Open Access
ARTICLE
Machine Learning-Based Efficient Discovery of Software Vulnerability for Internet of Things
Department of Future Convergence Technology Engineering, Sungshin Women’s University, Seoul, 02844, Korea
* Corresponding Author: Il-Gu Lee. Email:
(This article belongs to the Special Issue: Advanced Achievements of Intelligent and Secure Systems for the Next Generation Computing)
Intelligent Automation & Soft Computing 2023, 37(2), 2407-2419. https://doi.org/10.32604/iasc.2023.039937
Received 25 February 2023; Accepted 18 May 2023; Issue published 21 June 2023
Abstract
With the development of the 5th generation of mobile communication (5G) networks and artificial intelligence (AI) technologies, the use of the Internet of Things (IoT) has expanded throughout industry. Although IoT networks have improved industrial productivity and convenience, they are highly dependent on nonstandard protocol stacks and open-source-based, poorly validated software, resulting in several security vulnerabilities. However, conventional AI-based software vulnerability discovery technologies cannot be applied to IoT because they require excessive memory and computing power. This study developed a technique for optimizing training data size to detect software vulnerabilities rapidly while maintaining learning accuracy. Experimental results using a software vulnerability classification dataset showed that different optimal data sizes did not affect the learning performance of the learning models. Moreover, the minimal data size required to train a model without performance degradation could be determined in advance. For example, the random forest model saved 85.18% of memory and improved latency by 97.82% while maintaining a learning accuracy similar to that achieved when using 100% of data, despite using only 1%.Keywords
Cite This Article
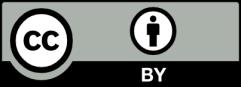