Open Access
ARTICLE
Modified Metaheuristics with Weighted Majority Voting Ensemble Deep Learning Model for Intrusion Detection System
1 Information Technology Department, Faculty of Computing and Information Technology, King Abdulaziz University, Jeddah, 21589, Saudi Arabia
2 Center of Excellence in Smart Environment Research, King Abdulaziz University, Jeddah, 21589, Saudi Arabia
3 Department of Computer Science, Faculty of Computing and Information Technology, King Abdulaziz University, Jeddah, 21589, Saudi Arabia
4 Information Systems Department, Faculty of Computing and Information Technology, King Abdulaziz University, Jeddah, 21589, Saudi Arabia
* Corresponding Author: Mahmoud Ragab. Email:
Computer Systems Science and Engineering 2023, 47(2), 2497-2512. https://doi.org/10.32604/csse.2023.041446
Received 23 April 2023; Accepted 12 June 2023; Issue published 28 July 2023
Abstract
The Internet of Things (IoT) system has confronted dramatic growth in high dimensionality and data traffic. The system named intrusion detection systems (IDS) is broadly utilized for the enhancement of security posture in an IT infrastructure. An IDS is a practical and suitable method for assuring network security and identifying attacks by protecting it from intrusive hackers. Nowadays, machine learning (ML)-related techniques were used for detecting intrusion in IoTs IDSs. But, the IoT IDS mechanism faces significant challenges because of physical and functional diversity. Such IoT features use every attribute and feature for IDS self-protection unrealistic and difficult. This study develops a Modified Metaheuristics with Weighted Majority Voting Ensemble Deep Learning (MM-WMVEDL) model for IDS. The proposed MM-WMVEDL technique aims to discriminate distinct kinds of attacks in the IoT environment. To attain this, the presented MM-WMVEDL technique implements min-max normalization to scale the input dataset. For feature selection purposes, the MM-WMVEDL technique exploits the Harris hawk optimization-based elite fractional derivative mutation (HHO-EFDM) technique. In the presented MM-WMVEDL technique, a Bi-directional long short-term memory (BiLSTM), extreme learning machine (ELM) and an ensemble of gated recurrent unit (GRU) models take place. A wide range of simulation analyses was performed on CICIDS-2017 dataset to exhibit the promising performance of the MM-WMVEDL technique. The comparison study pointed out the supremacy of the MM-WMVEDL method over other recent methods with accuracy of 99.67%.Keywords
Cite This Article
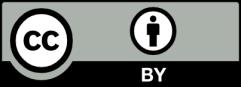