Open Access
ARTICLE
Deep Learning for Depression Detection Using Twitter Data
1 Department of Computer Sciences, College of Computer and Information Sciences, Princess Nourah bint Abdulrahman University, P.O. Box 84428, Riyadh, 11671, Saudi Arabia
2 Department of Computational Intelligence, SRM Institute of Science and Technology, Kattankulathur, Chennai, 603203, India
3 Department of Computer Science and Engineering, M. Kumarasamy College of Engineering, Karur, 639113, India
4 Department of Mathematics, Faculty of Science, Mansoura University, Mansoura, 35516, Egypt
5 Department of Computational Mathematics, Science, and Engineering (CMSE), College of Engineering, Michigan State University, East Lansing, MI, 48824, USA
* Corresponding Author: Faten Khalid Karim. Email:
Intelligent Automation & Soft Computing 2023, 36(2), 1301-1313. https://doi.org/10.32604/iasc.2023.033360
Received 15 June 2022; Accepted 01 August 2022; Issue published 05 January 2023
Abstract
Today social media became a communication line among people to share their happiness, sadness, and anger with their end-users. It is necessary to know people’s emotions are very important to identify depressed people from their messages. Early depression detection helps to save people’s lives and other dangerous mental diseases. There are many intelligent algorithms for predicting depression with high accuracy, but they lack the definition of such cases. Several machine learning methods help to identify depressed people. But the accuracy of existing methods was not satisfactory. To overcome this issue, the deep learning method is used in the proposed method for depression detection. In this paper, a novel Deep Learning Multi-Aspect Depression Detection with Hierarchical Attention Network (MDHAN) is used for classifying the depression data. Initially, the Twitter data was preprocessed by tokenization, punctuation mark removal, stop word removal, stemming, and lemmatization. The Adaptive Particle and grey Wolf optimization methods are used for feature selection. The MDHAN classifies the Twitter data and predicts the depressed and non-depressed users. Finally, the proposed method is compared with existing methods such as Convolutional Neural Network (CNN), Support Vector Machine (SVM), Minimum Description Length (MDL), and MDHAN. The suggested MDH-PWO architecture gains 99.86% accuracy, more significant than frequency-based deep learning models, with a lower false-positive rate. The experimental result shows that the proposed method achieves better accuracy, precision, recall, and F1-measure. It also minimizes the execution time.Keywords
Cite This Article
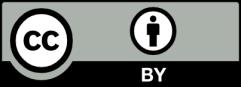