five-level linguistic terms were employed to (a) evaluate the importance of the criteria and (b) rank the experts’ knowledge. Table 1 shows the linguistic scales and corresponding IFNs, which are adopted from [66].
Step 4: Gather experts’ opinions.
In this step, we asked the group of experts to fill out our online survey by specifying the level of importance of each criterion listed in the questionnaire. E evaluated the importance of the criteria using linguistic variables represented by IFNs. The result of this step was a decision matrix that consisted of the criteria
The outcome of this step was a decision matrix represented as follows:
where:
Step 5: Calculate experts’ weights.
importance of each expert
The equivalent IFN was then used to calculate the average weight of each expert as follows:
Assume
Step 6: Aggregate the experts’ assessments.
All the experts’ judgments were combined into one group judgment by aggregating all individual judgments into a group decision matrix
where
Step 7: Ranking the IF group evaluation assessments based on crisp values
In this step, we converted the IF group evaluation assessments (
where
Step 8: Select significant criteria.
We screened the frameworks’ criteria based on threshold values to select the significant criteria. By setting a threshold
If
If
A threshold value for criterion m is defined as follows:
where
The proposed methodology, based on IF-DM, was applied to determine the most important determinants of blockchain’s suitability for business cases. After constructing the online questionnaire and identifying the expert panel
After obtaining the pairwise matrices, we moved to the aggregation step where we aggregated all the experts’ assessments into one group decision matrix
Lastly, to select the most significant determinants (criteria/indicators/factors) about whose relevance and importance most experts reached a consensus, we set a threshold value φ using Eq. (12) for each framework dimension as shown in the bottom row of Tables 2–4. We then screened all items defined in our blockchain suitability framework based on the resulting threshold vaes. As a result, our proposed method selected 33 out of 55 items that were important for suitability evaluation as shown in the right column (decision) of the tables below. The selected items were disseminated between the three dimensions as follows: 10 criteria for evaluating functional suitability, 16 indicators for assessing organizational applicability, and 7 factors for measuring ecosystem readiness.
In this section, for validation purposes, we compare our methodology with the existing fuzzy Delphi method proposed in [58], by applying that methodology to our data. Before comparing the results, we will illustrate their approach and highlight the main differences between the two methodologies. Then, we will compare the outcomes of the two methodologies, emphasizing the contrast in the results of the accepted and rejected criteria.
Firstly, both methods begin with designing a survey and defining the expert panel, followed by obtaining experts’ opinions using a set of linguistic variables. Subsequently, the authors transform the linguistic variables into TFNs; alternatively, in our proposed method, we use IFNs. The TFNs do not involve hesitation, which can be encountered in real-life MCGDM problems, while the IFNs are more efficient and capable of dealing with hesitation in real-world decision-making processes [71]. Secondly, in the defuzzification process, they use the centroid of gravity (COG) method, whereas we use Guo’s measure—based on the IFWA operator—to find the aggregated weights of each identified criterion. Additionally, we consider the weights of the experts in our aggregation method, enabling us to gauge the significance of the criteria by giving more qualified and experienced experts a chance to influence the outcomes.
As presented in Table 5, both methodologies suggest almost the same set of acceptable criteria. This confirms that our approach is consistent and that it is capable of emulating the results expected from utilizing the fuzzy Delphi method. For a more precise comparison, the graphical representation of the results is shown in Figs. 5–10. Nevertheless, our approach outperforms because of its ability to influence the results through the judgment of qualified experts. For example, criteria C3.6 (scalability and performance) was accepted in our decision model, but it was rejected by the comparing model. In light of the original judgments provided by our experts, we found that a lower-weighted expert deemed this criterion “unimportant,” whereas higher-weighted experts deemed this criterion “important” or “very important.” This clearly shows that our model was able to outbalance this insignificant judgment and to consider the opinions of the majority and of superior experts. A similar scenario happened with IN2.1 (Proportion of digital assets) and F4.2 (Ecosystem value proposition). In IN2.1, five out of eight experts deemed this indicator “very important”; the remaining experts chose “moderate” (two experts) and “unimportant” (one expert). This result consequently indicates that our model is adequate for capturing and balancing judgments by considering a number of sophisticated aspects.
Figure 5: Results from the proposed methodology for functional suitability criteria
Figure 6: Results of the compared methodology for functional suitability criteria
Figure 7: Results of the proposed methodology for organization applicability indicators
Figure 8: Results of the compared methodology for organization applicability indicators
Figure 9: Results of the proposed methodology for ecosystem readiness factors
Figure 10: Results of the compared methodology for ecosystem readiness factors
In conclusion, the differences between the two fuzzy set types (TFNs and IFNs) might cause a slight variance in the results, owing to the fact that IF theory lowers the degree of hesitation [72]. We argue that our proposed methodology might be preferable for solving MCGDM problems to deal with the hesitation caused by insufficient information from the decision-makers.
The proposed research determined the three essential dimensions of the blockchain suitability evaluation framework and identified lists of suitability determinants, which were classified as follows: functional suitability criteria, organizational applicability indicators, and ecosystem readiness factors. These determinants were exhaustively gathered, refined, and validated by a group of blockchain experts using the fuzzy Delphi method. In addition, we used the IFS theory to address the ambiguity of the decision-making process and to enable the determination of a group consensus with few hesitations. In the following paragraph, we highlight significant results and discuss their implications for business decisions when the implementation of a blockchain solution is under consideration. The discussion will emphasize the crucial factors for evaluating blockchain suitability that arise from the application of our methodology. We then try to align our findings with previous research dedicated to exploring blockchain suitability evaluation issues.
For the first dimension, we gathered 19 criteria that are mostly used to evaluate how blockchain is suitable for a use case’s functional requirements. Our experts selected 10 criteria for assessing blockchain’s functional suitability in relation to a given use case. Based on the experts’ rankings, the three most important criteria were as follows: the existence of trust issues, the need to ensure data integrity and transparency, and the need for traceability mechanisms for auditing purposes. Additionally, the experts pointed to the relevance of the multiplicity of business entities, which allows them to share a system with a decentralized database that could automatically perform their business transactions with data immutability guarantees. These results were also confirmed by the majority of existing publications [13,20,21,25,27,39,41,73].
Based on our findings, developing a use case using blockchain technology was not justified by the need to enhance privacy, maintain data governance, or make plans to get rid of third parties. The experts also minimized the significance of criteria such as needs of asset valuation, transaction cost reduction, and the development of reward and incentive mechanisms as reasons to use blockchain technology. However, our results might not be compatible with other research (e.g., [19,15,16,21]) for multiple reasons. This incompatibility may be due to the fact that understated criteria were suggested for sector-specific use cases, whereas our experts attempted to select important criteria that could be generalized for suitability assessment—for example, the need for blockchain to eliminate the role of third parties and thereby reduce costs. Although removing third parties is one of the advantages of enterprise blockchain, it was not a significant aspect of blockchain suitability decisions. Another justification for this incompatibility could be due to the cause-and-effect causal relationship that is not elaborated by this method [50,51]. Furthermore, the experts disagreed on the importance of asset tokenization in determining suitability; discussing aspects such as tokenization takes the discussion outside the scope of our research and redirects the use of blockchain as a cryptocurrency enabler.
Moving to the second dimension, our proposed framework suggested evaluating the organization’s applicability toward adopting blockchain applications. We collected 23 indicators that could be used for this purpose, and our experts selected 16 indicators as the most important. The research findings indicate that the most applicable organizations are those firms that operate with a transparent culture, encourage innovation, and aim to enhance competitive performance. In addition, applicable organizations should have a blockchain strategy that is supported by senior management and therefore has financial support. This support can be attributed to the perceived financial benefits of adopting blockchain in a way that mitigates risk and uncertainty in the organization’s business strategy. Moreover, the availability of technical infrastructure and know-how are also essential for determining applicability. Indeed, a company would be more applicable to the implementation of blockchain technology if it had governance structures and regulatory compliance that managed the enterprise consortium with transparent incentive structures.
Contrary findings indicate that applicability will decrease if the organization intends to implement blockchain for limited objectives such as enhancing security or improving auditability. In addition, one of the interesting results is that competitive pressure is not an influencing factor, although it has been proven to be an accelerator for the adoption of many other innovative technologies. This result demonstrates that blockchain technology is still in its early stages and that the applications have not yet matured to the point where they can dominate the technology market.
The last dimension of the blockchain suitability framework is the evaluation of the targeted ecosystem’s readiness. The ecosystem is a combination of homogeneous entities that compatibly work together in the same environment to achieve their goals. In this study, we classified ecosystem entities into four categories: government, business firms, solution providers, and customers or end users. We then identified 13 factors that could influence an ecosystem’s readiness to support blockchain projects or consortiums and our experts selected 9 factors that are important in assessing an ecosystem’s readiness to facilitate any blockchain initiatives. The most important factor is the availability of technology providers that offer competitive services, which saves time and effort for firms aiming to acquire the necessary infrastructure. The richness of business entities and the presence of enthusiastic participants are also critical factors, in addition to the regulations that organize participants’ incentive structures and guarantee participants’ rights. Although the experts agreed that it was critical to establish particular blockchain regulations, they did not agree on the relevance of government leadership as a component of ecosystem readiness. Additionally, the findings demonstrated that competitiveness between business entities in the same ecosystem is not considered a readiness factor, proving once more that blockchain has not yet produced a superior performance that boosts company competitiveness.
In the proposed approach, substantial limitations have been recognized, such as the number of professionals with varied or limited experience, which may affect the holistic sense of providing generalized judgments. All the accepted items represent the decisions of a group of experts (i.e., policymakers, practitioners, and academics) who are high-level decision-makers with vast experience in the industry of innovative technology. Their opinions represent what they expect from blockchain, and it could vary, or change based on the timing period or context. However, this work could be expanded in the future to address the interdependency between the identified determinants through an application of other MCDM models such as models proposed in [74], or by considering other factors or dimensions [45]. Moreover, the findings of this work can be adopted to make business decisions regarding the use of blockchain, that is by utilizing it as a base rule for a blockchain recommendation tool that helps in evaluating the suitability of blockchain.
This work proposes an evaluation approach for the suitability of blockchain for business use cases. This is done by proposing a generic, unified conceptual framework SEF-EB for assessing blockchain suitability, aided by a decision model to identify the significant suitability determinants. The findings of this research show that blockchain suitability is a multi-dimensional problem. Assessment of the suitability of a particular use case requires precise analysis that deeply investigates the actual functionality of the use case vs. blockchain capabilities. At the same time, the organization’s suitability regarding the implementation of blockchain solutions must be broadly examined. In addition, this analysis delves further into the surrounding environment and explores whether the intended ecosystem is ready to facilitate collaborative blockchain consortiums. For each of the identified blockchain suitability dimensions, we gathered the most crucial determinants to assess the use case’s functional suitability, organization applicability, and ecosystem readiness. Subsequently, a novel technique IFWA-Delphi utilizing a fuzzy Delphi method integrated with group decision-making and IFS was used to determine the key characteristics of successful blockchain cases. In conclusion, this work offers a distinctive approach to evaluating blockchain suitability. The significant advantage and the novelty of this research are summarized as follows:
■ Findings from both literature and gray literature have been used to identify a large group of blockchain suitability determinants. These determinants have been meticulously collected, refined, and categorized to serve the various assessment perspectives.
■ This research addresses the absence of a holistic evaluation approach that can help to determine business use case suitability for blockchain technology. Our conceptual framework SEF-EB comprises all of the aspects involved in the suitability evaluation process including the functional, organizational, or ecosystem determinants. Thus, it offers the foundational knowledge needed to provide a general framework that can be applied to multiple industries.
■ This research proposed a novel approach for selecting the most relevant determinants by utilizing the IFS-Delphi method that objectively accounts for the uncertainty associated with the novelty of the topic and addresses the vagueness of group decisions.
■ It is also considering the impact of knowledge diversification among experts to develop a methodology that aggregates experts’ opinions and their respective weights by utilizing the enhanced approach of the IFS-Delphi method, which is the IFWA-Delphi method.
■ The implementation of the method resulted in a substantial level of consensus among the experts, and great sensitivity to opinion variance.
■ The outcomes of this research could help various decision-makers employed in government, business enterprises and technology consulting firms make more informed decisions when blockchain adoption is under assessment.
Acknowledgement: This research project was supported by a grant from the “Research Center of College of Computer and Information Sciences” Deanship of Scientific Research, King Saud University.
Funding Statement: The authors received no specific funding for this study.
Conflicts of Interest: The authors declare that they have no conflicts of interest to report regarding the present study.
1The criteria description available on https://github.com/Tomader-msh/TAM
2The decision matrices available on https://github.com/Tomader-msh/TAM
References
1. B. Betzwieser, S. Franzbonenkamp, T. Riasanow, M. Böhm, H. Kienegger et al., “A decision model for the implementation of blockchain solutions,” in 25th Americas Conf. on Information Systems (AMCIS), Cancun, Mexico, 2019. [Google Scholar]
2. N. El Madhoun, J. Hatin and E. Bertin, “Going beyond the blockchain hype: In which cases are blockchains useful for IT applications?” in 2019 3rd Cyber Security in Networking Conf., CSNet, Quito, Ecuador, pp. 21–27, 2019. https://doi.org/10.1109/CSNet47905.2019.9108966 [Google Scholar] [CrossRef]
3. A. A. Arsyad, I. W. Widayat and M. Köppen, “Supporting farming smart documentation system by modular blockchain solutions,” Decision Making: Applications in Management and Engineering, vol. 5, no. 1, pp. 1–26, 2022, https://doi.org/10.31181/dmame0326022022a [Google Scholar] [CrossRef]
4. J. Zhou, Y. Wu, F. Liu, Y. Tao and J. Gao, “Prospects and obstacles analysis of applying blockchain technology to power trading using a deeply improved model based on the DEMATEL approach,” Sustainable Cities and Society, vol. 70, pp. 102910, 2021, https://doi.org/10.1016/j.scs.2021.102910 [Google Scholar] [CrossRef]
5. J. Lear Trujillo, S. Fromhart and V. Srinivas, “The evolution of blockchain technology,” Deloitte Insigts, 2017. [Online]. Available: https://www2.deloitte.com/us/en/insights/industry/financial-services/evolution-of-blockchain-github-platform.html [Google Scholar]
6. S. Balasubramanian, V. Shukla, J. S. Sethi, N. Islam and R. Saloum, “A readiness assessment framework for blockchain adoption: A healthcare case study,” Technological Forecasting and Social Change, vol. 165, no. January, pp. 120536, 2021, https://doi.org/10.1016/j.techfore.2020.120536 [Google Scholar] [CrossRef]
7. Rimol, M., “Gartner 2019 hype cycle for blockchain business shows blockchain will have a transformational impact across industries in five to 10 years,” 2019. [Online]. Available: https://www.gartner.com/en/newsroom/press-releases/2019-09-12-gartner-2019-hype-cycle-for-blockchain-business-shows [Google Scholar]
8. N. K. Ostern, F. Holotiuk and J. Moormann, “Organizations’ approaches to blockchain: A critical realist perspective,” Information and Management, vol. 59, no. 7, pp. 103552, 2022, https://doi.org/10.1016/j.im.2021.103552 [Google Scholar] [CrossRef]
9. T. A. Almeshal and A. A. Alhogail, “Blockchain for businesses: A scoping review of suitability evaluations frameworks,” IEEE Access, vol. 9, pp. 155425–155442, 2021. https://doi.org/10.1109/ACCESS.2021.3128608 [Google Scholar] [CrossRef]
10. S. Smetanin, A. Ometov, M. Komarov, P. Masek and Y. Koucheryavy, “Blockchain evaluation approaches: State-of-the-art and future perspective,” Sensors, vol. 20, no. 12, pp. 1–20, 2020. https://doi.org/10.3390/s20123358 [Google Scholar] [PubMed] [CrossRef]
11. J. Aslam, A. Saleem, N. T. Khan and Y. B. Kim, “Factors influencing blockchain adoption in supply chain management practices: A study based on the oil industry,” Journal of Innovation & Knowledge, vol. 6, no. 2, pp. 124–134, 2021. https://doi.org/10.1016/j.jik.2021.01.002 [Google Scholar] [CrossRef]
12. M. E. Peck, “Blockchain world-do you need a blockchain? This chart will tell you if the technology can solve your problem,” IEEE Spectrum, vol. 54, no. 10, pp. 38–60, 2017. [Google Scholar]
13. K. Wust and A. Gervais, “Do you need a blockchain?” in Proc.-2018 Crypto Valley Conf. on Blockchain Technology, CVCBT, Zug, Switzerland, pp. 45–54, 2018. https://doi.org/10.1109/CVCBT.2018.00011 [Google Scholar] [CrossRef]
14. N. Emmadi, R. Vigneswaran, S. Kanchanapalli, L. Maddali and H. Narumanchi, “Practical deployability of permissioned blockchains,” Lecture Notes in Business Information Processing, vol. 339, pp. 229–243, 2019. https://doi.org/10.1007/978-3-030-04849-5_21 [Google Scholar] [CrossRef]
15. S. K. Lo, X. Xu, Y. K. Chiam and Q. Lu, “Evaluating suitability of applying blockchain,” in Proc. of the IEEE Int. Conf. on Engineering of Complex Computer Systems, ICECCS, Melbourne, Australia, pp. 158–161, 2018. https://doi.org/10.1109/ICECCS.2017.26 [Google Scholar] [CrossRef]
16. M. J. M. Chowdhury, A. Colman, M. A. Kabir, J. Han and P. Sarda, “Blockchain versus database: A critical analysis,” in 17th IEEE Int. Conf. on Trust, Security and Privacy in Computing, New York, NY, USA, pp. 1348–1353, 2018. https://doi.org/10.1109/TrustCom/BigDataSE.2018.00186 [Google Scholar] [CrossRef]
17. N. El Madhoun, J. Hatin and E. Bertin, “Going beyond the blockchain hype: In which cases are blockchains useful for it applications?” in 2019 3rd Cyber Security in Networking Conf. (CSNet), pp. 21–27, 2019. [Google Scholar]
18. A. A. Simaremare, I. A. Aditya, F. N. Haryadi and H. Indrawan, “Suitability study of blockchain application in electric utility company business processes,” IOP Conference Series: Materials Science and Engineering, vol. 1098, no. 5, pp. 052105, 2021. https://doi.org/10.1088/1757-899x/1098/5/052105 [Google Scholar] [CrossRef]
19. T. Koens and E. Poll, “What blockchain alternative do you need?” in Data Privacy Management, Cryptocurrencies and Blockchain Technology, Springer International Publishing, Barcelona, Spain, pp. 113–129, 2018. https://doi.org/10.1007/978-3-030-00305-0_9 [Google Scholar] [CrossRef]
20. B. A. Scriber, “A framework for determining blockchain applicability,” IEEE Software, vol. 35, no. 4, pp. 70–77, 2018. https://doi.org/10.1109/MS.2018.2801552 [Google Scholar] [CrossRef]
21. S. Klein, W. Prinz and W. Gräther, “A use case identification framework and use case canvas for identifying and exploring relevant blockchain opportunities,” in Proc. of 1st ERCIM Blockchain Workshop 2018. European Society for Socially Embedded Technologies (EUSSET), Amsterdam, Netherlands, no. 10, pp. 1–8, 2018. https://doi.org/10.18420/blockchain2018 [Google Scholar] [CrossRef]
22. O. Labazova, “Towards a framework for evaluation of blockchain implementations,” in ICIS 2019 Proc., pp. 1, 2019. [Online]. Available: https://aisel.aisnet.org/icis2019/blockchain_fintech/blockchain_fintech/18/ [Google Scholar]
23. C. Lapointe and L. Fishbane, “The blockchain ethical design framework,” Innovations: Technology, Governance, Globalization, Glob, vol. 12, no. 3–4, pp. 50–71, 2019. https://doi.org/10.1162/inov_a_00275 [Google Scholar] [CrossRef]
24. S. Wibowo and E. P. Hw, “Blockchain implementation assessment framework, case study of IoT LPWA licensing in Indonesia,” in 2018 Int. Conf. on ICT for Smart Society (ICISS), West Java, Indonesia, pp. 1–5, 2018. https://doi.org/10.1109/ICTSS.2018.8549940 [Google Scholar] [CrossRef]
25. M. Çolak, I. Kaya, B. Özkan, A. Budak and A. Karaşan, “A Multi-criteria evaluation model based on hesitant fuzzy sets for blockchain technology in supply chain management,” Journal of Intelligent & Fuzzy Systems, vol. 38, no. 1, pp. 935–946, 2020. https://doi.org/10.3233/JIFS-179460 [Google Scholar] [CrossRef]
26. G. Büyüközkan and G. Tüfekçi, “A Decision-making framework for evaluating appropriate business blockchain platforms using multiple preference formats and VIKOR,” Journal of Intelligent & Fuzzy Systems, vol. 571, pp. 337–357, 2021. https://doi.org/10.1016/j.ins.2021.04.044 [Google Scholar] [CrossRef]
27. A. Vlachokostas, M. Poplawski and S. N. Gupta Gourisetti, “Is blockchain a suitable technology for ensuring the integrity of data shared by lighting and other building systems,” in 2020 Resilience Week, RWS 2020, Salt Lake City, UT, USA pp. 147–152, 2020. https://doi.org/10.1109/RWS50334.2020.9241309 [Google Scholar] [CrossRef]
28. I. Erol, I. M. Ar, A. I. Ozdemir, I. Peker, A. Asgary et al., “Assessing the feasibility of blockchain technology in industries: Evidence from Turkey,” Journal of Enterprise Information Management, vol. 34, no. 3, pp. 746–769, 2020. https://doi.org/10.1108/JEIM-09-2019-0309 [Google Scholar] [CrossRef]
29. N. Gupta Gourisetti, M. Mylrea and H. Patangia, “Application of rank-weight methods to blockchain cybersecurity vulnerability assessment framework,” in 2019 IEEE 9th Annual Computing and Communication Workshop and Conf. (CCWC), pp. 206–213, 2019. https://doi.org/10.1109/CCWC.2019.8666518 [Google Scholar] [CrossRef]
30. S. Flovik, R. A. R. Moudnib and P. Vassilakopoulou, “Determinants of blockchain technology introduction in organizations: An empirical study among experienced practitioners,” Procedia Computer Science, vol. 181, pp. 664–670, 2021. https://doi.org/10.1016/j.procs.2021.01.216 [Google Scholar] [CrossRef]
31. W. Yang, S. Garg, Z. Huang and B. Kang, “A decision model for blockchain applicability into knowledge-based conversation system,” Knowledge-Based Systems, vol. 220, pp. 106791, 2021. https://doi.org/10.1016/j.knosys.2021.106791 [Google Scholar] [CrossRef]
32. I. M. Ar, I. Erol, I. Peker, A. I. Ozdemir, T. D. Medeni et al., “Evaluating the feasibility of blockchain in logistics operations: A decision framework,” Expert Systems with Applications, vol. 158, pp. 113543, 2020. https://doi.org/10.1016/j.eswa.2020.113543 [Google Scholar] [CrossRef]
33. A. Maden, “Suitability evaluation of blockchain–based systems using fuzzy ANP-A case study in a logistics company,” Advances in Intelligent Systems and Computing, vol. 1029, pp. 401–407, 2020. https://doi.org/10.1007/978-3-030-23756-1_50 [Google Scholar] [CrossRef]
34. A. Maden and E. Alptekin, “A evaluation of factors affecting the decision to adopt blockchain technology: A logistics company case study using fuzzy DEMATEL,” Journal of Intelligent & Fuzzy Systems, vol. 39, pp. 6279–6291, 2020. https://doi.org/10.3233/JIFS-189096 [Google Scholar] [CrossRef]
35. H. Falwadiya and S. Dhingra, “Blockchain technology adoption in government organizations: A systematic literature review,” Journal of Global Operations and Strategic Sourcing, vol. 15, no. 3, Emerald Group Holdings Ltd., pp. 473–501, 2022. https://doi.org/10.1108/JGOSS-09-2021-0079 [Google Scholar] [CrossRef]
36. D. Ghode, V. Yadav, R. Jain and G. Soni, “Adoption of blockchain in supply chain: An analysis of influencing factors,” Journal of Enterprise Information Management, vol. 33, no. 3, pp. 437–456, 2020. https://doi.org/10.1108/JEIM-07-2019-0186 [Google Scholar] [CrossRef]
37. S. S. Kamble, A. Gunasekaran, V. Kumar, A. Belhadi and C. Foropon, “A machine learning based approach for predicting blockchain adoption in supply chain,” Technological Forecasting and Social Change, vol. 163, pp. 120465, 2021. https://doi.org/10.1016/j.techfore.2020.120465 [Google Scholar] [CrossRef]
38. A. K. Kar and L. Navin, “Diffusion of blockchain in insurance industry: An analysis through the review of academic and trade literature,” Telematics and Informatics, vol. 58, Elsevier Ltd., pp. 101532, 2021. https://doi.org/10.1016/j.tele.2020.101532 [Google Scholar] [CrossRef]
39. V. Hassija, S. Zeadally, I. Jain, A. Tahiliani, V. Chamola et al., “Framework for determining the suitability of blockchain: Criteria and issues to consider,” Transactions on Emerging Telecommunications Technologies, vol. 32, no. 10, 2021. https://doi.org/10.1002/ett.4334 [Google Scholar] [CrossRef]
40. B. A. Scriber, “A framework for determining blockchain applicability,” IEEE Software, vol. 35, no. 4, pp. 70–77, 2018. https://doi.org/10.1109/MS.2018.2801552 [Google Scholar] [CrossRef]
41. S. N. G. Gourisetti, M. Mylrea and H. Patangia, “Evaluation and demonstration of blockchain applicability framework,” IEEE Transactions on Engineering Management, vol. 67, no. 4, pp. 1142–1156, 2020. https://doi.org/10.1109/TEM.2019.2928280 [Google Scholar] [CrossRef]
42. T. Clohessy, T. Acton and N. Rogers, “Blockchain adoption: Technological, organisational and environmental considerations,” Business Transformation Through Blockchain, vol. 1, pp. 47–76, 2019. [Google Scholar]
43. S. Alzahrani, T. Daim and K. K. R. Choo, “Assessment of the blockchain technology adoption for the management of the electronic health record systems,” IEEE Transactions on Engineering Management, 2022. https://doi.org/10.1109/TEM.2022.3158185 [Google Scholar] [CrossRef]
44. B. Özkan, İ Kaya, M. Erdoğan and A. Karaşan, “Evaluating blockchain risks by using a MCDM methodology based on pythagorean fuzzy sets,” Advances in Intelligent Systems and Computing, vol. 1029, pp. 935–943, 2020. https://doi.org/10.1007/978-3-030-23756-1_111 [Google Scholar] [CrossRef]
45. K. A. Dawood, K. Y. Sharif, A. A. Ghani, H. Zulzalil, A. A. Zaidan et al., “Towards a unified criteria model for usability evaluation in the context of open source software based on a fuzzy Delphi method,” Information and Software Technology, vol. 130, pp. 106453, 2021. https://doi.org/10.1016/j.infsof.2020.106453 [Google Scholar] [CrossRef]
46. L. Jardim, S. Pranto, P. Ruivo and T. Oliveira, “What are the main drivers of blockchain adoption within supply chain?–an exploratory research,” Procedia Computer Science, vol. 181, pp. 495–502, 2021. https://doi.org/10.1016/j.procs.2021.01.195 [Google Scholar] [CrossRef]
47. L. M. De Rossi, N. Abbatemarco and G. Salviotti, “Towards a comprehensive blockchain architecture continuum.,” in Proc. of the 52nd Hawaii Int. Conf. on System Sciences, vol. 6, pp. 4605–4614, 2019. https://doi.org/10.24251/hicss.2019.557 [Google Scholar] [CrossRef]
48. M. Lustenberger, S. Malešević and F. Spychiger, “Ecosystem readiness: Blockchain adoption is driven externally,” Frontiers in Blockchain, pp. 33, 2021. https://doi.org/10.3389/FBLOC.2021.720454 [Google Scholar] [CrossRef]
49. B. W. Barnes III and B. Xiao, “Organizational adoption of blockchain technology: An ecosystem perspective,” in DIGIT 2019 Proceeding, vol. 9, 2019. [Google Scholar]
50. V. Chittipaka, S. Kumar, U. Sivarajah, J. L. H. Bowden and M. M. Baral, “Blockchain technology for supply chains operating in emerging markets: An empirical examination of technology-organization-environment (TOE) framework,” Annals of Operations Research, pp. 1–28, 2022. https://doi.org/10.1007/s10479-022-04801-5 [Google Scholar] [CrossRef]
51. S. Malik, M. Chadhar, S. Vatanasakdakul and M. Chetty, “Factors affecting the organizational adoption of blockchain technology: Extending the technology–organization–environment (TOE) framework in the Australian context,” Sustainability, vol. 13, no. 16, pp. 9404, 2021. https://doi.org/10.3390/su13169404 [Google Scholar] [CrossRef]
52. T. J. Murray, L. L. Pipino and J. P. Van Gigch, “A pilot study of fuzzy set modification of Delphi,” Human Systems Management, vol. 5, no. 1, pp. 76–80, 1985. https://doi.org/10.3233/HSM-1985-5111 [Google Scholar] [CrossRef]
53. K. F. H. Egfjord and K. J. Sund, “A modified Delphi method to elicit and compare perceptions of industry trends,” MethodsX, vol. 7, pp. 101081, 2020. https://doi.org/10.1016/j.mex.2020.101081 [Google Scholar] [PubMed] [CrossRef]
54. X. Ouyang and F. Guo, “Intuitionistic fuzzy analytical hierarchical processes for selecting the paradigms of mangroves in municipal wastewater treatment,” Chemosphere, vol. 197, pp. 634–642, 2018. https://doi.org/10.1016/j.chemosphere.2017.12.102 [Google Scholar] [PubMed] [CrossRef]
55. S. R. Mohandes, H. Sadeghi, A. Fazeli, A. Mahdiyar, M. R. Hosseini et al., “Causal analysis of accidents on construction sites: A hybrid fuzzy Delphi and DEMATEL approach,” Safety Science, vol. 151, pp. 105730, 2022. https://doi.org/10.1016/j.ssci.2022.105730 [Google Scholar] [CrossRef]
56. A. Tuni, W. L. Ijomah, F. Gutteridge, M. Mirpourian, S. Pfeifer et al., “Risk assessment for circular business models: A fuzzy Delphi study application for composite materials,” Journal of Cleaner Production, vol. 389, pp. 135722, 2023. https://doi.org/10.1016/j.jclepro.2022.135722 [Google Scholar] [CrossRef]
57. A. Garai, T. K. Roy, S. Mahavidyalaya and W. Bengal, “Weighted intuitionistic fuzzy Delphi method,” Notes on Intuitionistic Fuzzy Sets, vol. 4, no. 7, pp. 38–42, 2013. [Google Scholar]
58. A. Padilla-Rivera, B. B. T. do Carmo, G. Arcese and N. Merveille, “Social circular economy indicators: Selection through fuzzy Delphi method,” Sustainable Production and Consumption, vol. 26, pp. 101–110, 2021. https://doi.org/10.1016/j.spc.2020.09.015 [Google Scholar] [CrossRef]
59. G. Paré, A. F. Cameron, P. Poba-Nzaou and M. Templier, “A systematic assessment of rigor in information systems ranking-type delphi studies,” Information & Management, vol. 50, no. 5, pp. 207–217, 2013. https://doi.org/10.1016/j.im.2013.03.003 [Google Scholar] [CrossRef]
60. P. A. Ejegwa and I. C. Onyeke, “Intuitionistic fuzzy statistical correlation algorithm with applications to multicriteria-based decision-making processes,” International Journal of Intelligent Systems, vol. 36, no. 3, pp. 1386–1407, 2021. https://doi.org/10.1002/int.22347 [Google Scholar] [CrossRef]
61. A. Ishikawa, M. Amagasa, T. Shiga, G. Tomizawa, R. Tatsuta et al., “The max-min Delphi method and fuzzy Delphi method via fuzzy integration,” Fuzzy Sets and Systems, vol. 55, no. 3, pp. 241–253, 1993. https://doi.org/10.1016/0165-0114(93)90251-C [Google Scholar] [CrossRef]
62. L. A. Zadeh, “The concept of a linguistic variable and its application to approximate reasoning-III,” Information Sciences, vol. 9, no. 1, pp. 43–80, 1975. https://doi.org/10.1016/0020-0255(75)90017-1 [Google Scholar] [CrossRef]
63. K. T. Atanassov, “Intuitionistic fuzzy sets,” Fuzzy Sets and Systems, vol. 20, no. 1, pp. 87–96, 1986. https://doi.org/10.1016/S0165-0114(86)80034-3 [Google Scholar] [CrossRef]
64. C. Huang, M. Lin and Z. Xu, “Pythagorean fuzzy MULTIMOORA method based on distance measure and score function: Its application in multicriteria decision making process,” Knowledge and Information Systems, vol. 62, no. 11, pp. 4373–4406, 2020. https://doi.org/10.1007/s10115-020-01491-y [Google Scholar] [CrossRef]
65. P. A. Ejegwa and J. M. Agbetayo, “Similarity-distance decision-making technique and its applications via intuitionistic fuzzy pairs,” Journal of Computational and Cognitive Engineering, vol. 2, no. 1, pp. 68–74, 2023. https://doi.org/10.47852/BONVIEWJCCE512522514 [Google Scholar] [CrossRef]
66. M. Montajabiha, “An extended PROMETHE II multi-criteria group decision making technique based on intuitionistic fuzzy logic for sustainable energy planning,” Group Decision and Negotiation, vol. 25, no. 2, pp. 221–244, 2016. https://doi.org/10.1007/s10726-015-9440-z [Google Scholar] [CrossRef]
67. J. Roy, A. Ranjan, A. Debnath and S. Kar, “An extended MABAC for multi-attribute decision making using trapezoidal interval type-2 fuzzy numbers,” arXiv e-Prints, 2016. https://doi.org/10.48550/arXiv.1607.01254 [Google Scholar] [CrossRef]
68. S. Liu, J. Zhang, B. Niu, L. Liu and X. He, “A novel hybrid multi-criteria group decision-making approach with intuitionistic fuzzy sets to design reverse supply chains for COVID-19 medical waste recycling channels,” Computers & Industrial Engineering, vol. 169, pp. 108228, 2022. https://doi.org/10.1016/j.cie.2022.108228 [Google Scholar] [PubMed] [CrossRef]
69. K. Kumar and S. M. Chen, “Multiple attribute group decision making based on advanced linguistic intuitionistic fuzzy weighted averaging aggregation operator of linguistic intuitionistic fuzzy numbers,” Information Sciences, vol. 587, pp. 813–824, 2022. https://doi.org/10.1016/j.ins.2021.11.014 [Google Scholar] [CrossRef]
70. K. Guo and Q. Song, “On the entropy for atanassov’s intuitionistic fuzzy sets: An interpretation from the perspective of amount of knowledge,” Applied Soft Computing, vol. 24, pp. 328–340, 2014. https://doi.org/10.1016/J.ASOC.2014.07.006 [Google Scholar] [CrossRef]
71. S. Çalı and Ş. Y. Balaman, “A novel outranking based multi criteria group decision making methodology integrating ELECTRE and VIKOR under intuitionistic fuzzy environment,” Expert Systems with Applications, vol. 119, pp. 36–50, 2019. https://doi.org/10.1016/j.eswa.2018.10.039 [Google Scholar] [CrossRef]
72. R. Almahasneh, P. Foldesi and L. T. Koczy, “Fuzzy set based models comparative study for the td tsp with rush hours and traffic regions,” Communications in Computer and Information Science, vol. 1238 CCIS, pp. 699–714, 2020. https://doi.org/10.1007/978-3-030-50143-3_55 [Google Scholar] [CrossRef]
73. N. Tsoniotis, D. Kourtesis, S. Tsiakmakis, V. Christaras, M. Makridis et al., “Feasibility study and prototyping of a blockchain-based transport service pricing and allocation platform,” Technical Report. EUR 29738 EN., 2019. https://doi.org/10.2760/60436 [Google Scholar] [CrossRef]
74. W. Li, S. Zhai, W. Xu, W. Pedrycz, Y. Qian et al., “Feature selection approach based on improved fuzzy C-means with principle of refined justifiable granularity,” IEEE Transactions on Fuzzy Systems, pp. 1–15, 2022. https://doi.org/10.1109/TFUZZ.2022.3217377 [Google Scholar] [CrossRef]
Cite This Article
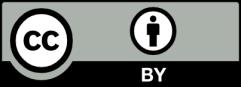