Open Access
ARTICLE
Multi-Level Deep Generative Adversarial Networks for Brain Tumor Classification on Magnetic Resonance Images
1 Radiological Sciences Department, College of Applied Medical Sciences, Najran University, Najran, 61441, Saudi Arabia
2 Department of Computer Science, COMSATS University Islamabad, Sahiwal Campus, Sahiwal, 57000, Pakistan
3 Electrical Engineering Department, College of Engineering, Najran University, Najran, 61441, Saudi Arabia
4 Anatomy Department, Medicine College, Najran University, Najran, Saudi Arabia
5 Department of Clinical Laboratory Sciences, Collage of Applied Medical Science, Najran University, Najran, Saudi Arabia
6 Department of Information Systems, College of Computer Science and Information Systems, Najran University, Najran, 61441, Saudi Arabia
* Corresponding Author: Ahmad Shaf. Email:
Intelligent Automation & Soft Computing 2023, 36(1), 127-143. https://doi.org/10.32604/iasc.2023.032391
Received 16 May 2022; Accepted 17 June 2022; Issue published 29 September 2022
Abstract
The brain tumor is an abnormal and hysterical growth of brain tissues, and the leading cause of death affected patients worldwide. Even in this technology-based arena, brain tumor images with proper labeling and acquisition still have a problem with the accurate and reliable generation of realistic images of brain tumors that are completely different from the original ones. The artificially created medical image data would help improve the learning ability of physicians and other computer-aided systems for the generation of augmented data. To overcome the highlighted issue, a Generative Adversarial Network (GAN) deep learning technique in which two neural networks compete to become more accurate in creating artificially realistic data for MRI images. The GAN network contains mainly two parts known as generator and discriminator. Commonly, a generator is the convolutional neural network, and a discriminator is the deconvolutional neural network. In this research, the publicly accessible Contrast-Enhanced Magnetic Resonance Imaging (CE-MRI) dataset collected from 2005-to 2020 from different hospitals in China consists of four classes has been used. Our proposed method is simple and achieved an accuracy of 96%. We compare our technique results with the existing results, indicating that our proposed technique outperforms the best results associated with the existing methods.Keywords
Cite This Article
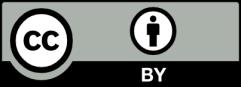