Open Access
ARTICLE
Multivariate Outlier Detection for Forest Fire Data Aggregation Accuracy
Alzaytoonah University of Jordan, Amman, 11733, Jordan
* Corresponding Author: Ahmad A. A. Alkhatib. Email:
Intelligent Automation & Soft Computing 2022, 31(2), 1071-1087. https://doi.org/10.32604/iasc.2022.020461
Received 25 May 2021; Accepted 03 July 2021; Issue published 22 September 2021
Abstract
Wireless sensor networks have been a very important means in forest monitoring applications. A clustered sensor network comprises a set of cluster members and one cluster head. The cluster members are normally located close to each other, with overlaps among their sensing coverage within the cluster. The cluster members concurrently detect the same event to send to the Cluster Head node. This is where data aggregation is deployed to remove redundant data at the cost of data accuracy, where some data generated by the sensing process might be an outlier. Thus, it is important to conserve the aggregated data’s accuracy by performing an outlier data detection process before data aggregation is implemented. This paper concerns evaluating multivariate outlier detection (MOD) analysis on aggregated accuracy of data generated by a forest fire environment using OMNeT++ and performing the analysis in MATLAB R2018b. The findings of the study showed that the MOD algorithm conserved approximately 59.5% of aggregated data accuracy, compared with an equivalent algorithm, such as the FTDA algorithm, which conserved 54.25% of aggregated data accuracy for the same event.Keywords
Cite This Article
Citations
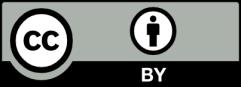