Open Access
ARTICLE
Ensemble Voting-Based Anomaly Detection for a Smart Grid Communication Infrastructure
1 Department of Computer Science, Faculty of Computing and Information Technology, King Abdulaziz University, Jeddah, 21589, Saudi Arabia
2 Self-Development Skills Department, Common First Year Deanship, King Saud University, Riyadh, 12211, Saudi Arabia
* Corresponding Author: Hend Alshede. Email:
Intelligent Automation & Soft Computing 2023, 36(3), 3257-3278. https://doi.org/10.32604/iasc.2023.035874
Received 08 September 2022; Accepted 04 November 2022; Issue published 15 March 2023
Abstract
Advanced Metering Infrastructure (AMI) is the metering network of the smart grid that enables bidirectional communications between each consumer’s premises and the provider’s control center. The massive amount of data collected supports the real-time decision-making required for diverse applications. The communication infrastructure relies on different network types, including the Internet. This makes the infrastructure vulnerable to various attacks, which could compromise security or have devastating effects. However, traditional machine learning solutions cannot adapt to the increasing complexity and diversity of attacks. The objective of this paper is to develop an Anomaly Detection System (ADS) based on deep learning using the CIC-IDS2017 dataset. However, this dataset is highly imbalanced; thus, a two-step sampling technique: random under-sampling and the Synthetic Minority Oversampling Technique (SMOTE), is proposed to balance the dataset. The proposed system utilizes a multiple hidden layer Auto-encoder (AE) for feature extraction and dimensional reduction. In addition, an ensemble voting based on both Random Forest (RF) and Convolutional Neural Network (CNN) is developed to classify the multiclass attack categories. The proposed system is evaluated and compared with six different state-of-the-art machine learning and deep learning algorithms: Random Forest (RF), Light Gradient Boosting Machine (LightGBM), eXtreme Gradient Boosting (XGboost), Convolutional Neural Network (CNN), Long Short-Term Memory (LSTM), and bidirectional LSTM (biLSTM). Experimental results show that the proposed model enhances the detection for each attack class compared with the other machine learning and deep learning models with overall accuracy (98.29%), precision (99%), recall (98%), F1 score (98%), and the UNDetection rate (UND) (8%).Keywords
Cite This Article
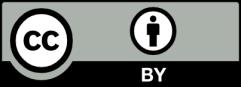