Open Access
ARTICLE
Building Graduate Salary Grading Prediction Model Based on Deep Learning
Department of Computer Science and Information Engineering, National Taipei University of Technology, Taipei, 106335, Taiwan
* Corresponding Author: Jong-Yih Kuo. Email:
Intelligent Automation & Soft Computing 2021, 27(1), 53-68. https://doi.org/10.32604/iasc.2021.014437
Received 20 September 2020; Accepted 26 October 2020; Issue published 07 January 2021
Abstract
Predicting salary trends of students after employment is vital for helping students to develop their career plans. Particularly, salary is not only considered employment information for students to pursue jobs, but also serves as an important indicator for measuring employability and competitiveness of graduates. This paper considers salary prediction as an ordinal regression problem and uses deep learning techniques to build a salary prediction model for determining the relative ordering between different salary grades. Specifically, to solve this problem, the model uses students’ personal information, grades, and family data as input features and employs a multi-output deep neural network to capture the correlation between the salary grades during training. Moreover, the model is pre-trained using a stacked denoising autoencoder and the corresponding weights after pre-training are used as the initial weights of the neural network. To improve the performance of model, dropout and bootstrap aggregation are used. The experimental results are very encouraging. With the predictive salary grades for graduates, school’s researchers can have a clear understanding of salary trends in order to promote student employability and competitiveness.Keywords
Cite This Article
Citations
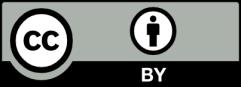