Open Access
ARTICLE
Energy-Efficiency Model for Residential Buildings Using Supervised Machine Learning Algorithm
1 School of Computer Science, National College of Business Administration and Economics, Lahore, 54000, Pakistan
2 Center for Cyber Security, Faculty of Information Science and Technology, Universiti Kebansaan Malaysia (UKM), 43600, Bangi, Selangor, Malaysia
3 School of Information Technology, Skyline University College, University City Sharjah, 1797, Sharjah, UAE
4 Department of Computer Science, Lahore Garrison University, Lahore, 54000, Pakistan
5 Canadian University Dubai, Dubai, UAE
6 Riphah School of Computing & Innovation, Faculty of Computing, Riphah International University, Lahore, 54000, Pakistan
7 Pattern Recognition and Machine Learning Lab, Department of Software Engineering, Gachon University, Seongnam, 13557, Korea
8 School of Computer Science, Minhaj University Lahore, Lahore, 54000, Pakistan
* Corresponding Author: Muhammad Adnan Khan. Email:
Intelligent Automation & Soft Computing 2021, 30(3), 881-888. https://doi.org/10.32604/iasc.2021.017920
Received 17 February 2021; Accepted 15 May 2021; Issue published 20 August 2021
Abstract
The real-time management and control of heating-system networks in residential buildings has tremendous energy-saving potential, and accurate load prediction is the basis for system monitoring. In this regard, selecting the appropriate input parameters is the key to accurate heating-load forecasting. In existing models for forecasting heating loads and selecting input parameters, with an increase in the length of the prediction cycle, the heating-load rate gradually decreases, and the influence of the outside temperature gradually increases. In view of different types of solutions for improving buildings’ energy efficiency, this study proposed a Energy-efficiency model for residential buildings based on gradient descent optimization (E2B-GDO). This model can predict a building’s heating-load conservation based on a building energy performance dataset. The input layer includes area (distribution of the glazing area, wall area, and surface area), relative density, and overall elevation. The proposed E2B-GDO model achieved an accuracy of 99.98% for training and 98.00% for validation.Keywords
Cite This Article
Citations
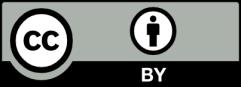