Open Access
ARTICLE
Prediction-Based Thunderstorm Path Recovery Method Using CNN-BiLSTM
1 School of Electronics and Information Engineering, Nanjing University of Information Science and Technology, Nanjing, 210044, China
2 Department of Electrical and Computer Engineering, University of Alberta, Edmonton, AB T6G 2R3, Canada
3 CCCC Highway Consultants Co., Ltd., Beijing, 100101, China
4 School of Geographical Sciences, Nanjing University of Information Science and Technology, Nanjing, 210044, China
5 State Key Laboratory of Resources and Environmental Information System, Institute of Geographic Sciences and Natural Resources Research, Chinese Academy of Sciences, Beijing, 100101, China
* Corresponding Author: Wenjie Zhang. Email:
(This article belongs to the Special Issue: Artificial Intelligence Algorithm for Industrial Operation Application)
Intelligent Automation & Soft Computing 2023, 37(2), 1637-1654. https://doi.org/10.32604/iasc.2023.039879
Received 22 February 2023; Accepted 06 April 2023; Issue published 21 June 2023
Abstract
The loss of three-dimensional atmospheric electric field (3DAEF) data has a negative impact on thunderstorm detection. This paper proposes a method for thunderstorm point charge path recovery. Based on the relationship between a point charge and 3DAEF, we derive corresponding localization formulae by establishing a point charge localization model. Generally, point charge movement paths are obtained after fitting time series localization results. However, AEF data losses make it difficult to fit and visualize paths. Therefore, using available AEF data without loss as input, we design a hybrid model combining the convolutional neural network (CNN) and bi-directional long short-term memory (BiLSTM) to predict and recover the lost AEF. As paths are not present during sunny weather, we propose an extreme gradient boosting (XGBoost) model combined with a stacked autoencoder (SAE) to further determine the weather conditions of the recovered AEF. Specifically, historical AEF data of known weathers are input into SAE-XGBoost to obtain the distribution of predicted values (PVs). With threshold adjustments to reduce the negative effects of invalid PVs on SAE-XGBoost, PV intervals corresponding to different weathers are acquired. The recovered AEF is then input into the fixed SAE-XGBoost model. Whether paths need to be fitted is determined by the interval to which the output PV belongs. The results confirm that the proposed method can effectively recover point charge paths, with a maximum path deviation of approximately 0.018 km and a determination coefficient of 94.17%. This method provides a valid reference for visual thunderstorm monitoring.Keywords
Cite This Article
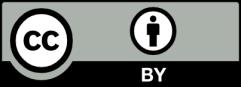