Open Access
ARTICLE
AttEF: Convolutional LSTM Encoder-Forecaster with Attention Module for Precipitation Nowcasting
1 School of Computer & Software, Engineering Research Center of Digital Forensics, Ministry of Education, Nanjing University of Information Science & Technology, Nanjing, 210044, China
2 Provincial Key Laboratory for Computer Information Processing Technology, Soochow University, Suzhou, 215325, China
3 Texas Tech University, Lubbock, TX79409, United States
* Corresponding Author: Wei Fang. Email: hsfangwei@ sina.com
Intelligent Automation & Soft Computing 2021, 30(2), 453-466. https://doi.org/10.32604/iasc.2021.016589
Received 05 January 2021; Accepted 19 April 2021; Issue published 11 August 2021
Abstract
Precipitation nowcasting has become an essential technology underlying various public services ranging from weather advisories to citywide rainfall alerts. The main challenge facing many algorithms is the high non-linearity and temporal-spatial complexity of the radar image. Convolutional Long Short-Term Memory (ConvLSTM) is appropriate for modeling spatiotemporal variations as it integrates the convolution operator into recurrent state transition functions. However, the technical characteristic of encoding the input sequence into a fixed-size vector cannot guarantee that ConvLSTM maintains adequate sequence representations in the information flow, which affects the performance of the task. In this paper, we propose Attention ConvLSTM Encoder-Forecaster(AttEF) which allows the encoder to encode all spatiotemporal information in a sequence of vectors. We design the attention module by exploring the ability of ConvLSTM to mergespace-time features and draw spatial attention. Specifically, several variants of ConvLSTM are evaluated: (a) embedding global-channel attention block (GCA-block) in ConvLSTM Encoder-Decoder, (b) embedding GCA-block in FconvLSTM Encoder-Decoder, (c) embedding global-channel-spatial attention block (GCSA-block) in ConvLSTM Encoder-Decoder. The results of the evaluation indicate that GCA-ConvLSTM produces the best performance of all three variants. Based on this, a new frame work which integrates the global-channel attention into the ConvLSTM encoder-forecaster is derived to model the complicated variations. Experimental results show that the main reason for the blurring of visual performance is the loss of crucial spatiotemporal information. Integrating the attention module can resolve this problem significantly.Keywords
Cite This Article
Citations
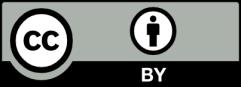