Open Access
ARTICLE
Evidence-Based Federated Learning for Set-Valued Classification of Industrial IoT DDos Attack Traffic
1 School of Computer Science, Nanjing University of Information Science and Technology, Nanjing, 210044, China
2 Jiangsu Collaborative Innovation Center of Atmospheric Environment and Equipment Technology (CICAEET), Nanjing University of Information Science and Technology, Nanjing, 210044, China
* Corresponding Author: Zilong Jin. Email:
Journal on Internet of Things 2022, 4(3), 183-195. https://doi.org/10.32604/jiot.2022.042054
Received 01 November 2022; Accepted 17 May 2023; Issue published 12 June 2023
Abstract
A novel Federated learning classifier is proposed using the Dempster-Shafer (DS) theory for the set-valued classification of industrial IoT Distributed Denial of Service (DDoS) attack traffic. The proposed classifier, referred to as the evidence-based federated learning classifier, employs convolution and pooling layers to extract high-dimensional features of Distributed Denial of Service (DDoS) traffic from the local data of private industrial clients. The characteristics obtained from the various participants are transformed into mass functions and amalgamated utilizing Dempster’s rule within the DS layer, situated on the federated server. Lastly, the set value classification task of attack mode is executed in the expected utility layer. A learning strategy is proposed to update the network parameters in a joint manner according to the weight of mass function value. Experiments on DDoS traffic classification tasks in CIC-DDoS2019 datasets prove that Federated learning, DS layer, and expected utility layer is effective in enhancing the multi-class classification accuracy, especially for those DDoS mixed attack traffic.Keywords
Cite This Article
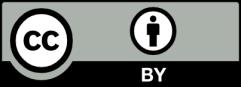