Open Access
ARTICLE
Optimal Allocation of Public Transport Hub Based on Load Loss Value and the Economy of Distribution Network
1 CHN Energy New Energy Technology Research Institute Co., Ltd., Beijing, 102211, China
2 School of Electrical and Electronic Engineering, North China Electric Power University, Beijing, 102206, China
3 CHN Energy Ningxia Yuanyanghu First Power Generation Co., Ltd., Yinchuan, 750409, China
* Corresponding Author: Yuying Zhang. Email:
Energy Engineering 2022, 119(6), 2211-2229. https://doi.org/10.32604/ee.2022.020341
Received 17 November 2021; Accepted 28 January 2022; Issue published 14 September 2022
Abstract
The rapid development of electric buses has brought a surge in the number of bus hubs and their charging and discharging capacities. Therefore, the location and construction scale of bus hubs will greatly affect the operation costs and benefits of an urban distribution network in the future. Through the scientific and reasonable planning of public transport hubs on the premise of meeting the needs of basic public transport services, it can reduce the negative impact of electric bus charging loads upon the power grids. Furthermore, it can use its flexible operation characteristics to provide flexible support for the distribution network. In this paper, taking the impact of public transport hub on the reliability of distribution network as the starting point, a three-level programming optimization model based on the value and economy of distribution network load loss is proposed. Through the upper model, several planning schemes can be generated, which provides boundary conditions for the expansion of middle-level optimization. The normal operation dispatching scheme of public transport hub obtained from the middle-level optimization results provides boundary conditions for the development of lower level optimization. Through the lower level optimization, the expected load loss of the whole distribution system including bus hub under the planning scheme given by the upper level can be obtained. The effectiveness of the model is verified by an IEEE-33 bus example.Keywords
Transportation is an important part of energy consumption. Replacing traditional fuel buses with electric buses can effectively reduce traffic exhaust emissions in cities, reduce environmental pollution and improve air quality. At the same time, the promotion of electric public transport can also reduce the transportation system’s dependence on oil and promote industrial upgrading. Therefore, making full use of the advantages of energy conservation and environmental protection of electric buses is one of the important ways to encourage China to achieve the goals of “emission peak” and “carbon neutrality.”
By the end of 2020, the number of electric buses in China exceeded 379,000 [1]. Electric buses generally need to be operated, dispatched, charged, and parked in large parking lot public transport hubs (PTH) and obtain the necessary power from the power grid through the charging pile arranged in PTH. In addition, compared with traditional private electric vehicles, electric buses have a larger battery capacity. Therefore, the charging load of such a large number of electric buses is aggregated through PTH and then connected to the power grid. Its demand is bound to have an important impact on the operation of the power grid.
The rapid development of electric buses has brought a surge in the number of PTH and their charging and discharging capacity. Therefore, the location and construction scale of PTH will greatly affect the operation cost and benefit of urban distribution networks in the future. How to make scientific and reasonable planning for PTH, reduce the negative impact of electric bus charging load on the power grid on the premise of meeting the needs of basic public transport services, and use the flexible operation characteristics of PTH to provide flexible support for the distribution network has become an important problem.
At present, some scholars have studied the planning of PTH. Some scholars consider the transportation network and distribution network simultaneously when planning and studying the transportation hub [2,3]. By integrating the two networks, considering the configuration of bus lines and charging stations, and studying the optimal planning of the rapid charging pile network of electric buses, the cost of distribution network expansion investment can be reduced [4,5]. In the operation stage, the use of the battery of the charging station or exchange station can cut the peak and fill the valley of the distribution network, reduce the charging cost and load fluctuation of the electric bus [6,7], and make full use of the battery energy storage resources of the electric bus in the transportation hub. In the research on the planning of transportation hubs, some scholars optimize the planning by combining them with photovoltaic and urban traction power supply systems [8] or considering the integrated energy system composed of distributed renewable energy and energy storage technology [9], so as to improve the benefits of planning and operation further. From the perspective of charging demand, some scholars have studied the operation lines of electric buses, the number and type of electric vehicles, and charging piles [10] by considering the uncertainty of charging load [11–13] based on the operation characteristics of electric bus [14].
Although the above research has conducted a comprehensive study on the planning of transportation hubs, there are few articles on the impact of transportation hubs on the reliability of distribution networks. Transportation hubs not only exists as a load, which may impact the safe, stable, and economically functional operation of distribution networks, but also because they can be used as a research object with virtual energy storage potential by participating in V2G (vehicle to grid).Some scholars have studied the impact of electric vehicles on the reliability of distribution networks [15]. Still, the operation characteristics of electric buses in transportation hubs are very different from ordinary private cars. The transportation hub has a fixed operation route and determined operation mode, so its impact on the distribution network is more predictable. And the battery capacity of electric buses has greater advantages than that of ordinary electric private cars.
Therefore, taking the impact of public transport hubs on the reliability and economy of distribution networks as the starting point, this paper proposes a PTH planning model for minimizing power loss in distribution network operation. Through the hierarchical coordination and optimization of the installation location, charging and discharging equipment capacity and operation strategy of the public transport hub in the distribution network (including the charging and discharging operation control strategy and departure plan under the normal and fault scenarios of the system), the flexible charging and discharging potential of the electric bus battery is fully released, and on the premise of meeting the public travel constraints, make it contribute to the improvement of power supply reliability level of distribution network, reduce the loss of power failure caused by distribution network failure, and take into account the economy of planning and operation.
2 Planning Model of Public Transport Pub
In order to realize the scientific planning and layout of the public transport hub in the future urban distribution network, provide power support for it in case of external power grid failure, so as to minimize the loss cost of load outage and the planning and operation cost of distribution network—a three-tier optimization method is proposed in this paper. The upper model aims to minimize the total cost and optimize the layout location of bus hubs, the purchased quantity of electric buses, and the layout quantity of charging piles. The middle-level model aims to minimize the power purchased by the public transport hub from the external power grid under the normal operation of the system and optimize the departure interval and bus charging plan. The lower level model aims to minimize the outage loss cost of a distribution system in case of external power grid failure, and optimize the departure interval, load reduction, departure state of electric bus, charge and discharge state and charge and discharge power of electric bus in fault operation state.
In the upper level, the genetic algorithm is used to determine the planning and layout scheme of the public transport hub, the layout position (node) of the public transport hub in the distribution network, the purchased quantity of electric public transport, and the layout quantity of charging piles are obtained. In the middle level, the daily dispatching is adopted, and the travel demand is predicted according to the historical number of passengers. By optimizing the departure interval of normal operation by linear programming method, the operation strategy of the bus hub satisfying travel demand and minimizing charging power is determined, and the load curve of the bus hub (the power interaction curve with the external power grid) is obtained when the simulation year is running normally. In the lower level, real-time dispatching is adopted. According to the planning scheme obtained by the upper level and the operation mode obtained by the middle level, the power support effect of the bus hub on the power grid in case of power grid failure is determined, and the rolling optimization method is adopted to optimize the departure interval of PTH, the departure state of electric buses, the charging and discharging state and charging and discharging power of electric bus, so as to determine the expected outage loss value of distribution network with PTH in case of fault.
In this paper, a three-level optimization model for PTH planning is proposed to describe and solve the above problems. Its basic framework is shown in Fig. 1.
Figure 1: Basic framework of the three-tier optimization model
2.1 Upper Level Programming Model
For the three-level optimization model shown in Fig. 1, the upper optimization problem is a nonlinear optimization problem. Its purpose is to determine the optimal layout position of PTH in the distribution network, the purchased quantity of electric buses in PTH, and the layout quantity of charging piles. The objective function and constraints of the model are described in detail as follows.
In this paper, the minimization of the total cost of the whole distribution network system in one year is taken as the objective function of the optimal planning model of the public transport hub in the distribution network, as shown in Eqs. (1)–(4).
The total cost includes the investment cost of the public transport hub in distribution network planning and the operation cost of the distribution network. The operation of the distribution network is divided into the normal part and the fault part. The investment cost includes the purchase cost of an electric bus in the bus hub and the purchase cost of the charging pile. The cost during normal operation comes from the power purchase cost of the distribution network and the operation and maintenance cost of electric buses and charging pile in a public transport hub. The cost during fault operation comes from the power load loss value generated during fault. Value of lost load (VOLL) refers to the loss to the national economy caused by power failure. It quantifies the social cost corresponding to the loss caused by power supply interruption.
Among them,
Due to the limitation of land area and space, the number of charging piles installed in a PTH shall not be too large, so the number of charging piles shall be less than the maximum limit:
In addition, the maximum number of buses purchased in each PTH shall not be greater than the maximum vehicle capacity limit, so there are:
where
2.2 Middle Level Normal Operation Optimization Model
In actual operation, the distribution system with PTH will face two situations: normal operation (all components or equipment of the system have no-fault and are in normal operation) and fault operation (some components or equipment in the system have to fault and cannot operate normally). Therefore, in order to effectively simulate the impact of PTH on the operation of the distribution system and its power supply reliability under the above two operation scenarios, the paper proposes a middle-level normal operation optimization model and a lower-level fault operation optimization model. The purpose of the middle-level optimization model is to simulate the normal operation mode of the system under the condition of a given system equipment configuration state. Specifically, for the normal operation state of the system, the model determines the optimal charging plan of its internal vehicles according to the calculation of transportation demand [the optimization variable is the departure interval of electric buses (
The objective function of the middle-level optimization model is to minimize the charging power in the operation cycle of the distribution system with PTH:
a) Restrictions on the number of buses on the line: The number of electric buses on the bus line is the number leaving the bus hubs before time t:
where,
b) Quantity constraints available for departure: The number of electric buses that can be issued by PTH is the number of electric buses that can start at any time minus the number of already started buses and plus car that is already charged at time t:
where,
c) Constraints on the number of arriving buses: The number of buses arriving at PTH at time t is the total number of electric buses of each line at departing time
where,
d)Constraints on the number of uncharged buses: The number of buses failing to charge at time t+1 is related to the number of buses failing to complete charging at time t and newly arrived buses:
where,
e) Bus charging quantity constraint: The relationship constraints of the number of electric buses need to be charged in transport hub i at time t+1 and the number of buses arriving at the bus hub are as follows:
f) Total bus quantity constraint: The total number of electric buses in PTH is the number of vehicles at the station plus the number of vehicles running on each bus line:
g) User satisfaction constraints: The operation of the bus needs to ensure that the passengers on the bus line can travel normally and meet the user's requirements for bus comfort. The quantity model of users on the line is as follows:
where,
Based on the above formula, the user satisfaction index is further defined, as shown in Eq. (19); in order to meet the travel satisfaction of users, the value shall not be lower than the set value:
where,
h) Power flow constraint of distribution system: When planning the public transport hub from the distribution network, it is necessary to consider the network constraints of the distribution network to ensure the safe and stable operation of the distribution network, including power flow constraints and safety constraints, as shown in Eqs. (18)–(27).
where,
Eq. (18) represents the node active power balance constraint without public transport hub; Eq. (19) represents the node active power balance constraint of the bus hub; Eq. (20) represents the node reactive power balance constraint without public transport hub; Eq. (21) represents the relationship constraint of adjacent node voltage based on ohm law; Eq. (22) represents the equality constraints of apparent power and node voltage and current; Eq. (23) indicates that Eq. (22) is subject to cone relaxation and convexity; Eqs. (24)–(25) represent the two norm forms of voltage and branch current in the defined standard form; Eq. (26) represents the constraints of line current and node voltage; Eq. (27) represents the branch network loss.
i) Power interaction between PTH and distribution network: According to the above formula, the load power of the public transport hub in normal operation can be obtained, and the public transport hub does not send back power to the power grid at this time:
where,
During normal operation, there is only charging power, so the power interaction with the power grid at time t is shown in Eq. (29):
where,
j) Power purchased to distribution network from the main network:
where,
2.3 Lower-Level Fault Operation Optimization Model
The purpose of the lower-level optimization model is to simulate the system operation mode under the condition of a given system equipment configuration state to determine the outage loss cost (power supply reliability level) caused by system outage. For the system fault state, the model calculates the optimal charge/discharge plan corresponding to each electric bus in PTH according to the distribution network fault and its impact; the optimization variables include the departure interval (
The VOLL data are transferred to the upper-level model to display the prior of reliability of the current PTH planning scheme provided by the upper-model. If the VOLL is too large, it indicates that the system reliability performance under the current scheme is poor and will be excluded in the next iteration. Through the above process, the PTH planning scheme with the best reliability performance will be found as the optimal solution.
The objective function of the lower-level optimization model is to minimize the value of power loss during the operation cycle of the distribution system with PTH, as shown in Eq. (32):
where,
2.3.2 Constraints of Fault Operation
When the user’s power supply is interrupted due to the failure of the distribution network, the system operator can dispatch the PTH electric bus in the system to supply power (provide emergency power support for the distribution network as the power supply), and jointly optimize the charge and discharge power of the two-way charging pile in the station and the departure plan, on the basis of meeting the constraints of bus service, minimize the load shortage and load loss cost of the system.
For fault operation, the corresponding constraints of the system are as follows, and the constraints of user satisfaction are the same as those of normal operation:
a) Departure quantity constraint: The number of electric buses on the bus line is the number of bus departures from bus hubs before time t:
where,
where,
b) Electric bus battery state constraints: In the fault operation state, the available energy in each electric bus battery is equal to the power at the previous time plus the charging power in this period minus the discharging power participating in V2G, as shown in Eq. (37):
where,
c) Battery capacity constraint: The battery capacity of each electric bus shall meet the following constraints:
where,
d) Interactive constraint between PTH and distribution network power: The charge and discharge power constraints of each electric bus are shown in Eqs. (39)–(41). Wherein, Eqs. (39)–(40) respectively indicate that the charging and discharging power of the electric bus needs to be less than the rated power of the charging pile; Eq. (41) indicates that the battery charging and discharging processes of each vehicle cannot be carried out at the same time, and the electric bus cannot participate in power interaction through the charging pile when driving on the road:
where,
e) Quantity restriction of charge/discharge electric bus: At the same time, the number of electric buses interacting with the power grid at the same node shall be less than the number of charging piles:
f) Power flow constraint of distribution system: When plans the public transport hub, it is necessary to consider the network constraints of the distribution network to ensure the safe and stable operation of the distribution network, including power flow constraints and safety constraints, as shown in Eqs. (20)–(26) and (43) and (44).
Eq. (43) represents the node active power balance constraint without public transport hub; Eq. (44) represents the node active power balance constraint with the bus hub.
In this paper, the planning of the public transport hub is divided into two levels: configuration decision-making and operation simulation. At the operation level, it is divided into two levels: normal operation and fault operation. This paper proposes a three-level optimization method, which can make the model level clear and easy to adopt different algorithms for the upper, middle and lower models, so as to solve the planning problem of public transport hubs to minimize the cost of outage loss.
The overall solution flow of the model is shown in Fig. 2.
Figure 2: Solution flow of multi-level optimization problem for PTH programming
The solution process of this model is as follows.
Based on meeting the conditions of system investment cost (economic budget), the upper model takes the minimization of load loss cost as the optimization objective to generate a PTH planning scheme that meets the investment budget constraints, including the layout location of PTH in the distribution network, the purchased quantity of buses, and the purchased quantity of charging piles. The obtained planning scheme will be used as the initial condition of the middle-level model (the optimized boundary condition).
Based on the PTH planning scheme determined by the upper model, the middle model determines the operation state of the distribution system with PTH under normal operation by means of optimization and calculates the interactive power (load curve) between PTH and the distribution network. On the one hand, the above calculation results serve as the basis for judging whether the planning scheme is reasonable or not; on the other hand, they provide initial conditions for the optimization calculation of the lower model. For simplicity, this paper believes that the fault will not occur again in a short time after fault recovery, so the bus hub has sufficient time to supplement the power released due to its previous participation in V2G until the bus hub returns to normal operation.
Based on the configuration scheme determined by the upper model and the initial operational state of the public transport hub in each period determined by the middle model, the lower model determines the power support effect of PTH on the distribution network in case of fault by means of optimization and calculates the load loss cost of the system in case of fault. On the one hand, the above calculation results will provide a basis for the determination of the objective function value of the optimization model (the effectiveness of the PTH planning scheme); on the other hand, it will also provide an optimization direction for the iterative improvement of the upper model planning scheme.
The detailed steps of the model are described as follows:
Step 1) Input the technical and economic parameters of the electric bus and charging pile in PTH integrated distribution system.
Step 2) Based on binary coding and integer coding, 2R initial planning schemes (i.e., chromosomes) are randomly generated.
Step 3) In normal operation, PTH adopts the charging mode of car-to-car charging, and optimizes the departure interval with the goal of minimizing the power purchase cost of the distribution network.
Step 4) Determine the operation mode of the system at the time t in the normal operation of the system, including the number of bus departures, departure interval, battery SoC of electric buses, and charging power of PTH. Make
Step 5) According to Step 3)–Step 4), the operation state of PTH in the simulation year M and the load power in normal operation are obtained;
Step 6) The sequential Monte Carlo simulation (SMCS) method is used to randomly simulate the state sequence (state duration) of each component the system.
Step 7) According to the time sequence state of the line in the m-th simulation year generated in step 6), judging whether there is a line fault at time t, if not,
Step 8) Use the rolling optimization method to optimize PTH operation strategy for the lower-level model, and the specific steps are as follows:
8-1) Determining the position of the fault node according to the fault/normal state of the line through the line state set generated in Step 6);
8-2) The shortest path method is used to reconstruct the network, and the tie line nearest to the fault node is closed.
8-3) In the fault operation state, with the aim of minimizing the value of power loss, the departure interval of PTH, the charging, discharging and departure state of electric buses, and the charging/discharging power of electric buses are optimized by rolling optimization method.
8-4) Calculate the VOLL of fault time t.
8-5) Update the system state, including SoC of power batteries and number of bus departures.
8-6) Judge whether
Step 9) Calculate the VOLL of the system. If
Step 10) Calculate the adaptive value of each chromosome in the current population, and keep the outstanding individuals in the parent generation through elite selection strategy without crossover and mutation operation.
Step 11) Carry out genetic, crossover and mutation operations on the remaining individuals.
Step 12) Determine whether the calculation result meets the convergence condition. If the algorithm has converged, output the current optimal solution as the final planning scheme; otherwise, return and repeat Step 3)–Step 11).
Taking IEEE-33 standard distribution network system [16] as an example, this network has 32 branches, 5 tie switch branches, 1 power network head-end reference voltage of 12.66 kv, and the three power reference values are 10 MVA.The grid structure is shown in Fig. 3, in which the dotted line is the tie line. In this paper, the load of each node is 1.8 times the original load. The load grade of each node is shown in Table 1; electric bus data are shown in Table 2, and bus line data is from reference [17]. The fault rate of the line is 0.05 times/(year * km), and the fault repair time is 4 h. The charging and discharging power of the charging pile is 40 kW.The annual investment/(pile) is 12,637.8 CNY. The passenger user satisfaction curve is shown in Fig. 4 [18].
Figure 3: IEEE-33 bus power distribution system diagram
Figure 4: Passenger satisfaction rate
Among the five nodes to be selected, the optimal configuration scheme can be obtained by optimizing the number of electric buses and charging piles. The specific results are shown in Table 3.
It can be seen from the above table that the optimal scheme of different nodes has the same number of electric buses. This is because the investment and operation cost of electric bus is large. Vehicle scheduling schemes of three routes in a typical day is illustrated in Fig. 5. After meeting the requirements for the number of electric buses during normal operation, further increasing the number of electric buses cannot reduce the total cost. When the public transport hub in the distribution network does not participate in V2G, the load loss value of the distribution network is 430,700 CNY.
Figure 5: Vehicle scheduling schemes of three routes on a typical day
The minimum cost of VOLL is 280,400 CNY, which reduces the loss by 34.9%, and the total cost of its configuration scheme is the lowest, which is 32.964 million CNY. Therefore, this scheme is the optimal scheme.
It can be seen from the above table that different nodes’ installation places and the number of charging piles will affect VOLL and cost. Figs. 5 and 6 show the impact.
Figure 6: Distribution network load loss value (ten thousand CNY)
As shown in Fig. 6, it can be seen from the plane where the green point is located that at different installation nodes, the VOLL will decrease monotonically with the increase of the number of charging piles because the increase of the number of charging piles will increase the amount of V2G at fault time, so as to reduce the value loss caused by line fault. However, with the increase in the number of charging piles, the falling speed of VOLL slows down. This is because, with the increase in the number of charging piles, all charging piles can be fully utilized only in one situation where there are serious faults and fewer passengers. In this case, the investment and operation cost of increasing the number of charging piles cannot be compensated by reducing the VOLL. As shown in Fig. 7, the total cost of distribution network operation will first decrease and then increase with the change of charging quantity, and all nodes have the same trend. Therefore, the goal of reducing the total operation cost cannot be achieved by reducing VOLL in a single aspect. The planning optimization method proposed in this paper can reduce the total operation cost of the distribution network while reducing the value of load loss.
Figure 7: Total cost of the distribution network (ten thousand CNY)
Moreover, the research on the influence of EB battery capacity was added to our manuscript. To achieve this, the capacity of power batteries
It can be seen in Table 4 that with the increase of
Moreover, we compare two different solving algorithms, namely, the global solving method (Method-I) and our proposed method (Method-II), particularly focusing on the differences in solving time and solution quality between the two methods. The performances of the two solving methods are listed in Table 5.
As the PTH optimal allocation model is a typical MILP problem, its exact solution can be directly solved by the commercial solver (such as Gurobi, Cplex, etc.), as shown in Table 5. The optimal solution obtained by Method-II is very close to that obtained by Method-I, which proves that our proposed solution method has high solution quality. However, compared with Method-I, the solving time of Method-II are shortened by 59.9%, which proves that our method has higher solving efficiency.
This paper proposes a PTH planning model for minimizing power loss in distribution network operation. The model is developed based on a hierarchical coordination framework. Firstly, we establish an optimal PTH allocation model (upper-level) to obtain the PTH planning schemes. Then, both the normal operation optimization model (middle-level) and fault optimization model (lower-level) is proposed, which could simulate the optimal operation of the PTH-integrated distribution network (PTH-DN) in both normal and contingency states. Through this design, the operation cost and load loss value of PTH-DN can be obtained and returned to the upper level to form a holistic evaluation of the current PTH planning scheme. The above processes are integrated into an iterative solution framework, which can obtain the PTH configuration plan with optimal coordination of economy and reliability. The results of the numerical study show that:
(a) Our proposed hierarchical model for optimal PTH configuration can reduce the investment cost and improve the economic and reliability of PTH-DN efficiently.
(b) Different nodes’ installation places and the number of charging piles will significantly affect VOLL and the operational cost of the system.
(c) With the increase of power battery capacity, the operating cost and VOLL decrease. However, when power battery capacity is in a high level, the decreasing trend of operating cost and VOLL is not obvious. This shows that it is unwise to blindly improve power battery capacity to gain reliability and economic benefits.
Funding Statement: The authors received no specific funding for this study.
Conflicts of Interest: The authors declare that they have no conflicts of interest to report regarding the present study.
References
1. Statistical Communiqué on the Development of the Transportation Industry (2020). https://xxgk.mot.gov.cn/2020/jigou/zhghs/202105/t20210517_3593412.html. [Google Scholar]
2. Wu, W., Lin, Y., Liu, R., Jin, W. (2022). The multi-depot electric vehicle scheduling problem with power grid characteristics. Transportation Research Part B: Methodological, 155(2), 322–347. DOI 10.1016/j.trb.2021.11.007. [Google Scholar] [CrossRef]
3. Wu, W., Lin, Y., Liu, R., Li, Y., Zhang, Y. et al. (2020). Online EV charge scheduling based on time-of-use pricing and peak load minimization: Properties and efficient algorithms. IEEE Transactions on Intelligent Transportation Systems, 23, 572–586. [Google Scholar]
4. Tao, J. (2019). Study on the optimal planning of electric bus charging network. North China Electric Power University (Beijing). [Google Scholar]
5. Lin, Y., Zhang, K., Shen, Z. J. M., Ye, B., Miao, L. (2019). Multistage large-scale charging station planning for electric buses considering transportation network and power grid. Transportation Research Part C: Emerging Technologies, 107, 423–443. DOI 10.1016/j.trc.2019.08.009. [Google Scholar] [CrossRef]
6. Zhang, W. (2013). Optimization design and economical operation of pure electric vehicles swapping station. Beijing Jiaotong University. [Google Scholar]
7. Miao, M., Lei, X., He, J. P., Li, F. (2015). The research of electric buses power plant charging optimization based on load shifting. Journal of Xihua University (Natural Science Edition), 34(4), 37–41. [Google Scholar]
8. Yang, H. (2019). Research on optimal operation strategy of electric bus charging and swapping station. Southwest Jiaotong University. [Google Scholar]
9. Cheng, Y., Wang, W., Ding, Z., He, Z. (2019). Electric bus fast charging station resource planning considering load aggregation and renewable integration. IET Renewable Power Generation, 13(7), 1132–1141. DOI 10.1049/iet-rpg.2018.5863. [Google Scholar] [CrossRef]
10. Topić, J., Soldo, J., Maletić, F., Škugor, B., Deur, J. (2020). Virtual simulation of electric bus fleets for city bus transport electrification planning. Energies, 13(13), 3410. DOI 10.3390/en13133410. [Google Scholar] [CrossRef]
11. An, K., Jing, W., Kim, I. (2020). Battery-swapping facility planning for electric buses with local charging systems. International Journal of Sustainable Transportation, 14(7), 489–502. DOI 10.1080/15568318.2019.1573939. [Google Scholar] [CrossRef]
12. An, K. (2020). Battery electric bus infrastructure planning under demand uncertainty. Transportation Research Part C: Emerging Technologies, 111(1), 572–587. DOI 10.1016/j.trc.2020.01.009. [Google Scholar] [CrossRef]
13. Liu, Z., Song, Z., He, Y. (2018). Planning of fast-charging stations for a battery electric bus system under energy consumption uncertainty. Transportation Research Record, 2672(8), 96–107. DOI 10.1177/0361198118772953. [Google Scholar] [CrossRef]
14. Wu, Z. (2017). Research on charging facility planning method electric bus based on driving characteristics. Smart Grid, 5(7), 660–664. [Google Scholar]
15. Mohammadi-Hosseininejad, S. M., Fereidunian, A., Lesani, H. (2018). Reliability improvement considering plug-in hybrid electric vehicles parking lots ancillary services: A stochastic multi-criteria approach. IET Generation, Transmission & Distribution, 12(4), 824–833. DOI 10.1049/iet-gtd.2017.0657. [Google Scholar] [CrossRef]
16. Kashem, M. A., Ganapathy, V., Jasmon, G. B., Buhari, M. I. (2000). A novel method for loss minimization in distribution networks. International Conference on Electric Utility Deregulation and Restructuring and Power Technologies, pp. 251–256. London, UK, IEEE. [Google Scholar]
17. Cheng, S. (2013). Research on short-turn vehicle combinational scheduling method for bus route. Huazhong University of Science and Technology. [Google Scholar]
18. Jiang, S., Wang, B., Yan, Z. (2017). Bus dispatching optimization model considering satisfaction degrees. Chinese Journal of Engineering Mathematics, 34(4), 375–382. [Google Scholar]
Cite This Article
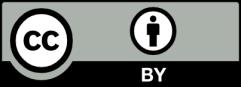