Open Access
ARTICLE
Ensemble Based Learning with Accurate Motion Contrast Detection
Hindusthan College of Engineering and Technology, Coimbatore, 641032, India
* Corresponding Author: M. Indirani. Email:
Intelligent Automation & Soft Computing 2023, 35(2), 1657-1674. https://doi.org/10.32604/iasc.2023.026148
Received 16 December 2021; Accepted 27 February 2022; Issue published 19 July 2022
Abstract
Recent developments in computer vision applications have enabled detection of significant visual objects in video streams. Studies quoted in literature have detected objects from video streams using Spatiotemporal Particle Swarm Optimization (SPSOM) and Incremental Deep Convolution Neural Networks (IDCNN) for detecting multiple objects. However, the study considered optical flows resulting in assessing motion contrasts. Existing methods have issue with accuracy and error rates in motion contrast detection. Hence, the overall object detection performance is reduced significantly. Thus, consideration of object motions in videos efficiently is a critical issue to be solved. To overcome the above mentioned problems, this research work proposes a method involving ensemble approaches to and detect objects efficiently from video streams. This work uses a system modeled on swarm optimization and ensemble learning called Spatiotemporal Glowworm Swarm Optimization Model (SGSOM) for detecting multiple significant objects. A steady quality in motion contrasts is maintained in this work by using Chebyshev distance matrix. The proposed system achieves global optimization in its multiple object detection by exploiting spatial/temporal cues and local constraints. Its experimental results show that the proposed system scores 4.8% in Mean Absolute Error (MAE) while achieving 86% in accuracy, 81.5% in precision, 85% in recall and 81.6% in F-measure and thus proving its utility in detecting multiple objects.Keywords
Cite This Article
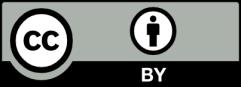