Open Access
ARTICLE
Spoofing Face Detection Using Novel Edge-Net Autoencoder for Security
1 Department of Computer Sciences, College of Computer and Information Sciences, Princess Nourah bint Abdulrahman University, Riyadh 11671, Saudi Arabia
2 Department of Electrical Electronics and Communication Engineering, GITAM School of Technology, GITAM Deemed to be University, Bengaluru Campus, Karnataka, 560065, India
3 Department of Computer Science and Engineering, CHRIST (Deemed to be University), Bangalore, 560074, India
4 Department of Mathematics, Faculty of Science, Mansoura University, Mansoura 35516, Egypt
5 Department of Computational Mathematics, Science, and Engineering (CMSE), Michigan State University, East Lansing, MI, 48824, USA
* Corresponding Author: Doaa Sami Khafaga. Email:
Intelligent Automation & Soft Computing 2023, 35(3), 2773-2787. https://doi.org/10.32604/iasc.2023.030763
Received 01 April 2022; Accepted 05 May 2022; Issue published 17 August 2022
Abstract
Recent security applications in mobile technologies and computer systems use face recognition for high-end security. Despite numerous security techniques, face recognition is considered a high-security control. Developers fuse and carry out face identification as an access authority into these applications. Still, face identification authentication is sensitive to attacks with a 2-D photo image or captured video to access the system as an authorized user. In the existing spoofing detection algorithm, there was some loss in the recreation of images. This research proposes an unobtrusive technique to detect face spoofing attacks that apply a single frame of the sequenced set of frames to overcome the above-said problems. This research offers a novel Edge-Net autoencoder to select convoluted and dominant features of the input diffused structure. First, this proposed method is tested with the Cross-ethnicity Face Anti-spoofing (CASIA), Fetal alcohol spectrum disorders (FASD) dataset. This database has three models of attacks: distorted photographs in printed form, photographs with removed eyes portion, and video attacks. The images are taken with three different quality cameras: low, average, and high-quality real and spoofed images. An extensive experimental study was performed with CASIA-FASD, 3 Diagnostic Machine Aid-Digital (DMAD) dataset that proved higher results when compared to existing algorithms.Keywords
Cite This Article
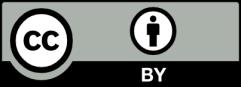