Open Access
ARTICLE
Optimized Neural Network-Based Micro Strip Patch Antenna Design for Radar Application
1 Dhanalakshmi Srinivasan Engineering College, Perambalur, 621212, Tamil Nadu, India
2 Sengunthar Engineering College, Tiruchengode, Namakkal, 637205, Tamil Nadu, India
* Corresponding Author: A. Yogeshwaran. Email:
Intelligent Automation & Soft Computing 2023, 35(2), 1491-1503. https://doi.org/10.32604/iasc.2023.026424
Received 25 December 2021; Accepted 01 March 2022; Issue published 19 July 2022
Abstract
Microstrip antennas are low-profile antennas that are utilized in wireless communication systems. In recent years, communication engineers have been increasingly interested in it. Because of downsizing, novelty, and cost reduction, the number of wireless standards has expanded in recent years. Wideband technologies have evolved in addition to analog and digital services. Radars necessitate antenna subsystems that are low-profile and lightweight. Microstrip antennas have these qualities and are suited for radars as an alternative to the bulky and heavyweight reflector/slotted waveguide array antennas. A perforated corner single-line fed microstrip antenna is designed here. When compared to the basic square microstrip antenna, this antenna has better specifications. Because key issue is determining the best values for various antenna parameters when developing the patch antenna. Optimized Neural Network (ONN) is one potential technique utilized to solve this issue, and this work also uses Particle Swarm Optimization (PSO) to enhance the antenna performance. Return loss (S11) and Voltage Standing Wave Ratio (VSWR) parameters are considered in all situations, developed with Advanced Design System (ADS) applications. The transmitters are made to emit in the Ku-band, which covers a wide range of wavelengths. From 5–15 GHz, it is used in most current radars. The ADS suite is used to create the simulation design.Keywords
Cite This Article
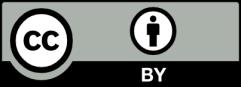