Open Access
ARTICLE
Flow Direction Level Traffic Flow Prediction Based on a GCN-LSTM Combined Model
1 Department of Transportation Engineering, Shandong University of Technology, Zibo, 255000, China
2 Center for Urban Transportation Research, University of South Florida, Tampa, 33620, USA
3 Road Traffic Safety Comprehensive Management Office, Traffic Police Detachment of Zibo Public Security Bureau, Zibo, 255000, China
* Corresponding Author: Yongqing Guo. Email:
Intelligent Automation & Soft Computing 2023, 37(2), 2001-2018. https://doi.org/10.32604/iasc.2023.035799
Received 04 September 2022; Accepted 06 November 2022; Issue published 21 June 2023
Abstract
Traffic flow prediction plays an important role in intelligent transportation systems and is of great significance in the applications of traffic control and urban planning. Due to the complexity of road traffic flow data, traffic flow prediction has been one of the challenging tasks to fully exploit the spatiotemporal characteristics of roads to improve prediction accuracy. In this study, a combined flow direction level traffic flow prediction graph convolutional network (GCN) and long short-term memory (LSTM) model based on spatiotemporal characteristics is proposed. First, a GCN model is employed to capture the topological structure of the data graph and extract the spatial features of road networks. Additionally, due to the capability to handle long-term dependencies, the long-term memory is used to predict the time series of traffic flow and extract the time features. The proposed model is evaluated using real-world data, which are obtained from the intersection of Liuquan Road and Zhongrun Avenue in the Zibo High-Tech Zone of China. The results show that the developed combined GCN-LSTM flow direction level traffic flow prediction model can perform better than the single models of the LSTM model and GCN model, and the combined ARIMA-LSTM model in traffic flow has a strong spatiotemporal correlation.Keywords
Cite This Article
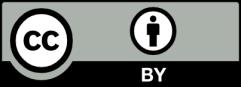