Open Access
ARTICLE
Incomplete Image Completion through GAN
1 School of Computer Science, Southwest Petroleum University, Chengdu, 610000, China
2 AECC Sichuan Gas Turbine Establishment, Mianyang, 621700, China
* Corresponding Author:Desheng Zheng. Email:
Journal of Quantum Computing 2021, 3(3), 119-126. https://doi.org/10.32604/jqc.2021.017250
Received 03 July 2021; Accepted 29 August 2021; Issue published 21 December 2021
Abstract
There are two difficult in the existing image restoration methods. One is that the method is difficult to repair the image with a large damaged, the other is the result of image completion is not good and the speed is slow. With the development and application of deep learning, the image repair algorithm based on generative adversarial networks can repair images by simulating the distribution of data. In the process of image completion, the first step is trained the generator to simulate data distribution and generate samples. Then a large number of falsified images are quickly generated using the generative adversarial network and search for the code of the closest damaged image. Finally, the generator generates missing content by using this code. On this basis, this paper combines the semantic loss function and the perceptual loss function. Experimental result show that the method successfully predicts the information of large areas missing in the image, and realizes the photorealism, producing clearer and more consistent results than previous methods.Keywords
Cite This Article
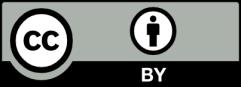
This work is licensed under a Creative Commons Attribution 4.0 International License , which permits unrestricted use, distribution, and reproduction in any medium, provided the original work is properly cited.