Open Access
ABSTRACT
Automatic Segmentation Methods Based on Machine Learning for Intracoronary Optical Coherence Tomography Image
1 School of Biological Science & Medical Engineering, Southeast University, Nanjing, 210096, China.
2 School of Mathematics, Southeast University, Nanjing, 210096, China.
3 Mathematical Sciences Department, Worcester Polytechnic Institute, Worcester, MA, 01609, USA.
4 Department of Medicine, Emory University School of Medicine, Atlanta, GA, 30307, USA.
5 Mallinckrodt Institute of Radiology, Washington University, St. Louis, MO, 63110, USA.
6 The Cardiovascular Research Foundation, Columbia University, New York, NY, 10022, USA.
7 The Wallace H. Coulter Department of Biomedical Engineering, Georgia Institute of Technology, Atlanta, GA, 30332, USA.
* Corresponding Author: Dalin Tang. Email: dtang@wpi.edu.
Molecular & Cellular Biomechanics 2019, 16(Suppl.1), 79-80. https://doi.org/10.32604/mcb.2019.05747
Abstract
Cardiovascular diseases are closely associated with sudden rupture of atherosclerotic plaques. Previous image modalities such as magnetic resonance imaging (MRI) and intravascular ultrasound (IVUS) were unable to identify vulnerable plaques due to their limited resolution. Optical coherence tomography (OCT) is an advanced intravascular imaging technique developed in recent years which has high resolution approximately 10 microns and could provide more accurate morphology of coronary plaque. In particular, it is now possible to identify plaques with fibrous cap thickness <65 μm, an accepted threshold value for vulnerable plaques. However, the current segmentation of OCT images are still performed manually by physicians and the process is time consuming. Automatic segmentation and recognition of vulnerable plaques through quantification of plaque components have great clinical significance for cardiovascular research. Two segmentation methods for intracoronary OCT image based on support vector machine (SVM) and convolutional neural network (CNN) were performed to detect the lumen borders and characterize the plaque component.In vivo IVUS and OCT coronary plaque data from 5 patients were acquired at Emory University with patient’s consent obtained. Seventy-seven matched IVUS and OCT slices with good image quality and medium to large lipid cores were selected for our segmentation study. Manual OCT segmentation was performed by experts and used as gold standard in the automatic segmentations. VH-IVUS was used as references and guide by the experts in the manual segmentation process. Three plaque component tissue classes were identified from OCT images in this work: lipid tissue (LT), fibrous tissue (FT) and background (BG). Procedures using two machine learning methods (CNN and SVM) were developed to segment OCT images, respectively. For CNN method, the U-Net architecture was selected due to its good performance in very different biomedical segmentation and very few annotated images. For SVM method, local binary patterns (LBPs), gray level co-occurrence matrices (GLCMs) which contains contrast, correlation, energy and homogeneity, entropy and mean value were calculated as features and assembled to feed SVM classifier. The accuracies of two segmentation methods were evaluated and compared using the OCT dataset. Segmentation accuracy is defined as the ratio of the number of pixels correctly classified over the total number of pixels.
The overall classification accuracy based CNN method reached 95.8%, and the accuracies for LT, FT and BG were 86.8%, 83.4%, and 98.2%, respectively. The overall classification accuracy based SVM was 71.9%, and per-class accuracy for LT, FT and BG was 75.4%, 78.3%, and 67.0%, respectively.
The two methods proposed can automatically detect the lumen borders and characterize the composition of the superficial plaque in OCT images and greatly reduce the time spent by doctors in segmenting and identifying plaques. CNN provided better segmentation accuracies compared to those achieved by SVM.
Keywords
Cite This Article
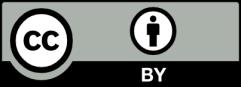