Open Access
ARTICLE
Terrorism Attack Classification Using Machine Learning: The Effectiveness of Using Textual Features Extracted from GTD Dataset
1 School of Computer Science and Technology, Wuhan University of Technology, Wuhan, 430070, China
2 LDDI Laboratory, Faculty of Science and Technology, University of Ahmed DRAIA, Adrar, 01000, Algeria
3 Information Systems Department, Faculty of Management, Comenius University, Bratislava, 82005, Slovakia
* Corresponding Author: Mohammed Abdalsalam. Email:
(This article belongs to the Special Issue: Advanced Machine Learning for Big Data Analytics in Natural Language Processing)
Computer Modeling in Engineering & Sciences 2024, 138(2), 1427-1467. https://doi.org/10.32604/cmes.2023.029911
Received 14 March 2023; Accepted 07 July 2023; Issue published 17 November 2023
Abstract
One of the biggest dangers to society today is terrorism, where attacks have become one of the most significant risks to international peace and national security. Big data, information analysis, and artificial intelligence (AI) have become the basis for making strategic decisions in many sensitive areas, such as fraud detection, risk management, medical diagnosis, and counter-terrorism. However, there is still a need to assess how terrorist attacks are related, initiated, and detected. For this purpose, we propose a novel framework for classifying and predicting terrorist attacks. The proposed framework posits that neglected text attributes included in the Global Terrorism Database (GTD) can influence the accuracy of the model’s classification of terrorist attacks, where each part of the data can provide vital information to enrich the ability of classifier learning. Each data point in a multiclass taxonomy has one or more tags attached to it, referred as “related tags.” We applied machine learning classifiers to classify terrorist attack incidents obtained from the GTD. A transformer-based technique called DistilBERT extracts and learns contextual features from text attributes to acquire more information from text data. The extracted contextual features are combined with the “key features” of the dataset and used to perform the final classification. The study explored different experimental setups with various classifiers to evaluate the model’s performance. The experimental results show that the proposed framework outperforms the latest techniques for classifying terrorist attacks with an accuracy of 98.7% using a combined feature set and extreme gradient boosting classifier.Keywords
Cite This Article
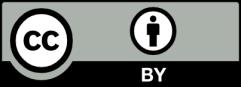