Open Access
ARTICLE
Graph Convolutional Neural Network Based Malware Detection in IoT-Cloud Environment
1 Department of Cybersecurity, College of Computer Science and Engineering, University of Jeddah, Jeddah, 21959, Saudi Arabia
2 Department of Information Systems, College of Computer and Information Sciences, Princess Nourah Bint Abdulrahman University, P.O.Box 84428, Riyadh, 11671, Saudi Arabia
3 Department of Computer Sciences, College of Computing and Information System, Umm Al-Qura University, Makkah, 24211, Saudi Arabia
4 Department of Computer and Self Development, Preparatory Year Deanship, Prince Sattam bin Abdulaziz University, AlKharj, 16242, Saudi Arabia
* Corresponding Author: Abdelwahed Motwakel. Email:
Intelligent Automation & Soft Computing 2023, 36(3), 2897-2914. https://doi.org/10.32604/iasc.2023.034907
Received 31 July 2022; Accepted 26 October 2022; Issue published 15 March 2023
Abstract
Cybersecurity has become the most significant research area in the domain of the Internet of Things (IoT) owing to the ever-increasing number of cyberattacks. The rapid penetration of Android platforms in mobile devices has made the detection of malware attacks a challenging process. Furthermore, Android malware is increasing on a daily basis. So, precise malware detection analytical techniques need a large number of hardware resources that are significantly resource-limited for mobile devices. In this research article, an optimal Graph Convolutional Neural Network-based Malware Detection and classification (OGCNN-MDC) model is introduced for an IoT-cloud environment. The proposed OGCNN-MDC model aims to recognize and categorize malware occurrences in IoT-enabled cloud platforms. The presented OGCNN-MDC model has three stages in total, such as data pre-processing, malware detection and parameter tuning. To detect and classify the malware, the GCNN model is exploited in this work. In order to enhance the overall efficiency of the GCNN model, the Group Mean-based Optimizer (GMBO) algorithm is utilized to appropriately adjust the GCNN parameters, and this phenomenon shows the novelty of the current study. A widespread experimental analysis was conducted to establish the superiority of the proposed OGCNN-MDC model. A comprehensive comparison study was conducted, and the outcomes highlighted the supreme performance of the proposed OGCNN-MDC model over other recent approaches.Keywords
Cite This Article
F. S. Alsubaei, H. M. Alshahrani, K. Tarmissi and A. Motwakel, "Graph convolutional neural network based malware detection in iot-cloud environment," Intelligent Automation & Soft Computing, vol. 36, no.3, pp. 2897–2914, 2023. https://doi.org/10.32604/iasc.2023.034907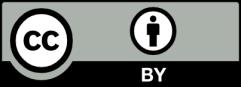