Open Access
ARTICLE
The Lambert-G Family: Properties, Inference, and Applications
1 Accounting Department, Ashur University, Baghdad, 10011, Iraq
2 Department of Statistics, Mathematics and Insurance, Benha University, Benha, 13511, Egypt
3 Department of Management Information Systems and Production Management, College of Business and Economics, Qassim University, Buraydah, 51452, Saudi Arabia
4 Department of Basic Sciences, Common First Year (CFY), King Saud University, Riyadh, 11362, Saudi Arabia
5 Department of Mathematics and Statistics, Osim Higher Institute of Administrative Science, Osim, 12961, Egypt
* Corresponding Authors: Ahmed Z. Afify. Email: ; Badr Alnssyan. Email:
(This article belongs to the Special Issue: Frontiers in Parametric Survival Models: Incorporating Trigonometric Baseline Distributions, Machine Learning, and Beyond)
Computer Modeling in Engineering & Sciences 2024, 140(1), 513-536. https://doi.org/10.32604/cmes.2024.046533
Received 05 October 2023; Accepted 15 January 2024; Issue published 16 April 2024
Abstract
This study proposes a new flexible family of distributions called the Lambert-G family. The Lambert family is very flexible and exhibits desirable properties. Its three-parameter special sub-models provide all significant monotonic and non-monotonic failure rates. A special sub-model of the Lambert family called the Lambert-Lomax (LL) distribution is investigated. General expressions for the LL statistical properties are established. Characterizations of the LL distribution are addressed mathematically based on its hazard function. The estimation of the LL parameters is discussed using six estimation methods. The performance of this estimation method is explored through simulation experiments. The usefulness and flexibility of the LL distribution are demonstrated empirically using two real-life data sets. The LL model better fits the exponentiated Lomax, inverse power Lomax, Lomax-Rayleigh, power Lomax, and Lomax distributions.Keywords
Many approaches have been suggested to propose new families of distributions or to generalize some of the classical distributions. These families and generalized distributions provide more flexibility in modeling real-life data in different applied fields. The most common feature of the new families and generalized distributions is represented by having one or more extra shape parameters. Hence, the statistical literature contains many families to generate new distributions by adding one or more shape parameters. Some examples include the Kumaraswamy-G by Cordeiro et al. [1], exponentiated T-X by Alzaghal et al. [2], Weibull-G by Bourguignon et al. [3], odd moment exponential-G by Haq et al. [4], Burr XII-G by Cordeiro et al. [5], generalized odd Burr III-G by Haq et al. [6], generalized odd half-logistic-G by Altun et al. [7], Marshall–Olkin alpha power by Nassar et al. [8], new exponential-X by Ahmad et al. [9], new extended heavy-tailed family by Aljohani et al. [10], and modified generalized-G by Shama et al. [11].
One of the most notable approaches to generating new distributions is constructed by using the Lambert-W (LW) function which is also known as the product logarithm function. This approach is discussed by Corless [12]. It is defined (for
The above equation contains only one real-valued solution. Recently, the LW function has been used in the distribution of prime numbers as discussed by Visser [13]. Goerg [14] adopted the LW function to introduce new families of distributions in the context of random variable transformations. Iriarte et al. [15] generated the Lambert-F class as an alternative family for positive data analysis.
This study introduces a new wider class based on the LW function called the Lambert-G (LG) family, in which the baseline distribution is a continuous distribution with positive support. The proposed approach applies a transformation to a baseline cumulative distribution function (cdf), as illustrated in Definition 1. The newly generated cdf of the LG family, with two extra shape parameters, has the quantile function (qf), which is expressed in a closed form in terms of the LW function; hence, the proposed generator is called the LG family.
The LG family has some desirable properties and it can be justified as follows. (i) The three-parameter special sub-models of the LG family are capable of modeling all important hazard rate (hr) shapes including increasing, decreasing, unimodal, J-shape, reversed J-shape, bathtub, and modified-bathtub failure rates; (ii) Moreover, the densities of its sub-models accommodate reversed J shaped, right-skewed, symmetric, left-skewed, decreasing-increasing-decreasing densities; (iii) The LG special sub-models generalize some well-known distributions in the distribution theory literature such as the modified Weibull model [16]; (iv) The LG special models provide better fit than other generalized models under the same baseline distribution as shown in case of the Lambert-Lomax (LL) model.
The paper is organized in the following sections. In Section 2, the LG family is presented. In Section 3, we provide three special sub-models of the LG family. The properties of the LL distribution along with its analytical shapes are explored in Section 4. In Section 5, the parameters of the LL distribution are estimated via six classical estimation methods. Section 6 presents simulation results to address the behavior of different estimators. To show the empirical importance of the LL distribution, two real-life data sets are analyzed in Section 7. Final remarks are given in Section 8.
Definition 1. A random variable X is said to follow the LG family, denoted by
where
The probability density function (pdf) corresponding to Eq. (2) reduces to
where
The hrf of the LG family becomes
According to Eq. (4), the hrf of the LG family has a flexible property because it depends on the value of the extra parameter
Eq. (5) is called the reduced Lambert-G (RLG) family.
The pdf and hrf of the RLG are given, respectively, by
and
3 Special Models of the LG Family
In this section, we provide three specific models of the LG family. These special distributions provide modified flexible forms of some standard distributions namely the exponential, Pareto, and Lomax distributions. The special sub-models of the LG family are called the Lambert-exponential (LE), Lambert-Pareto (LP), and LL distributions. These special models are capable of modeling all important hrf shapes including increasing, decreasing, unimodal, J-shape, reversed J-shape, bathtub, and modified bathtub failure rates. Moreover, the densities of these sub-models can also provide reversed J-shaped, right-skewed, symmetric, left-skewed, decreasing-increasing-decreasing densities.
The LE cdf follows from Eq. (2) by setting
where
The corresponding pdf and hrf of the LE distribution take the forms
and
The hrf shapes of the LE distribution depend only on the value of
Consider the sf of the Pareto distribution
where
and
The shape of the LP hrf depends on the values of
Figure 1: Possible shapes for the density and hazard functions of the LE and LP distributions
By taking the sf of the Lomax distribution,
where
The corresponding pdf and hrf are
and
The Lomax distribution follows as a special case of the LL distribution with
Figure 2: Possible shapes for the pdf of the LL distribution
Figure 3: Possible shapes for the hrf of the LL distribution
4 Properties of the LL Distribution
In this section, we provide some basic statistical properties of the LL distribution.
4.1 Behavior of the Density and Hazard Rate Functions
The pdf limits of the LL distribution as
Theorem 4.1. The graph of the pdf of the LL distribution is log-concave if
Proof. Setting
Differentiating twice concerning
Note that
The hrf limits of the LL distribution as
Theorem 4.2. The hrf of the LL distribution is
• Increasing for
• Decreasing for
• Unimodal for
• Bathtub for
• Decreasing-increasing-decreasing for
Proof. From Eq. (16), we have
The derivative of
where
where
Clearly, both the hrf of the LL distribution and
Case 1: For
Case 2: If
Case 3: If
Case 4: If
To discuss other cases, we have to get two critical values of
and
Case 5: For
Case 6: The function
Case 7: For
Theorem 4.3. The
Proof. The
Let
By using the binomial and series expansions, the
Let
Let
Finally, we have
which completes the proof.
As well as the measures of skewness, kurtosis, and asymmetry of the LL distribution are obtained by the following relations:
Table 1 provides some important LL measures for various parametric values. The numerical values show that the LL distribution can be right skewed for different values of
The qf of the LL distribution follows, by inverting its cdf (15), as
where
and
The order statistic for the LL distribution will be discussed in this section. It will also be useful to derive the pdf of the
We have
and
Substituting (21) and (22) in (20), one can write
Hence, the largest order statistic density follows as
The smallest order statistic pdf reduces to
5 Estimation of the LL Parameters
In this section, different techniques are used to estimate the LL parameters.
The LL parameters are estimated by the maximum likelihood (ML). Consider a random sample from the LL distribution denoted by
where
The ML estimators (MLE) of
and
5.2 Least-Squares and Weighted Least-Squares
The least squares (LS) and the weighted LS (WLS) methods are introduced by Swain et al. [17]. The LS estimators (LSE) of the LL parameters are calculated by minimizing the following function concerning
where
and
where
and
The WLS estimators (WLSE) of the LL parameters follow by minimizing the function
where
Also, these estimators are determined by solving the following non-linear system:
where
The Cramér–von Mises (CM) method was introduced by Choi et al. [18] depending on the CM statistics (Boos [19]). Then, the CM estimators (CME) of the LL parameters minimize the following function:
with respect to
where
5.4 Anderson–Darling and Right-Tail Anderson–Darling
Depending on the Anderson–Darling (AD) statistic, the AD method was proposed by Anderson et al. [20] and [21]. Hence, the AD estimators (ADE) of the LL parameters are calculated by minimizing the function
Therefore, the ADE is also obtained by solving the following non-linear system:
where
Luceno [22] applied some motivations to the AD statistic called the right-tail AD (RAD) statistic. Hence, to obtain the RAD estimators (RADE) of the LL parameters, we minimize the following function:
Additionally, the RADE is obtained by solving the non-linear system
where
All above mentioned non-linear systems of equations have no exact solutions, so the optim and nlminb functions in R software can be adopted for this purpose.
This section presents numerical simulation results to explore the efficiency and performance of different estimators for the LL parameters. The following algorithm is adopted to evaluate different estimators:
1. Set different initial values of sample size
2. Generate several random samples of size
3. The outcomes in the previous step are used to calculate the parameter estimates,
4. The three above steps are repeated 6,000 times.
5. Based on
and
From the LL distribution 6,000 samples are generated for
The AB and RMSE of the MLE, CME, LSE, ADE, WLSE, and RADE are presented in Tables 2–4. Moreover, the partial and overall ranks of the estimators are calculated in Table 5. From the values in these tables, one can note that
1. All estimates show the property of consistency, i.e., the AB and RMSE decrease as sample size increases for all parametric combinations.
2. According to AB and RMSE, the ordering of performance of estimators (from best to worst) for all parameters is the MLE, ADE, WLSE, RADE, LSE and CME.
In this section, we analyze two real-life data sets to demonstrate the performance of the LL distribution in practice. Two real-life data sets are fitted to compare the proposed LL model with other five known competitors, namely:
1. The Exponentiated Lomax (EL) distribution [23] with pdf
2. The Poisson–Lomax (PoL) distribution [24] with pdf
3. The Lomax-Rayleigh (LR) distribution [25] with pdf
4. The power Lomax (PL) distribution [26] with pdf
5. The Lomax (L) distribution [27] with pdf
The first data represent 63 service times (thousand hours) of aircraft windshield (unit in thousand hours) as reported in Murthy et al. [28]. The data are as follows:
The second data represents 63 strengths of 1.5 cm glass fibers which are measured by the National Physical Laboratory, in England as reported in Smith et al. [29]. The data are as follows:
Table 6 provides a brief summary for both data sets.
The parameters of the fitted distributions are estimated using the ML method and some discrimination measures are calculated to explore the efficiency of the competing distributions. These measures include the Akaike information criterion (AIC), Bayesian IC (BIC), corrected AIC (CAIC), Hannan–Quinn IC (HQIC), and –ℓ, where ℓ is the maximized log-likelihood. Additionally, goodness-of-fit statistics such as Anderson–Darling (An), Cramér–von Mises (Cr), and Kolmogorov–Smirnov (K-S) with its corresponding p-value (K-S p-value) are also calculated. More details about the goodness-of-fit statistics can be explored by Shama et al. [30].
Tables 7 and 8 present the MLEs of the parameters of the fitted distributions along with their discrimination measures for both data sets, respectively. Based on Tables 7 and 8, we conclude that the LL distribution is the best model as compared to other Lomax extensions. Tables 9 and 10 provide the values of goodness-of-fit measures of the LL model and other models for the two data sets. These results also indicate that the LL model provides a better fit to aircraft windshield and glass fibers data as compared to other Lomax models.
The probability-probability (P-P) plots of the fitted distributions for both data sets are provided in Figs. 4 and 5. The fitted density, cdf, sf, and P-P plots of the LL distribution for both data sets are displayed in Figs. 6 and 7. The plots support the results in Tables 7–10 and illustrate that the LL distribution provides a better approximation between the theoretical and empirical curves. Furthermore, Figs. 8 and 9 present the histograms of aircraft windshield and glass fibers data along with the fitted densities of the LL model and other studied distributions. All plots provide evidence that the LL distribution is the most well-adjusted model for aircraft windshield and glass fibers data.
Figure 4: P-P plots for aircraft windshield data
Figure 5: P-P plots for glass fibers data
Figure 6: Plots of the fitted functions of the LL model for aircraft windshield data
Figure 7: Plots of the fitted functions of the LL model for glass fibers data
Figure 8: Histogram of aircraft windshield data along with the estimated densities
Figure 9: Histogram of glass fibers data along with the estimated densities
This study introduces a new flexible family called the LG family. Its special sub-models can represent various shapes of aging failure criteria, including monotonic and non-monotonic failure rates. The densities of the sub-models of the LG family can be reversed-J shaped, right-skewed, symmetric, left-skewed, decreasing-increasing-decreasing densities. One of its special models, namely the LL, is studied in detail. The failure rate shapes of the LL distribution are derived and proved mathematically. In addition, various statistical properties of the LL distribution are investigated. Six estimation methods are employed to estimate the LL parameters, and their performance is explored via simulation results. The numerical experiments illustrate the accuracy of the maximum likelihood; hence, they are recommended for estimating the LL parameters. Two real-life datasets are analyzed, indicating that the LL distribution can provide a better fit for modeling actual data compared to some competing Lomax models.
The perspectives of this study can include the development of a bivariate LL distribution and the construction of a discrete version of the LL model.
Acknowledgement: The authors would like to thank the editor and the referees for valuable comments which greatly improved the paper.
Funding Statement: The authors received no specific funding for this study.
Author Contributions: The authors confirm contribution to the paper as follows: study conception and design: J.N.A.A., A.Z.A., B.A., M.S.S.; data collection: A.Z.A., M.S.S.; analysis and interpretation of results: J.N.A.A., A.Z.A., B.A., M.S.S.; draft manuscript preparation: J.N.A.A. All authors reviewed the results and approved the final version of the manuscript.
Availability of Data and Materials: This work is mainly a methodological development and has been applied on secondary data which are provided in the manuscript.
Conflicts of Interest: The authors declare that they have no conflicts of interest to report regarding the present study.
References
1. Cordeiro, G. M., de Castro, M. (2011). A new family of generalized distributions. Journal of Statistical Computation and Simulation, 81, 883–893. [Google Scholar]
2. Alzaghal, A., Lee, C., Famoye, F. (2013). Exponentiated T-X family of distributions with some applications. International Journal of Probability and Statistics, 2, 31–49. [Google Scholar]
3. Bourguignon, M., Silva, R. B., Cordeiro, G. M. (2014). The Weibull-G family of probability distributions. Journal of Data Science, 12, 53–68. [Google Scholar]
4. Haq, M. A. U., Handique, L., Chakraborty, S. (2018). The odd moment exponential family of distributions: Its properties and applications. International Journal of Applied Mathematics and Statistics, 57, 47–62. [Google Scholar]
5. Cordeiro, G. M., Yousof, H. M., Ramires, T. G., Ortega, E. M. (2018). The Burr XII system of densities: Properties, regression model and applications. Journal of Statistical Computation and Simulation, 88, 432–456. [Google Scholar]
6. Haq, M. A. U., Elgarhy, M., Hashmi, S. (2019). The generalized odd Burr III family of distributions: Properties, applications and characterizations. Journal of Taibah University for Science, 13, 961–971. [Google Scholar]
7. Altun, E., Alizadeh, M., Afify, A. Z., Ozel, G. (2019). The generalized odd half-logistic family of distributions with regression models. International Journal of Statistics & Economics, 20, 88–110. [Google Scholar]
8. Nassar, M., Kumar, D., Dey, S., Cordeiro, G. M., Afify, A. Z. (2019). The Marshall-Olkin alpha power family of distributions with applications. Journal of Computational and Applied Mathematics, 351, 41–53. [Google Scholar]
9. Ahmad, Z., Mahmoudi, E., Roozegar, R., Alizadeh, M., Afify, A. Z. (2021). A new exponential-X family: Modeling extreme value data in the finance sector. Mathematical Problems in Engineering, 2021, 1–14. [Google Scholar]
10. Aljohani, H. M., Bandar, S. A., Al-Mofleh, H., Ahmad, Z., El-Morshedy, M. et al. (2022). A new asymmetric extended family: Properties and estimation methods with actuarial applications. PLoS One, 17, e0275001. [Google Scholar] [PubMed]
11. Shama, M. S., El Ktaibi, F., Al Abbasi, J. N., Chesneau, C., Afify, A. Z. (2023). Complete study of an original power-exponential transformation approach for generalizing probability distributions. Axioms, 12, 67. [Google Scholar]
12. Corless, R. M., Gonnet, G. H., Hare, D. E. G., Jeffrey, D. J., Knuth, D. E. (1996). On the Lambert W function. Advances in Computational Mathematics, 5, 329–359. [Google Scholar]
13. Visser, M. (2018). Primes and the Lambert W function. Mathematics, 6, 56. [Google Scholar]
14. Goerg, G. M. (2011). Lambert W random variables—A new family of generalized skewed distributions with applications to risk estimation. Annals of Applied Statistics, 5, 2197–2230. [Google Scholar]
15. Iriarte, Y. A., de Castro, M., Gómez, H. W. (2020). The Lambert-F distributions class: An alternative family for positive data analysis. Mathematics, 8, 1398. [Google Scholar]
16. Lai, C., Xie, M., Murthy, D. (2003). A modified Weibull distribution. IEEE Transactions on Reliability, 52, 7–33. [Google Scholar]
17. Swain, J., Venkatraman, S., Wilson, J. R. (1988). Least squares estimation of distribution function in Johnsons translation system. Journal of Statistical Computation and Simulation, 29, 271–297. [Google Scholar]
18. Choi, K., Bulgren, W. (1968). An estimation procedure for mixtures of distributions. Journal of the Royal Statistical Society Series B:Methodological, 30, 444–460. [Google Scholar]
19. Boos, D. D. (1981). Minimum distance estimators for location and goodness of fit. Journal of the American Statistical Association, 76, 663–670. [Google Scholar]
20. Anderson, T. W., Darling, D. A. (1952). Asymptotic theory of certain “goodness of fit” criteria based on stochastic processes. The Annals of Mathematical Statistics, 23, 193–212. [Google Scholar]
21. Anderson, T. W., Darling, D. A. (1954). A test of goodness of fit. Journal of the American Statistical Association, 49, 765–769. [Google Scholar]
22. Luceno, A. (2006). Fitting the generalized Pareto distribution to data using maximum goodness-of-fit estimators. Computational Statistics & Data Analysis, 51, 904–917. [Google Scholar]
23. Gupta, R. C., Gupta, P. I., Gupta, R. D. (1998). Modeling failure time data by Lehmann alternatives. Communications in Statistics-Theory and Methods, 27, 887–904. [Google Scholar]
24. Al-Zahrani, B., Sagor, H. (2014). The Poisson-Lomax distribution. Revista Colombiana de Estadística, 37, 225–245. [Google Scholar]
25. Venegas, O., Iriarte, Y. A., Astorga, J. M., Borger, A., Bolfarine, H. et al. (2019). Lomax-Rayleigh distribution with an application. Applied Mathematics & Information Sciences, 13, 741–748. [Google Scholar]
26. Rady, E. A., Hassanein, W. A., Elhaddad, T. A. (2016). The power Lomax distribution with an application to bladder cancer data. Springerplus, 5, 1838. [Google Scholar] [PubMed]
27. Lomax, K. S. (1954). Business failures: Another example of the analysis of failure data. Journal of the American Statistical Association, 49, 847–852. [Google Scholar]
28. Murthy, D. N. P., Xie, M., Jiang, R. (2004). Weibull models, wiley series in probability and statistics. New York: John Wiley and Sons. [Google Scholar]
29. Smith, R., Naylor, J. (1987). A comparison of maximum likelihood and Bayesian estimators for the three-parameter Weibull distribution. Applied Statistics, 36, 358–369. [Google Scholar]
30. Shama, M. S., Dey, S., Altun, E., Afify, A. Z. (2022). The gamma-Gompertz distribution: Theory and applications. Mathematics and Computers in Simulation, 193, 689–712. [Google Scholar]
Cite This Article
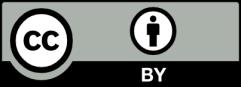