Open Access
ARTICLE
Abstractive Arabic Text Summarization Using Hyperparameter Tuned Denoising Deep Neural Network
1 Department of Computer Science, College of Science and Arts, Sharurah, Najran University, Sharurah, Saudi Arabia
2 Department of Applied Linguistics, College of Languages, Princess Nourah bint Abdulrahman University, P.O. Box 84428, Riyadh, 11671, Saudi Arabia
3 Department of Information Systems, College of Computing and Information System, Umm Al-Qura University, Makkah, Saudi Arabia
4 Department of Digital Media, Faculty of Computers and Information Technology, Future University in Egypt, New Cairo, 11835, Egypt
5 Computer Department, Applied College, Najran University, Najran, 66462, Saudi Arabia
6 Department of Computer and Self Development, Preparatory Year Deanship, Prince Sattam bin Abdulaziz University, AlKharj, Saudi Arabia
* Corresponding Author: Manar Ahmed Hamza. Email:
Intelligent Automation & Soft Computing 2023, 38(2), 153-168. https://doi.org/10.32604/iasc.2023.034718
Received 25 July 2022; Accepted 11 November 2022; Issue published 05 February 2024
Abstract
Abstractive text summarization is crucial to produce summaries of natural language with basic concepts from large text documents. Despite the achievement of English language-related abstractive text summarization models, the models that support Arabic language text summarization are fewer in number. Recent abstractive Arabic summarization models encounter different issues that need to be resolved. Syntax inconsistency is a crucial issue resulting in the low-accuracy summary. A new technique has achieved remarkable outcomes by adding topic awareness in the text summarization process that guides the module by imitating human awareness. The current research article presents Abstractive Arabic Text Summarization using Hyperparameter Tuned Denoising Deep Neural Network (AATS-HTDDNN) technique. The presented AATS-HTDDNN technique aims to generate summaries of Arabic text. In the presented AATS-HTDDNN technique, the DDNN model is utilized to generate the summary. This study exploits the Chameleon Swarm Optimization (CSO) algorithm to fine-tune the hyperparameters relevant to the DDNN model since it considerably affects the summarization efficiency. This phase shows the novelty of the current study. To validate the enhanced summarization performance of the proposed AATS-HTDDNN model, a comprehensive experimental analysis was conducted. The comparison study outcomes confirmed the better performance of the AATS-HTDDNN model over other approaches.Keywords
Cite This Article
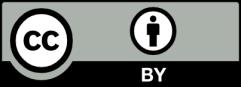