Open Access
ARTICLE
Letter Recognition Reinvented: A Dual Approach with MLP Neural Network and Anomaly Detection
Faculty of Computing and Information Technology in Rabigh, King Abdulaziz University, Jeddah, 21589, Saudi Arabia
* Corresponding Author: Nesreen M. Alharbi. Email:
Computer Systems Science and Engineering 2024, 48(1), 175-198. https://doi.org/10.32604/csse.2023.041044
Received 09 April 2023; Accepted 30 June 2023; Issue published 26 January 2024
Abstract
Recent years have witnessed significant advancements in the field of character recognition, thanks to the revolutionary introduction of machine learning techniques. Among various types of character recognition, offline Handwritten Character Recognition (HCR) is comparatively more challenging as it lacks temporal information, such as stroke count and direction, ink pressure, and unexpected handwriting variability. These issues contribute to a poor level of precision, which calls for the adoption of anomaly detection techniques to enhance Optical Character Recognition (OCR) schemes. Previous studies have not researched unsupervised anomaly detection using MLP for handwriting recognition. Therefore, this study proposes a novel approach for enhanced English letter identification based on a Multi-Layer Perceptron (MLP) neural network and an anomaly detection method. The MLP neural network is trained on a massive dataset of letters, enabling it to achieve high accuracy in recognizing letters from different font styles. Additionally, to further enhance the performance of the system, an anomaly detection method is employed to identify and eliminate any outliers or anomalies in the recognition process. The combination of these two techniques results in a highly accurate and robust letter recognition system that can be applied to a wide range of practical applications. The hybrid approach aims to increase the accuracy of English letter identification while minimizing misclassification, i.e., classification issues associated with recognizing English letters in images. To identify handwritten letters based on their underlying patterns, discriminant samples with extracted attributes were fed into a neural network, which was then used to categorize the data. This paper achieved significant improvements in English letter identification by coupling the anomaly detection algorithm and the multilayer perceptron neural network. When tested on the UCI-English letters dataset, this hybrid technique boosted the accuracy from 84.11% using the multiple neural networks to an impressive 92.84% with the proposed method. Moreover, the experimental results of the study have demonstrated that the hybrid method outperforms modern classification techniques for handwriting letter recognition. Overall, the proposed approach has tremendous potential to advance the field of character recognition, especially for offline handwritten character recognition, and can pave the way for developing more accurate and efficient OCR systems in the future.Keywords
Cite This Article
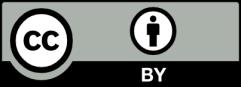