Open Access
ARTICLE
Machine Learning for Hybrid Line Stability Ranking Index in Polynomial Load Modeling under Contingency Conditions
1 Jawaharlal Nehru Technological University, Anantapur, Ananthapuramu
2 JNTUA College of Engineering, Constituent College of
Jawaharlal Nehru Technological Univeristy, Anantapur, Ananthapuramu
* Corresponding Author: P. Venkatesh. Email:
Intelligent Automation & Soft Computing 2023, 37(1), 1001-1012. https://doi.org/10.32604/iasc.2023.036268
Received 23 September 2022; Accepted 11 January 2023; Issue published 29 April 2023
Abstract
In the conventional technique, in the evaluation of the severity index, clustering and loading suffer from more iteration leading to more computational delay. Hence this research article identifies, a novel progression for fast predicting the severity of the line and clustering by incorporating machine learning aspects. The polynomial load modelling or ZIP (constant impedances (Z), Constant Current (I) and Constant active power (P)) is developed in the IEEE-14 and Indian 118 bus systems considered for analysis of power system security. The process of finding the severity of the line using a Hybrid Line Stability Ranking Index (HLSRI) is used for assisting the concepts of machine learning with J48 algorithm, infers the superior affected lines by adopting the IEEE standards in concern to be compensated in maintaining the power system stability. The simulation is performed in the WEKA environment and deals with the supervisor learning in order based on severity to ensure the safety of power system. The Unified Power Flow Controller (UPFC), facts devices for the purpose of compensating the losses by maintaining the voltage characteristics. The finite element analysis findings are compared with the existing procedures and numerical equations for authentications.Keywords
Cite This Article
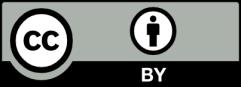