Open Access
ARTICLE
MNIST Handwritten Digit Classification Based on Convolutional Neural Network with Hyperparameter Optimization
1 Department of Electrical and Computer Engineering, University of Nevada Las Vegas, Las Vegas, 89154, NV, USA
2 Ed W. Clark High School, Las Vegas, 89102, NV, USA
3 School of Computer, Jiangsu University of Science and Technology, Zhenjiang, 212003, China
* Corresponding Author: Shengjie Zhai. Email:
Intelligent Automation & Soft Computing 2023, 36(3), 3595-3606. https://doi.org/10.32604/iasc.2023.036323
Received 26 September 2022; Accepted 14 November 2022; Issue published 15 March 2023
Abstract
Accurate handwriting recognition has been a challenging computer vision problem, because static feature analysis of the text pictures is often inadequate to account for high variance in handwriting styles across people and poor image quality of the handwritten text. Recently, by introducing machine learning, especially convolutional neural networks (CNNs), the recognition accuracy of various handwriting patterns is steadily improved. In this paper, a deep CNN model is developed to further improve the recognition rate of the MNIST handwritten digit dataset with a fast-converging rate in training. The proposed model comes with a multi-layer deep arrange structure, including 3 convolution and activation layers for feature extraction and 2 fully connected layers (i.e., dense layers) for classification. The model’s hyperparameters, such as the batch sizes, kernel sizes, batch normalization, activation function, and learning rate are optimized to enhance the recognition performance. The average classification accuracy of the proposed methodology is found to reach 99.82% on the training dataset and 99.40% on the testing dataset, making it a nearly error-free system for MNIST recognition.Keywords
Cite This Article
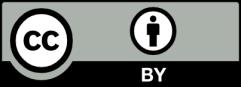