Open Access
REVIEW
Quality of Experience in Internet of Things: A Systematic Literature Review
Information System Department, Faculty of Computing and Information Technology, King Abdul Aziz University, Jeddah, Saudi Arabia
* Corresponding Author: Rawan Sanyour. Email:
Journal on Internet of Things 2022, 4(4), 263-282. https://doi.org/10.32604/jiot.2022.040966
Received 06 April 2023; Accepted 01 June 2023; Issue published 18 July 2023
Abstract
With the rapid growth of the Internet of Things paradigm, a tremendous number of applications and services that require minimal or no human involvement have been developed to enhance the quality of everyday life in various domains. In order to ensure that such services provide their functionalities with the expected quality, it is essential to measure and evaluate this quality, which can be in some cases a challenging task due to the lack of human intervention and feedback. Recently, the vast majority of the Quality of Experience QoE works mainly address the multimedia services. However, the introduction of Internet of Things IoT has brought a new level of complexity into the field of QoE evaluation. With the emerging of the new IoT technologies such as machine to machine communication and artificial intelligence, there is a crucial demand to utilize additional evaluation metrics alongside the traditional subjective and objective human factors and network quality factors. In this systematic review, a comprehensive survey of the QoE evaluation in IoT is presented. It reviews the existing quality of experience definitions, influencing factors, metrics, and models. The review is concluded by identifying the current gaps in the literature and suggested some future research directions accordingly.Keywords
In recent years, IoT technologies have emerged among fundamental aspects of our everyday lives. In 2011, CISCO stated in one of their reports that the total number of interconnected objects worldwide exceeded the number of human beings on our planet [1]. A report published by Statista Research Development indicated that the total number of connected IoT devices is dramatically increased to reach 75.44 billion worldwide by 2025, making a dramatic change in the digital domain. IoT paradigm is rapidly growing to cover substantial applications including healthcare, transportation, manufacturing, and smart cities. In such applications, the IoT technologies have an essential role in fine-grained monitoring and decision-making processes through generating valuable insights and recommendations.
Measuring the QoE of an application is a significant indicator for developers and designers since it provides them a mechanism to quantify the performance of their applications and services. Often, QoE is assessed using traditional subjective tests in which users are asked to evaluate the quality of an application or a service through some scales (e.g., Likert-like scales), and decisions are made consequently based on these rates. In the existing literature, the focus is mostly on evaluating the QoE for multimedia services through subjective human feedback for applications/services. However, When IoT services and applications deployed to support different industries, it is difficult to assess the quality of their performance (i.e., their ability to fulfill users’ satisfactions) using the traditional subjective metrics due to several reasons: first, as some of these applications are functioning with the minimal human involvement (i.e., the output of an automatic IoT application utilized by another IoT device), typically there is no human feedback available by which the quality of experience can be estimated in Realtime. Moreover, results from these applications are not just actions from actuation processes, but instead, they are generated from other IoT components that collect and analyze data in real-time. Assessing how such individual components affect the final provided quality is a grand challenge.
The crucial demand of evaluating the IoT services and applications objectively is enforced strongly by the fact that the existing traditional subjective measures evaluate the quality from end user’s perspective and focus mainly on user satisfaction, thus, not sufficient to deal with the complexity of modern IoT applications and services. In order to ensure that such applications function as expected, it is essential to evaluate their quality, which is challenging in the absence of humans in the loop. Recently, Minovski et al. [2,3] and Mitra et al. [4] have argued the demand of to understand and model the quality of IoT ecosystems wherein users can be both human and/or other systems and IoT devices. The rapid increase and adoption of analytic-driven actuations in these ecosystems has raised the need for assessing QoE objectively, as poor quality of decisions and resulting actions can degrade the quality leading to economic and social losses. Moreover, the distributed and heterogeneous nature of such systems makes them distinct from other QoE measurement methods in the literature. Given these facts, this review aims mainly to provide a wide vision of evaluating the quality of experience in the field of IoT. Its main contributions are:
• Reviewing the existing QoE definitions in the literature.
• Reviewing the state of the art of the QoE measurements in IoT, their models and influencing factors.
• Introducing the QoE as a level of aggregated values of various metrics measured as different IoT architecture layers.
• Summarizing the gaps in the existing literature and identifying some future research directions.
As the guidelines stated by Wolfswinkel et al. [5] and Biolchini et al. [6], in order to conduct a systematic literature review, there are four essential steps to be incorporated: 1) defining the review scope. 2) searching for initial articles. 3) selecting the most related works from this list. 4) analyzing the data in these selected studies. Fig. 1 depicts the research process followed in this review.
Figure 1: Research process
2.1 Defining the Scope of the Review
At this stage, the establishment of articles inclusion and exclusion criteria, the identification of appropriate research field, and, the selection and formulation of databases and search terms are performed.
A) Establishment of inclusion and exclusion criteria
In an attempt to sort the retrieved studies, inclusion criteria were identified to select appropriate studies to be included in this work. Studies had to: (1) be written in the English language; (2) explicitly discuss QoE in IoT context; and (3) should not be duplicated, that is if a similar text is found in other publications, then, one of these studies is considered in the review. To determine which of the collected publications to be included in this review, each must satisfy all these criteria, otherwise it is excluded. Note, since that the QoE in IoT paradigm is relatively new, there was no reason to identify a publishing-date inclusion criterion.
B) Identification of research field
Despite the fact that IoT is a research area that covers diverse domains and has a widespread impact across several fields, this study investigates the literature in which the quality of experience in IoT is addressed from computer science field perspective.
C) Selection of databases
Several scientific databases have been reviewed to extract studies that are relevant to the research questions. These databases are: Google Scholar, ACM (Association for Computing Machinery) library, Science Direct, IEEE Xplore, Springer Link. In order to enrich the potential articles list, The reference lists of the found articles were also searched to find additional articles that are related to the research area (backward snowballing approach) [5]. It has been noticed that some of the included articles are cited from more than one bibliographical database, these duplicated articles were automatically excluded from the study selection.
D) Formulation of research terms
The primary search keywords for this review were: (1) Quality of Experience, and (2) Internet of Things. Alternative terms such as QoE and IoT are also adopted in the search process to identify a comprehensive set of related papers to the area of study. The search has been started by using Boolean operators such as AND and OR to connect the two terms: Quality of Experience, and Internet of Things to search in the databases.
2.2 Searching for the Initial List of Papers
Using the formulated search terms, targeted papers were evaluated through their title, abstract, keywords and introduction as shown in Fig. 1.
2.3 Selecting the Relevant Articles
As illustrated in Fig. 1, initial papers were filtered for further comprehensive analysis. At first, 27 articles were downloaded based on our inclusion and exclusion criteria. After examining these articles, 7 of them were excluded based on reviewing their abstract, introduction and full text. In the snowballing process, additional 23 articles were selected. Finally, a total of 43 articles remained for the final analysis.
2.4 Synthesizing Data from the Selected Studies
The selected papers have been read, and findings have been extracted and analyzed based on some predefined themes. These themes are: QoE definitions, QoE influencing factors, QoE metrics, the need to go beyond the traditional QoE metrics, QoE in IoT, QoE models and metrics, challenges, and future directions. However, not every article addresses all defined themes, thus, they were read individually, and the themes were matched to their sections accordingly.
Reviewing the literature, it was noticeable that the vast majority of existing QoE definitions focus on applications in which humans are in the loop to provide their feedback regarding the performance of the intended service and/or application, and neglect to cover applications where humans are out of the loop. As stated by Table 1, QoE definitions can be categorized into: subjective QoE evaluation metrics and combined metrics wherein both subjective and objective measures are combined. Subjective measures include factors that are related to human perception such as satisfaction, happiness, feelings, expectations, desires, etc. On contrary, objective measures are quantitative metrics that reflect the actual performance of an application/service. Some works in the literature evaluated the services through objectively measure QoS parameters such as latency, packet loss, jitter, and throughput. However, these parameters do not reflect the actual users’ quality perception but the media and the network underlying the service.
Although subjective measures are considered the most utilized tools to grasp users’ opinions, they may fail to evaluate the QoE of real time applications and services [7]. It is worth noting that all these definitions focus on evaluating the quality of applications/services (particularly multimedia services) through users being involved and not consider machine experience, wherein, users are not always involved in the process. In IoT, the case is different since that IoT is a network of connected devices, considering device to device interaction is essential to evaluate application/service quality. Minovski et al. [2] introduced a new term to define the IoT quality of experience: Quality of IoT-experience (QoIoT). This term evaluates the performance of IoT service from two different perspectives: user centric QoE which address the regular quality of experience that address both subjective and objective human factors and machine-based quality, termed Quality of Machine Experience (QoME) by which machine performance is measured objectively.
As the QoE can be generally defined as the acceptance degree of a service/application by the end user, it can be seen as a subjective feeling users have when they interact with services and applications. The European Network Qualinet community defines QoE influence factor as “any characteristic of a user, system, service, application, or context whose actual state or setting may have influence on the Quality of Experience for the user’’ [9].
Because the interaction here is between humans and applications in a specific context, Brunnström et al. [9] have classified the QoE influence factors into: human, system and context. Human factors include any static or dynamic characteristics related to the user such as demographic information, socio-economic background, physical and mental state. System factors include all technical, media, network and device related characteristics by which the overall quality is affected. Context factors describe user’s surrounding environment regarding physical, temporal, and social characteristics. Juluri et al. [12] categorized the influence factors into four levels: system level in which the technical level factors such as network parameters (bandwidth, delay), device parameters (battery and configuration) and application parameters (codec, performance) are involved. Context level that encounters the environmental parameters such as user’s location and time. User level in which the user’s phycological factors such as expectations, history and interests are involved. Content level which considers the characteristics of the service.
Going further, Yang et al. [13] classified the QoE influencing factors into: objective and subjective factors. System and context parameters are categorized under objective factors while subjective factors consist of human parameters such as mental state, user profile, motivation and expectations. Authors emphasized that although objective factors have an essential role in the assessment process, subjective parameters are significant as they reflect the direct users’ perception. Table 2 illustrates some examples of parameters of each indicated factor.
Investigating the literature, it has been found that there are some conventional subjective metrics used to evaluate the overall QoE of services and applications. These metrics are: Mean opinion Score MOS, Standard Deviation of Opinion Score SOS by which users diversity, and its relation to MOS is reflected, Acceptability that reflects users satisfaction/dis-satisfaction, and the quantiles %GoB and %PoW, which estimate the percentage that the user judged the service as (good, better, poor, or worse). Table 3 shows the definition and description of each of them.
In addition to these measures that reflect the qualitative opinion of end users, Hoßfeld et al. [14], identified another type of metrics (called behavioral metrics) such as complaints, user engagements, and physiological metrics that can be used to indirectly infer the opinion of users through their behavior when using the service. Fig. 2 depicts the classification of these opinion and behavioral QoE metrics.
Figure 2: Classification of QoE measurements according to [14]
In order to qualify these subjective measures, authors applied them to real datasets to obtain deeper insights through comparing their MOS values to these quantities. They considered three types of services: speech, video and web services. Their experiments showed interesting results that justifies the necessity to go beyond the regular MOS metric. These results can be directly related to different service provisioning aspects from technical ones such as network management to marketing strategies customer support.
Using the experiments results, it has been proved that although MOS is a simple, practical and most utilized tool to apply, it hides some significant details that have an impact on service performance, thus the business aspect of such service. In addition, it is made evident that tools such as acceptability, by which a global quality threshold (same for all users) is defined, can bring more insights into QoE analysis. Despite that, this acceptability can reflect discrepant results according to the application under assessment, it is an essential metric when business considerations are significant. The other distributions and quantiles, e.g., GoB and PoW, are important as well in the case that more actionable information is required since they allow to gain a better understanding on how users perceive the quality of the used service and to what extent they happy or not.
3.3.1 The Need to go Beyond Conventional Subjective Metrics
MOS as mentioned earlier is a well-established metric, by which the perceived quality is quantified in both research literature and practical applications [15]. Due to its simplicity and effectiveness in various technical cases and scenarios, it can be utilized in several systems and applications such as performance evaluation of networking and SW applications. As a subjective measurement, MOS has been addressed by wealth of studies such as [16,17]. However, none of these works considered more complex metrics to analyze subjective data and estimate the QoE except [18] which discussed the significant limitations, alternatives and issues of MOS.
Often, researchers try to answer several different questions related to the service under study when they conduct subjective assessment. Such assessment is achieved either by explicitly asking users about their opinions or infer those opinions indirectly through some behavioral or psychological aspects. Restricting the results of subjective assessment into MOS values and neglecting such implicit aspects hides significant information regarding inter-user variation. For example, if the MOS value of a specific service is 3, there is no way to discover whether all users have rated the quality as “acceptable” (i.e., gave it 3 as a score) or if some of them rated the perceived quality as 5 while some others gave it 1 as a score or something in between. This type of measurements hides a significant percentage of users who rate the service as “unacceptable”, thus, poses the problem of the conflict between the service rate (good) and receiving customers complaints.
Hoßfeld et al. [14] have insist the necessity to go beyond standard quality measures, particularly the MOS in subjective quality assessments to gain a better understanding of how the quality is perceived by the user population. They identified the behavioral metrics as a new dimension to allow service providers to efficiently operate their services. They link the conventional subjective QoE measurements and the behavioral aspects such as acceptability and analyze its relation to MOS values to address the uncertainty masked by such measurement, thus, effectively provide more accurate QoE estimation.
Reviewing the existing QoE literature, it has been noticed that the vast majority of its definitions focus on the idea of assessing applications quality from regular users perspective. However, in IoT applications, in which human involvement in processes such as data collection, transformation, processing and making decisions is limited, poor quality decisions can significantly degrade the applications quality, thus, cause economic and social loss.
When IoT applications are designed and implemented, often, it is difficult to evaluate their actual performance and quality to ensure that they meet the specified KPIs and quality specifications. Fizza et al. [19] have stated multiple reasons for such difficulty. First, as stated earlier, in IoT applications, there is no human feedback through which the QoE of the application can be assessed in real time. Second, results we gained from IoT applications are not just simple actuations (i.e., applying specific settings to a specific machine), instead, they are generated by the integration of multiple components including sensors for collecting data, data processing and analytics tools and making decisions accordingly. Fig. 3 shows the stages of IoT applications lifecycle. Evaluating how these integrated components can affect the final results quality is a challenging task. Furthermore, with the introducing of edge computing paradigm, by which data storage and analysis are carried out at the network edge without the need to move them to a distant data center, additional complexities are added due to its distribution nature. This distributed data processing IoT applications require continuous quality evaluation.
Figure 3: Stages of IoT applications lifecycle
Recently, Mitra et al. [4] and Minovski et al. [2,3] have argued the fact that the output of an IoT application may be utilized by another IoT device rather than a human, thus, users’ feedback is not available. Based on this idea, they emphasized that, in ecosystems such as IoT system, users can be both a human and/or other IoT machine. Therefore, there is an urgent demand for more objective measures (using mathematical/statistical models) in addition to the trivial subjective ones to consider device to device interaction in IoT applications.
The demand to assess the QoE objectively is essential to control the delivered service as it is perceived by the end user, thus, optimizing quality while controlling the utilized IoT system resources. Floris et al. [20] have categorized the IoT applications according to end user orientation. In user oriented IoT applications, humans have an essential role in the evaluation process, since they are the fundamental beneficiaries from the content provided by the application. On the other hand, in system oriented IoT applications, data is automatically acquired, analyzed, and managed in order to perform a specific task and make a specific decision. However, authors emphasized that users participation should be always considered even with system-oriented applications as they have the task of smart controllers.
3.4.1 QoE Evaluation in IoT Environment
Even though subjective quality evaluating techniques, in which the quality of a services/application is measured through users’ feedback and tools such as MOS, differential MOS and the ACR-HR [21–23], are the most popular used techniques, recently, their efficiency is widely questioned by researchers. In IoT, there are some automatic applications, wherein human feedback is not always available, thus, only subjective metrics may not be suitable to evaluate their overall QoE. Therefore, there is a substantial demand for identifying some metrics on which the application quality is based. As mentioned earlier, since that evaluating the QoE in IoT is a relatively new research area, there is still no consensus on well-defined metrics to assess the quality of services and applications. In this section, metrics were classified into: Quality of Service QoS-based metrics, Quality of Data QoD-based metrics, and combined metrics. Table 4 illustrates these metrics and their related references.
Quality of Service QoS Metrics
IoT services can range from application layer functions by which users can control electronic devices to perform some specific daily life functions in their smart homes such as locking/unlocking doors, turning on/off lights, etc., to other applications including smart healthcare, smart agriculture and smart cars. As the quality of these services are essentially based on the performance of the network they rely on, studying the quality of user experience is vitally essential to provide satisfactory services [24]. At the same time, IoT services are often used and experienced by end users, thus, QoE subjective evaluation needs focus as well [25]. QoS factors can be defined as “the ability of the network to provide a service with an assured service level” [26]. As the IoT consists of technical infrastructure and services targeted end user, it is essential to integrate both QoS and QoE appropriately. Shaikh et al. [27] argued that research on QoE and QoS has been fragmentary and their relation remains blurry with the recent emerging technologies such as IoT.
Khamosh et al. [28] emphasized the fact that the connection between the IoT devices is basically depends on a wireless network due to their geographically location distribution. Often, wireless networks are unreliable and have their distortion rates are high. Therefore, the network level QoS factors such as latency, jitter and pocket loss can negatively affect overall performance of IoT services. As mentioned earlier, although the relationship between QoS factors and QoE has been significantly addressed in multimedia services context, however, there is a lack of studies on the effect of the network performance factors on the QoE of IoT services, thus, there is an evident room to explore such impact.
In order to investigate the correlation between three QoS parameters: packet loss, latency and jitter and the QoE for IoT services, Khamosh et al. [28] conducted a subjective experiment and a real time IoT service controlling testbed. The selected QoS parameters were investigated individually to discover their impact on the QoE. They found that all these factors have a considerable relationship with the QoE of IoT services. However, packet loss has the most significant impact on the quality of the provided services, latency came second and the last is jitter with the weakest correlation. Another work [29] aimed to evaluate the QoE through the QoS parameters. Authors proposed a mathematical model between QoS and QoE. The model utilized the Principal Component Analysis PCA to conduct the principal components and use these components to deduce the regression equation that can be used to connect QoS and QoE.
Fizza et al. [19] argued that QoS metrics such as throughput, delay, packet loss, reliability and availability are precise in definition and reflect the actual performance of the targeted network. However, they are crude, and don’t provide a real view of the quality of IoT application.
Data represents a valuable asset in IoT paradigm. It plays an essential role in evaluating the quality of IoT application/service since this data is sensed, analyzed, and then used to actuate some actions. Data quality refers to what extent data meets its consumers requirements. It means how suitable the gathered data to provide satisfiable services to the users. Therefore, it has become a product that is judged by end users rather than information systems professionals. Given this, the existing literature is surveyed to investigate the criteria, aspects and dimensions on which the quality of data in IoT is tackled.
A) IoT data lifecycle
As depicted in Fig. 4, the well-known traditional Internet lifecycle data is produced by people using their own computers and at the same time utilized to provide services for these same people. In contrast, in IoT, things are the main producers and consumers of the generated data to provide different services. Here, data can be considered as the main communication tool in machine-to-machine paradigm that allows IoT devices to communicate and collaborate to produce new services. In IoT, data performs a valuable asset that can provide insights regarding people, entities and surrounding environment. Such insights can be utilized to provide intelligent services. if such data is generated inaccurately for any reason, consequently, the extracted information and knowledge will probably be unsound [30,31].
Figure 4: Data lifecycle in the conventional internet and IoT [47]
B) IoT data characteristics
Often, IoT objects monitor some variables in the surrounding environment such as temperature, heart rate, sleep habits, etc. Moreover, since the fact that the harvesting of such data occurs in continuously changing environment, several characteristics distinguish it from other types of data. These characteristics are [47]:
• Uncertainty, nosiness and erroneousness: due to the several factors that can affect the accuracy of gathered data, it can be uncertain and erroneous.
• Voluminous and distributed: usually, in IoT, data sources are physically and logically distributed all over the world. Data generation rate can be easily overwhelmed.
• Smoothly variated: often, the physically monitored variables in IoT show smooth variations in which small or even no variations occur in two adjacent timestamps.
• Correlation: IoT data values are often correlated. These data are either temporally or spatially correlated or both.
• Periodic: the generated datasets that related to several phenomenon may appear in a periodic pattern in which same values can periodically occur at specific time intervals.
• Continuous: data in IoT is generated in a continuous form even in the case of adopting some sampling strategies. Often, sampling techniques are used for energy efficiency purposes because the monitored variables of interest do not change suddenly.
C) IoT data quality
Karkouch et al. [33] proposed a survey paper that aimed to broadly investigate data quality in IoT. They identified data properties, life cycle, and the factors that significantly impact the data quality in IoT environment. Furthermore, they identified data outliers as one of the factors that have an adverse effect on the quality of data. Lui et al. also conducted a systematic literature review about data quality in IoT. They categorized data quality dimensions, data quality issues manifestations, and provide some methods to measure data quality. Finally, they suggested some new research areas for further analysis and investigations. The most popular data quality assessment metrics are: accuracy, timeliness, completeness, and usefulness. Table 5 illustrates these four dimensions, their definitions, and some related works. The table indicates that accuracy is the most utilized metric in data quality assessment and timeliness comes next. It also shows that some works combined two or more metrics in the assessment process.
Pal et al. [36,40] modeled the relationship between users experience and quality perception in smart wearable applications domain. They represented the QoE as a function of QoD and Quality of Information QoI, i.e., the quality of the information that meets user’s requirement at a specific time, place and social settings. Steps counts and heart rates that are read by the wearable devices are used to measure the QoD while the information richness, ease of use and the perceived usefulness are used to measure to evaluate the QoI. In such applications, authors identified three significant factors by which the quality of this type of applications is determined: the quality of the embedded sensors in devices, the algorithms that utilized to analyze the collected raw data and the way the application present these data to users (applications characteristics).
Their quantitative approach is based on comparing balanced, correlation, hybrid and priority weighted averages to calculate the overall QoE through considering multiple parameters from both QoD and QoI. In order to build their mathematical model, a subjective experiment is conducted in a free-living environment. The accuracy of their model is tested by comparing the QoE obtained from the mathematical model and the subjective test. Although their work considered some significant user centric factors such the QoI and their model achieved 0.65 and 0.63 accuracy values for R2 and adjusted R2 measures respectively, they neglected the impact of the technology centric measurements such as QoS which are important in the case of IoT applications.
In [34], authors emphasized that due to the tremendous amount of data from IoT devices, any type of errors from user entry, data sensing, integrations and processing can cause a vital error that consequently affect the decision making process. Therefore, they stated that the quality of data is essential not only at the device and network layer, but at the application layer as well. Their work identified several challenges and solutions regarding the impact of the quality of data at the Open Systems Interconnection (OSI) network model levels. However, their proposed approach considers only subjective techniques i.e., users feedback to assess the application quality.
In [35] authors studied the role of data in managing the performance of QoS/QoE through proposing a data driven QoS/QoE management framework in smart cities. They emphasized that in smart cities, metrics such as error rates and loss rates can significantly affect the quality of data directly in addition to the traditional QoS metrics. Their framework consists of online and offline modules by which the QoS and QoE performance is predicted. In the offline modules, data such as communication context, the perceived experience of the target and neighbors objects are collected and used to build a trained module using machine learning techniques. This module is then utilized to predict the QoS/QoE performance. Although the proposed work seems promising, some phases such as gathering and evaluating users feedback, for example, was not explained, in addition, it was proposed theoretically as a tutorial study without implementing and testing its validity.
Minovski et al. [2] used metrics such as data, network and context quality to evaluate the overall quality in IoT autonomous vehicles. They broaden the QoE paradigm to address the relationship between human and intelligent machines. The term QoIoT in autonomous vehicles was first introduced by authors through which the quality assessment considers the perspective of the intelligent IoT machines beside the traditional user subjective metrics and the objective evaluation metrics. Authors emphasized the significance of data accuracy in evaluating the performance of IoT applications (collision free working of autonomous vehicles in their case study). In [38] authors conducted an in depth analysis to address the issues of evaluating QoE in IoT applications. In their analysis, they provide several reasons to justify their discouragement of conducting a traditional subjective quality assessment for evaluating the quality of IoT applications. Finally, they defined some of best practices for designing IoT applications by which some useful information about end users could be collected and utilized to predict the QoE.
In summary, broadly investigating the literature, it is worth noting that despite the diversity of the proposed works, the vast majority of them emphasized the crucial role of data in evaluating the overall quality of IoT applications/services.
IoT applications are not limited to multimedia services but rather, they involve additional functionalities such as gathering data from sensors and analyzing it to gain meaningful knowledge through some intelligent processes. As a result, the performance of such services/applications is not reflected by considering the network QoS metrics alone. realizing this idea, Floris et al. [20] introduced a layered QoE model that evaluate and combine several influence factors to estimate the overall QoE in multimedia IoT applications. In this model, each layer models the quality required by the specific IoT layer and has the ability to be combined with both upper and lower layers. Such feature allows for building a model wherein the output of one layer can be interpreted and enhanced by higher layers. The model consists of five layers: physical layer, network layer, virtualization layer, combination layer and application layer. In order to test their model, it is experimented with two use cases with comprehensive analysis: a smart surveillance application and multimedia vehicle application. Three parameters were subjectively evaluated by 24 people: the video quality, the synchronization and vehicle data accuracy. Their results indicated that users expect to have accurate and synchronized data. in terms of video quality and data presentations, results showed that they don’t have a great impact on the overall QoE. Although their model considered some significant influence factors such as the accuracy of data rather than just estimating the QoS factors, its attention was mainly focused on multimedia IoT applications.
A scalable layered QoE evaluation model that takes into account various quality metrics has been also proposed by Ikeda et al. [41]. They introduced the new terms: physical and metaphysical metrics. Physical metrics are related to the IoT architecture metrics such as network, sensing, and computation quality while metaphysical metrics represent the quality metrics that end users often require. The physical metrics were further categorized into static and dynamic metrics according to their relation to the surrounding situation. The metaphysics metrics are strongly attached with concepts of Quality of Context QoC and QoI that were proposed in some other studies such as [42,43]. The proposed work is based basically on finding the relation between these two types of metrics. For example, the accuracy of context can be considered as a function of physical metrics since that it depends significantly on the accuracy of the gathered data at the sensing layer, transferred through the network layer, analyzed at the computation layer and presented to the user at the presentation layer. Although the work aimed to maximize users’ satisfaction at the minimum cost, it has not been evaluated and tested. Moreover, how to determine the formulas and their coefficients remained unclear. Jagarlamudi et al. [48] proposed a survey that studied the functional and quality characteristics associated with the context consumer’s QoC requirements. They identified the limitations in the current QoC functionalities and modeling approaches to obtain adequate QoC, and thus, enhance context consumers’ QoE. Although their study is limited to only one IoT quality metric, they highlighted the direct impact of different QoS metrics considering both context processing and delivery phases. They emphasized the explicit assertion of identifying the QoC metrics affected by each QoS metric as such impact is essential in the context management platforms. QoS metrics such as availability, reliability, and scalability, that are related to context processing phase, have been proved to have a direct effect on the spatial and temporal QoC characteristics of high-level context namely: significance, completeness, confidence, timeliness, and resolution. Furthermore, QoS metrics related to the networks have been proved to affect the context during context delivery phase. For example, Response time, delay, jitter, bandwidth, loss rate, error rate, etc. directly some of QoC metrics such as timeliness, precision, and accuracy.
Instead of a regular layered QoE model, Karaadi et al. [45] proposed a cross layered Quality of Things QoT model for multimedia IoT applications. In the traditional layered models, information is only shared between the adjacent layers while in crossed layers models provide a direct communication between non-adjacent layers. Th proposed model consists of five layers: physical layer, network layer, virtual layer, service layer and application layer. In this work, authors introduced a new concept which is QoT for multimedia communication in IoT. They argued that with the tremendous number of connected devices/objects in IoT, machine to machine M2M communications will be the dominant applications. However, the elements of the identified QoT concept (environmental factors, application factors, network factors and device factors) is quite similar as some other works in the literature such as [41].
Shin [25] introduced a study by which the relation between users experience and quality perception has been examined. In addition, they developed a conceptual QoE model in personal informatics. The work characterized the QoS metrics and conducted a subjective evaluation to compare the QoS to QoE. Shin [25] identified three factors that influence QoE: content quality, system quality and service quality. Content quality is related to the reliability and timeliness of the knowledge provided by IoT services. within the literature, the term “content quality” is often used interchangeably with “information content”. In another work, based on factors analysis, Shin [44] found that content quality is a significant factor in any technology adoption. System quality is the evaluation of system’s performance from user’s perspective, thus, has a strong relation with his satisfaction. Several research works found that IoT has been significantly affected by system quality. Service quality is the evaluation of how well a specific service meets user’s requirements. In IoT, with the increased levels of automation, considering service quality is critical in determining the success of IoT services. comparing the QoS and QoE factors in three different layers: application, network and sensing, Shin found that the experiment’s participants considered the application layer QoS factors as the most significant factors followed by sensing and network layers. Although the author emphasized that the study can be considered as a groundwork to develop IoT services that meet users QoE requirements, his findings cannot be generalized to the overall QoE estimation.
Wu et al. [46] defined a new paradigm called Cognitive Internet of Things (CIoT) wherein both physical and virtual are connected and act as agents with the minimum user intervention. The defined framework measures three different factors: QoD, QoI, and user QoE that focus on metrics in four different levels: access, communication, computation, and application. For each of IoT architecture layer: sensing, networking and application, Authors defined a monitoring module that works as a function of the measured QoS metrics: information accuracy, sensing precision and energy consumption at the sensing layer, bandwidth, delay and throughput at the networking layer and service cost, performance time and reliability at the application layer.
In summary, as illustrated in Table 6, some of the defined metrics can be directly associated with the IoT architecture, thus, quality evaluation processes could be accomplished separately at different layers. Then, the overall QoE is predicted based on these combined metrics.
4 Challenges and Future Research Directions
IoT applications differ from other traditional applications in the fact that they are inherently distributed in both computation processes and the contribution of these distributed components in the applications outcomes. Therefore, it is essential to determine how different quality factors such as QoD, QoS, QoI and QoC that have been identified in the literature affect the stages of IoT applications life cycle, i.e., sensing, analytics, and actuating. Following some of the challenges and research gaps found in the literature:
• Security. As mentioned above, IoT applications are physically and logically distributed on several networks due to its nature. Thus, sensing components in the sensing stage can be on one network while data analysis components at the analytics stage can be on another network. This necessitates transferring data between different networks/clouds which consequently renders threats to the application security and the privacy of IoT system. Further investigations are required to tackle such issue while maintaining acceptable QoE for IoT applications.
• Traditionally, QoE is measured through subjective evaluation metrics using techniques such as MOS, DMOS and ACR-HR. research in IoT area should focus on finding other techniques that consider the quality of things for machine-to-machine M2M autonomic IoT applications where human feedback is not always available.
• The vast majority of current QoE research is limited to a single user use case. More efforts should be made to consider system level QoE with large scale IoT systems and massive users.
• The distribution nature of IoT applications across several clouds, edges and networks significantly affect their responsiveness specially of latency sensitive applications. Such impact should be tackled while maintaining acceptable QoE for IoT applications.
• Often, IoT applications/systems work in real time, thus, novel methods for estimating real time QoE are essential to gain better QoE prediction accuracy values.
• Despite the diversity of works proposed in the QoE research area, ranging from in depth analysis to layered prediction models, there is no globally well-defined methodology/approach for defining QoE evaluation models to assess the overall IoT applications quality.
• Till now, all the proposed works are suitable for specific application domain such as smart city, multimedia, wearable devices, etc. with specific scenarios. There is no QoE estimation model that can be general enough to be broadened to multiple IoT domains, albeit it is a challenging task that requires extensive research efforts.
• Lastly, more efforts should be focused on building the bridge from theory works to practice. To determine to what extent the theoretical studies in the literature can be utilized and applied in real world use case scenarios.
This systematic review provides an extensive vision of understanding the QoE in IoT environment. The existing literature is reviewed including the various QoE definitions which as noticed are mainly focused on users’ feedback. In addition, an in-depth analysis is conducted to investigate the proposed QoE models, metrics and influencing factors. Investigations proved that, unlike the traditional applications wherein quality can be estimated by evaluating subjective measures, IoT applications require more advance metrics such as QoD, QoI and QoC to evaluate their overall quality. In addition, there is a crucial demand for developing QoE models for quality estimation of autonomic IoT applications with limited or no human intervention. Quality of machines is another topic that required to be attentional (except [2], there is no other work that considered the quality of machines experience). Subsequently, a set of challenges and future research directions are provided to be considered. This review indicated that there is still a gap in QoE estimation for IoT applications and proved that there is room for further research.
Funding Statement: The authors received no specific funding for this study.
Conflicts of Interest: The authors declare that they have no conflicts of interest to report regarding the present study.
References
1. E. Dave, “The internet of things how the next evolution of the internet is changing everything,” 2011. http://www.cisco.com/web/about/ac79/docs/innov/IoT_IBSG_0411FINAL.pdf [Google Scholar]
2. D. Minovski, C. Ahlund and K. Mitra, “Modeling quality of IoT experience in autonomous vehicles,” IEEE Internet Things J., vol. 7, no. 5, pp. 3833–3849, 2020. [Google Scholar]
3. D. Minovski, C. Ahlund, K. Mitra and R. Zhohov, “Defining quality of experience for the internet of things,” IT Prof., vol. 22, no. 5, pp. 62–70, 2020. [Google Scholar]
4. K. Mitra, A. Zaslavsky and C. Ahlund, “Context-aware QoE modelling, measurement, and prediction in mobile computing systems,” IEEE Trans. Mob. Comput., vol. 14, no. 5, pp. 920–936, 2015. [Google Scholar]
5. J. F. Wolfswinkel, E. Furtmueller and C. P. M. Wilderom, “Using grounded theory as a method for rigorously reviewing literature,” Eur. J. Inf. Syst., vol. 22, no. 1, pp. 45–55, 2013. [Google Scholar]
6. J. Biolchini, P. Gomes Mian, A. Candida Cruz Natali and G. Horta Travassos, “Systematic review in software engineering,” Systems Engineering and Computer Science Department, Technical Report, 2005. [Google Scholar]
7. W. Pan, G. Cheng, H. Wu and Y. Tang, “Towards QoE assessment of encrypted YouTube adaptive video streaming in mobile networks,” in IEEE/ACM 24th Int. Symp. Qual. Serv., Beijing, 2016. [Google Scholar]
8. “ITU-T. Definition of Quality of Experience (QoE),” Itu, 2007. [Online]. Available: https://www.itu.int/md/T05-FG.IPTV-IL-0050/en [Google Scholar]
9. K. Brunnström, S. A. Beker, K. De Moor, A. Dooms, S. Egger et al., “Qualinet white paper on definitions of quality of experience,” 2013. [Google Scholar]
10. ETSI, “Human factors (HF); quality of experience (QoE) requirements for real-time communication services,” Tech. Rep., vol. 1, pp. 1–37, 2009. [Google Scholar]
11. W. Wu, A. Arefin, R. Rivas, K. Nahrstedt, R. Sheppard et al., “Quality of experience in distributed interactive multimedia environments: Toward a theoretical framework,” in MM’09—Proc. 2009 ACM Multimed. Conf. with Co-Located Work. Symp., Beijing, China, pp. 481–490, 2009. [Google Scholar]
12. P. Juluri, V. Tamarapalli and D. Medhi, “Measurement of quality of experience of video-on-demand services: A survey,” IEEE Commun. Surv. Tutorials, vol. 18, no. 1, pp. 401–418, 2016. [Google Scholar]
13. M. Yang, S. Wang, R. N. Calheiros and F. Yang, “Survey on QoE assessment approach for network service,” IEEE Access, vol. 6, pp. 48374–48390, 2018. [Google Scholar]
14. T. Hoßfeld, P. E. Heegaard, M. Varela and S. Möller, “QoE beyond the MOS: An in-depth look at QoE via better metrics and their relation to MOS,” Qual. User Exp., vol. 1, no. 1, pp. 1–23, 2016. [Google Scholar]
15. “ITU-T Recommendation P.800.1. Mean opinion score (MOS) terminology. International Telecommunication Union, Mar. 2003”. [Google Scholar]
16. P. Mohammadi, A. Ebrahimi-Moghadam and S. Shirani, “Subjective and Objective Quality Assessment of Image: A Survey,” Prepr. Submitt. to Elsevier, 2014. [Google Scholar]
17. M. Mu, A. Mauthe, G. Tyson and E. Cerqueira, “Statistical analysis of ordinal user opinion scores,” in IEEE Consum. Commun. Netw. Conf., Las Vegas, NV, USA, 2012. [Google Scholar]
18. R. C. Streijl, S. Winkler, D. S. Hands, R. C. Streijl, S. Winkler et al., “Multimedia systems mean opinion score (MOS) revisited: Methods and applications, limitations and alternatives,” Multimed. Syst., vol. 22, pp. 213–227, 2016. [Google Scholar]
19. K. Fizza, A. Banerjee, K. Mitra, P. P. Jayaraman, R. Ranjan et al., “QoE in IoT: A vision, survey and future directions,” Discov. Internet Things, vol. 1, pp. 1–14, 2021. [Google Scholar]
20. A. Floris and L. Atzori, “Managing the quality of experience in the multimedia internet of things: A layered-based approach,” Sensors (Switzerland), vol. 16, no. 12, pp. 2057, 2016. [Google Scholar]
21. M. Suryanegara, D. A. Prasetyo, F. Andriyanto and N. Hayati, “A 5-step framework for measuring the quality of experience (QoE) of internet of things (IoT) services,” IEEE Access, vol. 7, pp. 175779–175792, 2019. [Google Scholar]
22. J. Song, F. Yang, Y. Zhou, S. Wan and H. R. Wu, “QoE evaluation of multimedia services based on audiovisual quality and user interest,” IEEE Trans. Multimed, vol. 18, no. 3, pp. 444–457, 2016. [Google Scholar]
23. J. Van Der Hooft, M. T. Vega, C. Timmerer, A. C. Begen, F. De Turck et al., “Objective and subjective QoE evaluation for adaptive point cloud streaming,” in 2020 12th Int. Conf. Qual. Multimed. Exp. QoMEX 2020, Athlone, Ireland, pp. 1–6, 2020. [Google Scholar]
24. G. Gardašević, M. Veletić, N. Maletić, D. Vasiljević, I. Radusinović et al., “The IoT architectural framework, design issues and application domains,” Wirel. Pers. Commun., vol. 92, no. 1, pp. 127–148, 2017. [Google Scholar]
25. D. H. Shin, “Conceptualizing and measuring quality of experience of the internet of things: Exploring how quality is perceived by users,” Inf. Manag., vol. 54, no. 8, pp. 998–1011, 2017. [Google Scholar]
26. D. Pal and V. Vanijja, “Effect of network QoS on user QoE for a mobile video streaming service using H.265/VP9 codec,” Procedia Comput. Sci., vol. 111, pp. 214–222, 2017. [Google Scholar]
27. J. Shaikh, M. Fiedler and D. Collange, “Quality of experience from user and network perspectives,” Ann. Des. Telecommun. Telecommun., vol. 65, no. 1–2, pp. 47–57, 2010. [Google Scholar]
28. A. Khamosh, M. A. Anwer, N. Nasrat, J. Hamdard, G. S. Gawhari et al., “Impact of network QoS factors on QoE of IoT services,” in CIT 2020—5th Int. Conf. Inf. Technol., Chonburi, Thailand, pp. 61–65, 2020. [Google Scholar]
29. L. Li, M. Rong and G. Zhang, “An internet of things QoE evaluation method based on multiple linear regression analysis,” in 10th Int. Conf. Comput. Sci. Educ. ICCSE 2015, Cambridge, UK, no. Iccse, pp. 925–928, 2015. [Google Scholar]
30. C. C. Aggarwal, N. Ashish and A. Sheth, “The internet of things: A survey from the data-centric perspective,” In: C. Aggarwal (Ed.Managing and Mining Sensor Data. Boston, MA: Springer, 2013. [Google Scholar]
31. D. J. Tsiatsis, V. Mulligan, C. Avesand, S. Karnouskos and S. Boyle, “Real-world design constraints,” From Machine-to-Machine to the Internet of Things: Introduction to a New Age of Intelligence, pp. 225–231, Academic Press, 2014. https://doi.org/10.1016/B978-0-12-407684-6.00009-7 [Google Scholar] [CrossRef]
32. Y. Qin, Q. Z. Sheng, N. J. G. Falkner, S. Dustdar, H. Wang et al., “When things matter: A data-centric view of the internet of things,” 2014. [Google Scholar]
33. A. Karkouch, H. Mousannif, H. Al Moatassime and T. Noel, “Data quality in internet of things: A state-of-the-art survey,” J. Netw. Comput. Appl., vol. 73, pp. 57–81, 2016. [Google Scholar]
34. T. Banerjee and A. Sheth, “IoT quality control for data and application needs,” IEEE Intell. Syst., vol. 32, no. 2, pp. 68–73, 2017. [Google Scholar]
35. W. Tu, “Data-driven QoS and QoE management in smart cities: A tutorial study,” IEEE Commun. Mag., vol. 56, no. 12, pp. 126–133, 2019. [Google Scholar]
36. D. Pal, V. Vanijja, C. Arpnikanondt, X. Zhang and B. Papasratorn, “A quantitative approach for evaluating the quality of experience of smart-wearables from the quality of data and quality of information: An end user perspective,” IEEE Access, vol. 7, no. June, pp. 64266–64278, 2019. [Google Scholar]
37. J. Zhang, Y. Ma and D. Hong, “Research on data quality assessment of accuracy and quality control strategy for sensor networks,” J. Phys. Conf. Ser., vol. 1288, no. 1, pp. 012041, 2019. [Google Scholar]
38. A. Floris and L. Atzori, “Towards the evaluation of quality of experience of internet of things applications,” in 9th Int. Conf. Qual. Multimed. Exp., Erfurt, Germany, pp. 5, 2017. [Google Scholar]
39. A. A. Barakabitze, N. Barman, A. Ahmad, S. Zadtootaghaj, L. Sun et al., “QoE management of multimedia streaming services in future networks: A tutorial and survey,” IEEE Commun. Surv. Tutorials, vol. 22, no. 1, pp. 526–565, 2020. [Google Scholar]
40. D. Pal, T. Triyason, V. Varadarajan and X. Zhang, “Quality of experience evaluation of smart-wearables: A mathematical modelling approach,” in Proc.—2019 IEEE 35th Int. Conf. Data Eng. Work. ICDEW 2019, Macao, China, pp. 74–80, 2019. [Google Scholar]
41. Y. Ikeda, S. Kouno, A. Shiozu and K. Noritake, “A framework of scalable QoE modeling for application explosion in the internet of things,” in 2016 IEEE 3rd World Forum Internet Things, WF-IoT 2016, Reston, VA, USA, pp. 425–429, 2017. [Google Scholar]
42. C. Perera, A. Zaslavsky, P. Christen and D. Georgakopoulos, “Context aware computing for the internet of things: A survey,” IEEE Commun. Surv. Tutorials, vol. 16, no. 1, pp. 414–454, 2014. [Google Scholar]
43. Z. Sun, C. H. Liu, C. Bisdikian, J. W. Branch and B. Yang, “QoI-aware energy management in internet-of-things sensory environments,” Annu. IEEE Commun. Soc. Conf. Sensor, Mesh Ad Hoc Commun. Networks Work., vol. 1, pp. 19–27, 2012. [Google Scholar]
44. D. H. Shin, “Cross-platform users’ experiences toward designing interusable systems,” Int. J. Hum. Comput. Interact., vol. 32, no. 7, pp. 503–514, 2016. [Google Scholar]
45. A. Karaadi, L. Sun and I. H. Mkwawa, “Multimedia communications in internet of things QoT or QoE?” in Proc.—2017 IEEE Int. Conf. Internet Things, IEEE Green Comput. Commun. IEEE Cyber, Phys. Soc. Comput. IEEE Smart Data, iThings-GreenCom-CPSCom-SmartData 2017, Exeter, UK, vol. 2018-January, pp. 23–29, 2018. [Google Scholar]
46. Q. Wu, G. Ding, Y. Xu, S. Feng, Z. Du et al., “Cognitive internet of things: A new paradigm beyond connection,” IEEE Internet Things J., vol. 1, no. 2, pp. 129–143, 2014. [Google Scholar]
47. Y. Qin, Q. Z. Sheng, N. J. G. Falkner, S. Dustdar, H. Wang et al., “When things matter: A data-centric view of the internet of things,” ACM Journal Name, vol. V, no. N, pp. 1–35, 2014. [Google Scholar]
48. K. S. Jagarlamudi, A. Zaslavsky, S. W. Loke, A. Hassani and A. Medvedev, “Requirements, limitations and recommendations for enabling End-to-end quality of context-awareness in IoT middleware,” Sensors, vol. 22, no. 4, pp. 1632, 2022. [Google Scholar] [PubMed]
Cite This Article
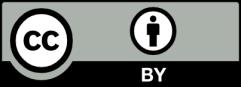