Open Access
ARTICLE
Big Data Analytics with OENN Based Clinical Decision Support System
1 Department of CSE, Acharya Nagarjuna University, Guntur, Andhra Pradesh, 522510, India
2 MIT Art Design and Technology University, Pune, 412201, India
3 Department of CSE, Gokaraju Rangaraju Institute of Engineering & Technology, Hyderabad, 500090, India
4 Department of ECE, KITS, Warangal, 506015, India
5 Department of CSE, South Point Group of Institutions, Sonepat, 131001, India
6 School of Computer Science and Engineering, Lovely Professional University, Punjab, 144411, India
7 Department of Computer Application, Shri Ramdeobaba College of Engineering and Management, Nagpur, India
* Corresponding Author: Kranthi Kumar Singamaneni. Email:
(This article belongs to the Special Issue: Soft Computing Methods for Intelligent Automation Systems)
Intelligent Automation & Soft Computing 2022, 31(2), 1241-1256. https://doi.org/10.32604/iasc.2022.020203
Received 14 May 2021; Accepted 08 July 2021; Issue published 22 September 2021
Abstract
In recent times, big data analytics using Machine Learning (ML) possesses several merits for assimilation and validation of massive quantity of complicated healthcare data. ML models are found to be scalable and flexible over conventional statistical tools, which makes them suitable for risk stratification, diagnosis, classification and survival prediction. In spite of these benefits, the utilization of ML in healthcare sector faces challenges which necessitate massive training data, data preprocessing, model training and parameter optimization based on the clinical problem. To resolve these issues, this paper presents new Big Data Analytics with Optimal Elman Neural network (BDA-OENN) for clinical decision support system. The focus of the BDA-OENN model is to design a diagnostic tool for Autism Spectral Disorder (ASD), which is a neurological illness related to communication, social skills and repetitive behaviors. The presented BDA-OENN model involves different stages of operations such as data preprocessing, synthetic data generation, classification and parameter optimization. For the generation of synthetic data, Synthetic Minority Over-sampling Technique (SMOTE) is used. Hadoop Ecosystem tool is employed to manage big data. Besides, the OENN model is used for classification process in which the optimal parameter setting of the ENN model by using Binary Grey Wolf Optimization (BGWO) algorithm. A detailed set of simulations were performed to highlight the improved performance of the BDA-OENN model. The resultant experimental values report the betterment of the BDA-OENN model over the other methods in terms of distinct performance measures. Ligent healthcare systems assists to make better decision, which further enables the patient to provide improved medical services. At the same time, skin lesion is a deadly disease that affects people of all age groups. Early, skin lesion segmentation and classification play a vital role in the precise diagnosis of skin cancer by intelligent system. But the automated diagnosis of skin lesions in dermoscopic images is a challenging process because of the problems such as artifacts (hair, gel bubble, ruler marker), blurry boundary, poor contrast and variable sizes and shapes of the lesion images. To address these problems, this study develops Intelligent Multi-Level Thresholding with Deep Learning (IMLT-DL) based skin lesion segmentation and classification model using dermoscopic images. Primarily, the presented IMLT-DL model incorporates the Top hat filtering and inpainting technique for the preprocessing of the dermoscopic images. In addition, the Mayfly Optimization (MFO) with multilevel Kapur's thresholding-based segmentation process is used to determine the affected region. Besides, Inception v3 based feature extractor is applied to derive the useful set of feature vectors. Finally, the classification process is carried out using a Gradient Boosting Tree (GBT) model. The performance of the presented model takes place against International Skin Imaging Collaboration (ISIC) dataset and the experimental outcome is inspected in distinct evaluation measures. The resultant experimental values ensure that the proposed IMLT-DL model outperforms the existing methods by achieving a higher accuracy of 99.2%.Keywords
Cite This Article
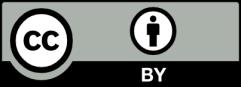