Open Access
ARTICLE
Adversarial Examples Generation Algorithm through DCGAN
1 School of Computer Science, Southwest Petroleum University, Chengdu, 610500, China
2 AECC Sichuan Gas Turbine Establishment, Mianyang, 621700, China
3 Department of Computer Science, Brunel University London, Middlesex, UB8 3PH, United Kingdom
* Corresponding Author: Desheng Zheng. Email:
Intelligent Automation & Soft Computing 2021, 30(3), 889-898. https://doi.org/10.32604/iasc.2021.019727
Received 23 April 2021; Accepted 06 July 2021; Issue published 20 August 2021
Abstract
In recent years, due to the popularization of deep learning technology, more and more attention has been paid to the security of deep neural networks. A wide variety of machine learning algorithms can attack neural networks and make its classification and judgement of target samples wrong. However, the previous attack algorithms are based on the calculation of the corresponding model to generate unique adversarial examples, and cannot extract attack features and generate corresponding samples in batches. In this paper, Generative Adversarial Networks (GAN) is used to learn the distribution of adversarial examples generated by FGSM and establish a generation model, thus generating corresponding adversarial examples in batches. The experiment shows that using the Unsupervised Representation Learning with Deep Convolutional Generative Adversarial Networks (DCGAN) to extract and learn the attack characteristics from the FGSM algorithm, the generated adversarial examples attacked the original model with a success rate of 89.1%. For the model attack with increased protection, the success rate increased by 30.3%. This suggests that the adversarial examples generated by GAN are more effective and aggressive. This paper proposes a new approach to generate adversarial examples.Keywords
Cite This Article
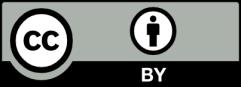