Open Access
ARTICLE
MSF-Net: A Multilevel Spatiotemporal Feature Fusion Network Combines Attention for Action Recognition
1 School of Electronic and Information Engineering, Nanjing University of Information Science and Technology, Nanjing, 210044, China
2 Jiangsu Key Laboratory of Meteorological Observation and Information Processing, Nanjing, 210044, China
* Corresponding Author: Chuang Zhang. Email:
Computer Systems Science and Engineering 2023, 47(2), 1433-1449. https://doi.org/10.32604/csse.2023.040132
Received 06 March 2023; Accepted 17 April 2023; Issue published 28 July 2023
Abstract
An action recognition network that combines multi-level spatiotemporal feature fusion with an attention mechanism is proposed as a solution to the issues of single spatiotemporal feature scale extraction, information redundancy, and insufficient extraction of frequency domain information in channels in 3D convolutional neural networks. Firstly, based on 3D CNN, this paper designs a new multilevel spatiotemporal feature fusion (MSF) structure, which is embedded in the network model, mainly through multilevel spatiotemporal feature separation, splicing and fusion, to achieve the fusion of spatial perceptual fields and short-medium-long time series information at different scales with reduced network parameters; In the second step, a multi-frequency channel and spatiotemporal attention module (FSAM) is introduced to assign different frequency features and spatiotemporal features in the channels are assigned corresponding weights to reduce the information redundancy of the feature maps. Finally, we embed the proposed method into the R3D model, which replaced the 2D convolutional filters in the 2D Resnet with 3D convolutional filters and conduct extensive experimental validation on the small and medium-sized dataset UCF101 and the large-sized dataset Kinetics-400. The findings revealed that our model increased the recognition accuracy on both datasets. Results on the UCF101 dataset, in particular, demonstrate that our model outperforms R3D in terms of a maximum recognition accuracy improvement of 7.2% while using 34.2% fewer parameters. The MSF and FSAM are migrated to another traditional 3D action recognition model named C3D for application testing. The test results based on UCF101 show that the recognition accuracy is improved by 8.9%, proving the strong generalization ability and universality of the method in this paper.Keywords
Cite This Article
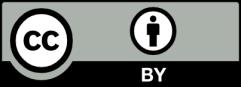