Open Access
ARTICLE
Performance Analysis of a Chunk-Based Speech Emotion Recognition Model Using RNN
1 Division of Software Convergence, Hanshin University, Osan-si, 18101, Korea
2 Division of AI Software Engineering, Pai Chai University, Daejeon, 35345, Korea
* Corresponding Author: Jun-Ki Hong. Email:
Intelligent Automation & Soft Computing 2023, 36(1), 235-248. https://doi.org/10.32604/iasc.2023.033082
Received 07 June 2022; Accepted 12 July 2022; Issue published 29 September 2022
Abstract
Recently, artificial-intelligence-based automatic customer response system has been widely used instead of customer service representatives. Therefore, it is important for automatic customer service to promptly recognize emotions in a customer’s voice to provide the appropriate service accordingly. Therefore, we analyzed the performance of the emotion recognition (ER) accuracy as a function of the simulation time using the proposed chunk-based speech ER (CSER) model. The proposed CSER model divides voice signals into 3-s long chunks to efficiently recognize characteristically inherent emotions in the customer’s voice. We evaluated the performance of the ER of voice signal chunks by applying four RNN techniques—long short-term memory (LSTM), bidirectional-LSTM, gated recurrent units (GRU), and bidirectional-GRU—to the proposed CSER model individually to assess its ER accuracy and time efficiency. The results reveal that GRU shows the best time efficiency in recognizing emotions from speech signals in terms of accuracy as a function of simulation time.Keywords
Cite This Article
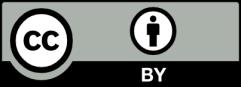