Open Access
ARTICLE
A Learning Framework for Intelligent Selection of Software Verification Algorithms
1 Shenzhen University, Shenzhen, China
2 Hangzhou Dianzi University, Hangzhou, China
3 Southern University of Science and Technology, Shenzhen, China
* Corresponding Author: Qiang Wang. Email:
Journal on Artificial Intelligence 2020, 2(4), 177-187. https://doi.org/10.32604/jai.2020.014829
Received 20 October 2020; Accepted 29 November 2020; Issue published 31 December 2020
Abstract
Software verification is a key technique to ensure the correctness of software. Although numerous verification algorithms and tools have been developed in the past decades, it is still a great challenge for engineers to accurately and quickly choose the appropriate verification techniques for the software at hand. In this work, we propose a general learning framework for the intelligent selection of software verification algorithms, and instantiate the framework with two state-of-the-art learning algorithms: Broad learning (BL) and deep learning (DL). The experimental evaluation shows that the training efficiency of the BL-based model is much higher than the DL-based models and the support vector machine (SVM)-based models, while the prediction accuracy of the DLbased model is much higher than other models.Keywords
Cite This Article
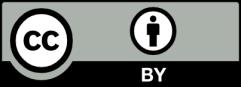