Open Access
ARTICLE
Adaptive Multi-Layer Selective Ensemble Least Square Support Vector Machines with Applications
1 State Key Laboratory of Process Automation in Mining & Metallurgy, Beijing, 102600, China
2 Faculty of Information Technology, Beijing University of Technology, Beijing, 100024, China
3 School of Computer and Software, Nanjing University of Information Science & Technology, 210044, China
4 Beijing Key Laboratory of Process Automation in Mining & Metallurgy, Beijing, 102600, China
5 BGRIMM Technology Group Co., Ltd., Beijing, 102600, China
6 Department of Computer and Information Science, The University of Mississippi, Mississippi, MS 38655, USA
* Corresponding Author: Jian Tang. Email:
Intelligent Automation & Soft Computing 2021, 29(1), 273-290. https://doi.org/10.32604/iasc.2021.016981
Received 17 January 2021; Accepted 01 March 2021; Issue published 12 May 2021
Abstract
Kernel learning based on structure risk minimum can be employed to build a soft measuring model for analyzing small samples. However, it is difficult to select learning parameters, such as kernel parameter (KP) and regularization parameter (RP). In this paper, a soft measuring method is investigated to select learning parameters, which is based on adaptive multi-layer selective ensemble (AMLSEN) and least-square support vector machine (LSSVM). First, candidate kernels and RPs with K and R numbers are preset based on prior knowledge, and candidate sub-sub-models with K*R numbers are constructed through utilizing LSSVM. Second, the candidate sub-sub-models with same KPs and different RPs are selectively fused by using the branch and bound SEN (BBSEN) to obtain K SEN-sub-models. Third, these SEN-sub-models are selectively combined through using BBSEN again to obtain SEN models with different ensemble sizes, and then a new metric index is defined to determine the final AMLSEN-LSSVM-based soft measuring model. Finally, the learning parameters and ensemble sizes of different SEN layers are obtained adaptively. Simulation results based on the UCI benchmark and practical DXN datasets are conducted to validate the effectiveness of the proposed approach.Keywords
Cite This Article
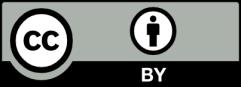