Open Access
ARTICLE
Malware Attacks Detection in IoT Using Recurrent Neural Network (RNN)
1 Computer Science Department, Imam Abdulrahman Bin Faisal University, Damam, 32232, Saudi Arabia
2 Department of Computer Science, Applied College, University of Tabuk, Tabuk, 47512, Saudi Arabia
3 Department of Computer Sciences, College of Computer and Information Sciences, Princess Nourah bint Abdulrahman University, P. O. Box 84428, Riyadh, 11671, Saudi Arabia
4 Department of Computer Sciences and Information Technology, Hazara University, Mansehra, Pakistan
5 Faculty of Computing, Department of Software Engineering, International Islamic University, Islamabad, Pakistan
6 Department of Architectural Engineering, Sejong University, Seoul, 05006, Korea
* Corresponding Author: Tan N. Nguyen. Email:
Intelligent Automation & Soft Computing 2024, 39(2), 135-155. https://doi.org/10.32604/iasc.2023.041130
Received 12 April 2023; Accepted 17 June 2023; Issue published 21 May 2024
Abstract
IoT (Internet of Things) devices are being used more and more in a variety of businesses and for a variety of tasks, such as environmental data collection in both civilian and military situations. They are a desirable attack target for malware intended to infect specific IoT devices due to their growing use in a variety of applications and their increasing computational and processing power. In this study, we investigate the possibility of detecting IoT malware using recurrent neural networks (RNNs). RNN is used in the proposed method to investigate the execution operation codes of ARM-based Internet of Things apps (OpCodes). To train our algorithms, we employ a dataset of IoT applications that includes 281 malicious and 270 benign pieces of software. The trained model is then put to the test using 100 brand-new IoT malware samples across three separate LSTM settings. Model exposure was not previously conducted on these samples. Detecting newly crafted malware samples with 2-layer neurons had the highest accuracy (98.18%) in the 10-fold cross validation experiment. A comparison of the LSTM technique to other machine learning classifiers shows that it yields the best results.Keywords
Cite This Article
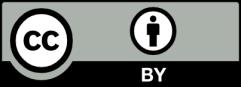