Open Access
ARTICLE
Diagnostic and classification value of immune-related lncRNAs in dilated cardiomyopathy
1 Information Center, West China Hospital, Sichuan University, Chengdu, 610041, China
2 Laboratory of Cardiovascular Diseases, Regenerative Medicine Research Center, West China Hospital, Sichuan University, Chengdu, 610041, China
3 Health Management Center, General Practice Medical Center, West China Hospital, Sichuan University, Chengdu, 610041, China
4 Department of Cardiology, West China Hospital, Sichuan University, Chengdu, 610041, China
* Corresponding Authors: Xiaojing Liu, ; Junteng Zhou,
(This article belongs to the Special Issue: Subcellular Organelles and Cellular Molecules: Localization, Detection, Prediction, and Diseases)
BIOCELL 2023, 47(11), 2517-2533. https://doi.org/10.32604/biocell.2023.043864
Received 14 July 2023; Accepted 08 September 2023; Issue published 27 November 2023
Abstract
Background: Various physiological mechanisms are linked to dilated cardiomyopathy (DCM) development, including oxidative stress, immune irregularities, inflammation, fibrosis, and genetic changes. However, precise molecular drivers of DCM, especially regarding abnormal immune responses, remain unclear. This study investigates immune-related long non-coding RNAs (lncRNAs) in DCM’s diagnostic and therapeutic potential. Methods: GSE141910, GSE135055, and GSE165303 datasets were acquired from the GEO database. LASSO, SVM-RFE, and random forest algorithms identified DCM-associated immune-related lncRNAs. Diagnostic capabilities were assessed by Nomogram and receiver operating characteristic (ROC) curves. Multivariate linear regression explored lncRNA correlations with ejection fraction. Single-sample gene set enrichment analysis (ssGSEA) gauged immune cell infiltration/functions. Functional enrichment analyses were performed using Gene set variation analysis (GSVA), gene ontology (GO), and the Kyoto Encyclopedia of Genes and Genomes (KEGG). Consensus clustering categorized DCM cases. Results: Ten immune-related lncRNAs emerged: C10orf71-AS1, FHAD1-AS1, SCIRT, FNDC1-AS1, MELTFAS1, LOC101928834, GDNF-AS1, DCXR-DT, C3orf36, and LOC107985323. These lncRNAs, tied to immunomodulation, showed promising DCM diagnostic accuracy. Adjusted for confounders, they independently correlated with ejection fraction. Using lncRNA expression, DCM patients were grouped into subtypes. Subtype C1 displayed a higher level of immune cell infiltration and immune checkpoint expression compared to subtype C2, emphasizing the variations in the immune microenvironment. Conclusion: This study identifies ten immune-related lncRNAs for further exploration in DCM diagnosis and subtyping. Based on expression patterns, we propose two potential DCM subtypes. Notably, findings are preliminary and hypothesis-generating, demanding validation and further investigation. This research provides insights into DCM diagnosis and classification.Graphic Abstract
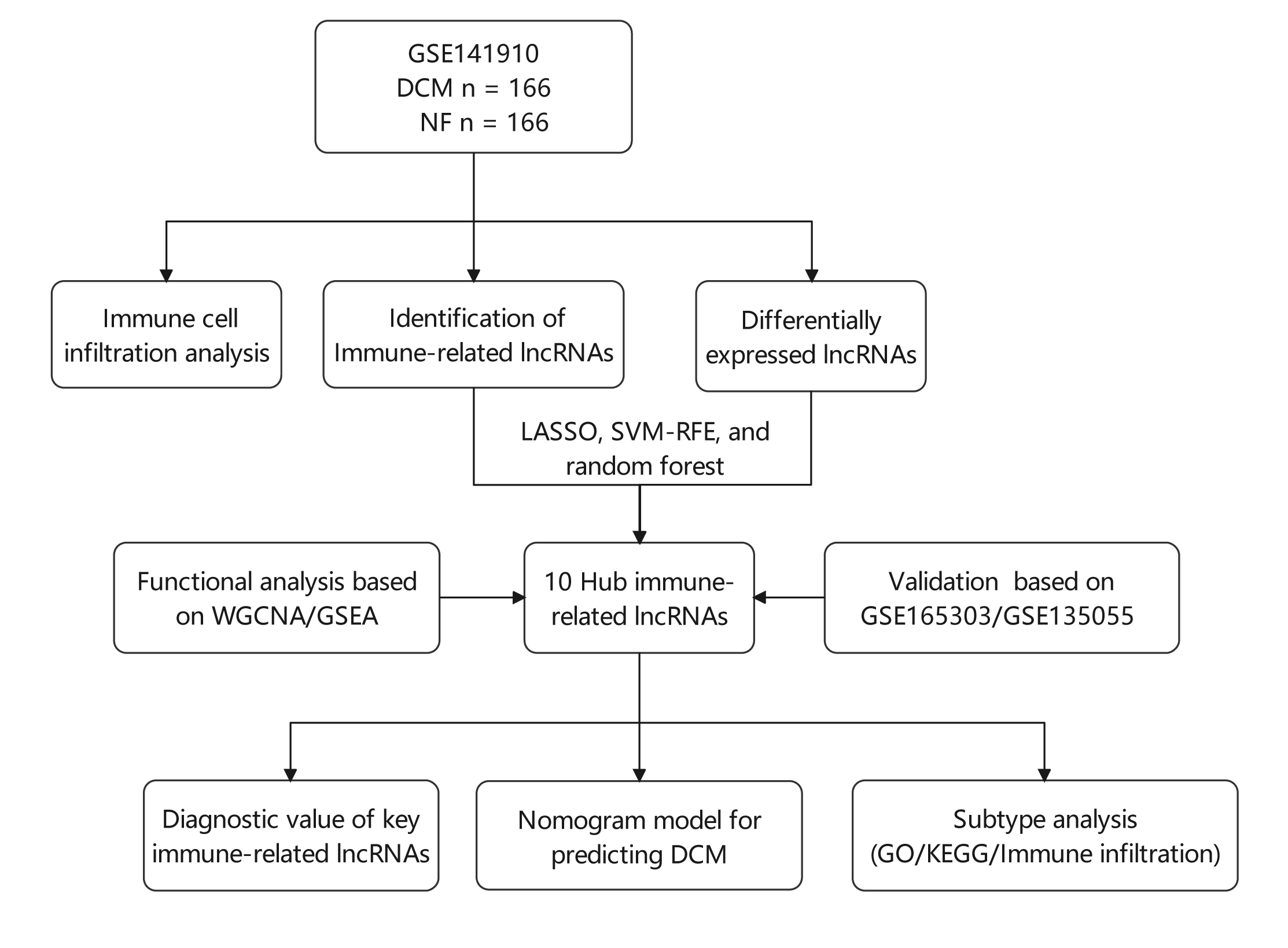
Keywords
Supplementary Material
Supplementary Material FileDilated cardiomyopathy (DCM) characterized by impaired ventricular systolic and dilated function is the main cause of heart failure (Weintraub et al., 2017). The enlargement of the heart is characterized by significant dilation of the left ventricle and abnormal myocardial contraction, which eventually leads to the disturbance of blood pumping (Du et al., 2018). Myocardial remodeling, which depends on changes in myocardial structure and composition, is considered to be an important reason for the development of DCM (Weintraub et al., 2017). It has been previously reported that local necrosis, fibrosis, and calcification often occur in the myocardial tissue of DCM (Reichart et al., 2019; Lakdawala et al., 2013). DCM formation is a lengthy and intricate procedure, presenting a crucial chance for the early detection and treatment of DCM at the molecular level. A variety of techniques, including electrocardiogram, echocardiography, and magnetic resonance imaging, have become diagnostic strategies for DCM (Ampong, 2022). In addition, as a biomarker, B-type natriuretic peptide has been widely used in the early diagnosis of DCM (Harding et al., 2023). The advancement of sequencing technology has led to the utilization of diverse methods like genomics, proteomics, and metabonomics to identify novel biomarkers for diagnosing and predicting DCM (Raghow, 2016; Portokallidou et al., 2023). Studies have shown that circular RNA, long noncoding RNA (lncRNA) and microRNA are significantly differentially expressed in DCM and can be used as noninvasive biomarkers for the diagnosis of DCM (Costa et al., 2021; Miyamoto et al., 2015; Li et al., 2018). Although DCM can be caused by pathological processes caused by a variety of environmental factors (McDonagh et al., 2021), approximately 40% of DCM is determined by genes (Hershberger et al., 2013). These genes encode ion channels, sarcomeres, the nuclear membrane and the cytoskeleton (Weintraub et al., 2017). Thus, the formation mechanism of DCM is very complex. Therefore, at the level of cell function, it is very important to find biomarkers that play an important role in the diagnosis of DCM.
Significantly, immune dysfunction plays a crucial part in the pathological progression of DCM (Harding et al., 2023). Cardiac pathological tissue examination often reveals the presence of immune cell infiltration (Mahon et al., 2002). Studies have shown that autoantibodies produced by immune cells promote the development of heart disease, including DCM (Zhao and Fu, 2018), and DCM can be improved by using immunoadsorption therapy that removes autoantibodies from plasma (Zheng et al., 2022; Felix et al., 2015). In addition, in preclinical experimental studies, innate immunity is considered to be an important reason for increasing susceptibility to DCM (Elamm et al., 2012). The above results emphasize the important role of immune regulation in DCM. However, the significance of molecular targets involved in immune regulation in the diagnosis of DCM has not been fully elucidated. As a kind of RNA, lncRNA, which lacks the ability of protein-coding, can participate in various pathophysiological processes through epigenetic regulation and other ways (Nojima and Proudfoot, 2022). Previous studies have analyzed the key role of lncRNAs in the process of immune regulation (Robinson et al., 2020). Importantly, lncRNAs are also involved in the development of DCM. For example, in the DCM mouse model, lncRNA ZNF593-AS can improve cardiac systolic dysfunction by regulating calcium homeostasis (Fan et al., 2021). LncRNA ENST00000507296 can be used as a prognostic index for patients with DCM (Zhang et al., 2019). Nevertheless, the scarcity of compelling evidence regarding the potential impact of lncRNAs on DCM through immune response regulation remains. A recent study based on sex differences showed that immune-related competitive endogenous RNA may provide a theoretical basis for the pathogenesis of DCM (Liu et al., 2022). This study suggests that immune-related lncRNA signals may contribute to the diagnosis of DCM.
The type and abundance of gene expression in tissues under specific conditions can reflect the characteristics of a disease (Long et al., 2016). Therefore, in this study, we chose to analyze three DCM datasets: GSE141910, GSE135055, and GSE165303, which have been previously utilized in other studies. Zhang et al. (2021) confirmed the STAT3-related pathway and CD163+LYVE1+ macrophages as potential key pathways and immune cell subtypes in HCM using the GSE36961 and GSE141910 datasets. Bian et al. (2022) identified key modules and important genes associated with heart failure through the analysis of the GSE135055 and GSE123976 datasets. They pinpointed a module and two genes, CUX1 and ASB1, which might play crucial roles in heart failure (Bian et al., 2022). The GSE165303 dataset constructed by Feng et al. (2022) offered fresh insights into the chromatin topology underlying the pathogenesis of DCM (Feng et al., 2022). However, none of these studies focussed on immune-related lncRNAs analysis in DCM. To diagnose DCM, we utilized three machine-learning techniques: LASSO, random forest, and SVM-RFE. These algorithms were employed to identify the distinctive genes between DCM and immune-related lncRNAs. Furthermore, we put forward a novel form of DCM that relies on the manifestation of lncRNAs.
Acquiring data and quantifying expression profiles of lncRNAs
To obtain sequencing data and lncRNA expression profiles, we downloaded the raw data for GSE141910, GSE135055, and GSE165303 from the GEO database (https://www.ncbi.nlm.nih.gov/geo/). Specifically, GSE141910 includes myocardial tissue samples from 166 normal individuals and 166 patients with dilated cardiomyopathy (Flam et al., 2022). The clinical information within the GEO dataset originates from the website (https://github.com/mpmorley/MAGNet/blob/master/phenoData.csv). The origin of DCM was determined by analyzing medical records and conducting histopathological examinations. For a comprehensive understanding of recruiting methods and diagnostic criteria, refer to previously published research (Liu et al., 2015a). GSE135055 includes myocardial tissue samples from 9 normal individuals and 18 patients with dilated cardiomyopathy (Hua et al., 2020), while GSE165303 encompasses a comprehensive collection of RNA sequencing data obtained from 50 DCM samples, as well as 51 samples of non-failing biobanked heart tissues (Feng et al., 2022). To process the raw data, we performed quality control and trimming of the FASTQ files using TrimGalore (http://www.bioinformatics.babraham.ac.uk/projects/trim_galore) to remove low-quality sequencing data and adapter sequences. After obtaining clean reads, we used HISAT2 (http://daehwankimlab.github.io/hisat2) to align them to the GRCh38 reference genome. In the end, we employed StringTie (http//ccb.jhu.edu/software/stringtie/) for quantifying the expression levels of both lncRNAs and mRNAs using the alignment outcomes. By adhering to these procedures, we successfully acquired expression data of excellent quality for both mRNAs and lncRNAs, which can be utilized in subsequent studies.
Identification of immune-related lncRNAs
First, we obtained the mRNA and lncRNA expression for all samples and performed differential expression analysis using edgeR software. We filtered differentially expressed lncRNAs based on the criteria of logFC absolute value greater than 0.5 and FDR (p adj) <0.05. Next, we calculated the correlation between lncRNAs and mRNAs based on their expression profiles. We ranked all mRNAs based on their correlation coefficients with a specific lncRNA and used the GSEA algorithm to determine whether immune-related genes were enriched in the resulting gene sets. Based on the p-value and ES score from the GSEA, we used the immu. LncRNA package to calculate a lncRES score for each lncRNA-gene set pair (Li et al., 2020a), where ES represents the enrichment score of the lncRNA in the immune pathway gene set. Finally, we identified immune-related lncRNAs by selecting those with an absolute lncRES score greater than 0.995 and FDR less than 0.05 in dilated cardiomyopathy. Next, we obtained the differentially expressed immune-related lncRNAs by intersecting the sets of differentially expressed lncRNAs and immune-related lncRNAs. By utilizing this method, we were able to discover lncRNAs that are strongly associated with immune pathways and hold promising clinical implications for the diagnosis and treatment of dilated cardiomyopathy.
Selection of hub immune-related lncRNAs
Three machine learning algorithms, LASSO, Random Forest, and SVM-RFE, were utilized to screen immune-related lncRNAs in dilated cardiomyopathy. Lasso regression, a linear regression model, was implemented with 10-fold cross-validation using the glmnet package to shrink the coefficients of unimportant variables to zero, thus selecting the most significant variables (Engebretsen and Bohlin, 2019). The Random Forest algorithm was employed to eliminate feature redundancy, select the optimal feature combinations, and reduce feature dimensions to identify key lncRNAs with a relative importance greater than 1 (Ellis et al., 2014). SVM-RFE ranked each feature by score after training on model samples, removed the feature with the smallest score, trained the model again with the remaining features, and iterated until the requisite number of features were selected (Sanz et al., 2018). By taking the intersection of critical variables determined by the three machine learning algorithms, key immune-related lncRNAs were identified.
Single-sample GSEA was carried out based on mRNA expression profiles to evaluate immune cell infiltration and function in each sample, thereby showcasing differences in immune infiltration between normal and dilated cardiomyopathy tissues, as well as the correlation between key immune-related lncRNAs and immune infiltration.
A predictive model for dilated cardiomyopathy was constructed using the rms package based on key immune-related lncRNAs identified from the GSE141910 dataset. A nomogram plot was created, and the area under the ROC curve (discrimination) was calculated, along with a calibration curve (calibration accuracy, U test). External validation of the model was performed using the GSE135055 dataset.
Weighted gene co-expression network analysis (WGCNA)
WGCNA was performed on immune-related lncRNA and gene expression profiles using the “WGCNA” package. To obtain a scale-free network, a soft threshold of 11 was chosen to construct the adjacency matrix. Module clustering was carried out using the dynamic cut tree method. To identify the most significant module, downstream analysis selected the module that had the strongest correlation with immune-related lncRNAs. The methods of the WGCNA have previously been described in detail (Zhou et al., 2023).
The ConsensusClusterPlus package was utilized to conduct consensus clustering analysis on individuals with dilated cardiomyopathy and normal populations, employing expression profiling of 10 immune-related lncRNAs. The k-means algorithm was used to divide each subsample into a maximum of k (max k = 9) clusters, based on the Euclidean distance. By utilizing the plot of the consensus matrix, the plot of the consensus cumulative distribution function (CDF), and the tracking plot, the ideal number of clusters was determined. Principal component analysis (PCA) was utilized to define the differences between the two subtypes.
Functional enrichment analysis
The GO and KEGG pathway enrichment analyses were conducted using Metascape (http//metascape.org). GSEA was performed on immune-associated lncRNA activities by correlating them with mRNA in DCM, using a ranking system. The GSVA package was used to analyze the functional variances among subtypes, utilizing GO and KEGG datasets from MSigDB. Additionally, the limma package was employed to calculate the disparities in GSVA scores between the subtypes.
Continuous variables that conformed to a normal distribution are expressed as the mean ± standard deviation, while nonnormally distributed continuous variables are expressed as the median (interquartile range). Categorical variables were expressed as numbers (percentages). Differences between groups in continuous variables were calculated using t-tests or Wilcoxon tests, and differences in categorical variables were calculated using chi-square tests. ROC analysis was used to evaluate the predictive accuracy of continuous variables for dilated cardiomyopathy. Pearson correlation analysis was used to assess the correlation between lncRNA and left ventricular ejection fraction. Both univariate and multivariate linear regression analyses were conducted. A p-value below 0.05 was deemed to be statistically significant. R version 4.1.3 was utilized for all analyses.
Patient clinical information is outlined below and consolidated in Table 1. The clinical characteristics of 332 participants in GSE141910 were obtained from an article published by Flam et al. (2022). The results revealed distinct clinical profiles between the two study groups. Among the 332 participants, 166 were assigned to the NF group and an equal number to the DCM group. Notably, the DCM group exhibited a younger age (52.07 ± 10.58 years) compared to the NF group (55.92 ± 13.96 years, p = 0.005), and a higher proportion of males (60.24% vs. 46.39%, p = 0.011). The racial distribution also significantly differed, with a larger representation of African Americans in the DCM group (46.39% vs. 26.51%, p < 0.001). While BMI did not differ significantly, heart weight (514.83 ± 138.84 g vs. 424.57 ± 115.23 g, p < 0.001) and left ventricular mass (330.04 ± 85.87 g vs. 229.82 ± 69.09 g, p < 0.001) were notably higher in the DCM group. Furthermore, the DCM group showed significantly reduced left ventricular ejection fraction (0.15, 25th–75th percentile: 0.10–0.20) compared to the NF group (0.55, 25th–75th percentile: 0.50–0.65, p < 0.001). A higher prevalence of atrial fibrillation (40.36% vs. 10.24%, p < 0.001) and ventricular tachycardia/ventricular fibrillation (42.77% vs. 0.60%, p < 0.001) was also observed in the DCM group. The prevalence of hypertension was significantly higher in NF individuals compared to DCN patients (p = 0.015), with 61.45% of NF and 46.99% of DCM patients having hypertension., while diabetes prevalence did not significantly differ between groups. This comprehensive analysis underscores the substantial differences in demographics, clinical measurements, and cardiac function between the two groups, elucidating the distinctive profiles of DCM and NF individuals in the cohort.
Discovery of immune-related lncRNAs in DCM
We first analyzed RNA-seq data in dilated cardiomyopathy (DCM) and normal human myocardial tissue using a single-sample gene set enrichment analysis (ssGSEA) to calculate immune cell infiltration scores. As shown in Fig. 1A, compared to the normal myocardial tissue, DCM exhibited a significant increase in activated B cells, activated CD8+ T cells, CD56 dim natural killer cells, effector memory CD8+ T cells, gamma delta T cells, immature B cells, immature dendritic cells, memory B cells, monocytes, natural killer cells, and type 1 T helper cells. In contrast, DCM exhibited a notable reduction in activated dendritic cells, CD56 bright NK cells, central memory CD8+ T cells, eosinophils, macrophages, myeloid-derived suppressor cells, NK T cells, Tregs, Tfh cells, and Th17 cells. To explore immune-related lncRNAs in dilated cardiomyopathy (DCM), we adopted a computational framework based on a collection of samples with matched data on lncRNA and mRNA expression. We identified a total of 3196 lncRNA-gene set pairs across 17 immune-related gene set categories and 681 lncRNAs associated with immunity in DCM across 16 categories (Suppl. Table S1) (Fig. 1B). Most immune-related lncRNAs were identified in the Antimicrobials, Cytokines, Natural Killer Cell Cytotoxicity, TCR signaling pathway, and Antigen Processing and Presentation categories, suggesting that these pathways could be potential therapeutic targets for treating DCM. Next, differential analysis was performed on the lncRNA expression profiles of dilated cardiomyopathy (DCM) and normal myocardial tissue. Using screening criteria of absolute logFC greater than 0.5 and FDR less than 0.05, a total of 1033 differentially expressed genes were identified, among which 545 were upregulated and 488 were downregulated (Fig. 1C). The intersection between immune-related lncRNAs and the differentially expressed lncRNAs revealed 281 immune-related differential lncRNAs, which were found to be statistically significant by Fisher’s exact test (Fig. 1D). These results suggest that the majority of differentially expressed lncRNAs are involved in immune regulation in dilated cardiomyopathy.
Figure 1: Identification of immune-related lncRNAs in dilated cardiomyopathy. (A) Immune cell infiltration in dilated cardiomyopathy and normal cardiac tissue. (B) Distribution and enrichment of lncRNAs among different immune pathways. (C) Heatmap of differentially expressed lncRNAs between dilated cardiomyopathy and normal cardiac tissue. (D) Relationship between immune-related lncRNAs and differentially expressed lncRNAs.
Identification of hub immune-related lncRNAs
Three different machine learning algorithms were used to construct a diagnostic prediction model for dilated cardiomyopathy (DCM). Using the LASSO algorithm, 45 genes were screened (Fig. 2A). Based on SVM-RFE, 36 genes were determined to be the best candidate genes for DCM (Fig. 2B). By employing random forest, a set of 38 genes were identified as diagnostic genes (Fig. 2C). Based on the intersection of the SVM-RFE, LASSO, and RF models, 10 hub immune-related lncRNAs were identified (DCXR-DT, SCIRT, FNDC1-AS1, FHAD1-AS1, C10orf71-AS1, LOC101928834, GDNF-AS1, C3orf36, MELTF-AS1 and LOC107985323; Fig. 2D). Significant increases were observed in the expression levels of C10orf71-AS1, FHAD1-AS1, SCIRT, FNDC1-AS1, MELTF-AS1, LOC101928834, and GDNF-AS1 in dilated cardiomyopathy, whereas DCXR-DT, C3orf36, and LOC107985323 were significantly reduced (Fig. 2E). Subsequently, we validated our findings using the GSE135055 database and found that, except for C10orf71-AS1, C3orf36, and DCXR-DT, all other lncRNAs showed significant differences (Fig. 2F). In order to further strengthen the robustness of our findings and enhance the accuracy of our results, we extended our validation efforts to include the GSE165303 dataset, which provides a comprehensive representation of both pathological and healthy cardiac states. Upon rigorous analysis of the GSE165303 dataset, we observed a compelling alignment with our earlier results. Notably, with the exception of C3orf36, which displayed some variability, all the other lncRNAs under investigation exhibited trends consistent with our initial findings. This congruence between our findings across multiple datasets underscores the reliability and significance of our research outcomes (Suppl. Fig. S1). Based on the aforementioned findings, subsequent analysis was performed using the 10 identified key immune-related lncRNAs.
Figure 2: Selection of hub immune-related lncRNAs in dilated cardiomyopathy. (A) Ten-fold cross-validation and minimum feature gene coefficients in the LASSO model. (B) Ten-fold cross-validation of gene number and model error using the SVM-RFE algorithm. (C) Ranking of lncRNA importance in the random forest. (D) Relationship between LASSO, SVM-RFE, and random forest feature genes. (E) Expression changes in key immune-related lncRNAs in different groups. (F) Expression patterns of key immune-related lncRNAs in dilated cardiomyopathy and normal cardiac tissue in the GSE135055 dataset. *p < 0.05, **p < 0.01, ***p < 0.001, ****p < 0.0001.
The diagnostic values of the hub immune-related lncRNAs
The diagnostic values of the 10 identified key immune-related lncRNAs in dilated cardiomyopathy were evaluated separately. ROC analysis revealed that the areas under the curve (AUCs) for FNDC1-AS1, FHAD1-AS1, SCIRT, C10orf71-AS1, LOC101928834, MELTF-AS1, GDNF-AS1, LOC107985323, DCXR-DT, and C3ORF36 were 0.9439, 0.9618, 0.9102, 0.882, 0.8716, 0.896, 0.8541, 0.918, 0.805, and 0.8509, respectively, indicating good predictive performance of the hub immune-related lncRNAs (Figs. 3A–3J, Table 2).
Figure 3: Diagnostic value of key immune-related lncRNAs in dilated cardiomyopathy. ROC curve analysis for (A) FNDC1-AS1, (B) FHAD1-AS1, (C) SCIRT, (D) C10orf71-AS1, (E) LOC101928834, (F) MELTF-AS1, (G) GDNF-AS1, (H) LOC107985323, (I) DCXR-DT, and (J) C3ORF36 in GSE14190. The blue area represents the 95% confidence interval of the AUC.
The diagnostic value of the aforementioned lncRNAs was also confirmed by GSE135055 data (Suppl. Fig. S2). To further explore these 10 immune-related lncRNAs, a nomogram model was constructed based on these genes (Fig. 4A). The calibration curve showed good agreement between the nomogram model based on the 10 immune-related lncRNAs and the ideal model (Fig. 4B). DCA showed that although both the nomogram model and individual diagnostic-related lncRNAs produced net benefits, the net benefits of the nomogram model were significantly greater than those of individual diagnostic-related lncRNAs, indicating that the nomogram model may have greater clinical value than individual diagnostic-related lncRNAs (Fig. 4C). In addition, the diagnostic efficacy of the model reached 100% (Fig. 4D). External validation using GSE135055 showed that the AUC area of the column chart model based on the 10 genes reached 99.8%, reflecting the clinical application value of the predictive model (Figs. 4E, 4F, Suppl. Fig. S3).
Figure 4: Nomogram model for predicting dilated cardiomyopathy based on hub immune-related lncRNAs in the GSE14190 dataset, a predictive nomogram model for dilated cardiomyopathy based on key immune-related lncRNAs (A). The model’s prediction accuracy using a calibration curve (B), and its clinical benefit demonstrated by decision curve analysis (C). The ROC curve used to assess the model's predictive ability (D). In the GSE135055 dataset, a predictive model for dilated cardiomyopathy was developed based on hub immune-related lncRNAs (E), and its diagnostic performance was validated through ROC analysis (F).
The clinical values of the hub immune-related lncRNAs
We conducted an in-depth analysis of the clinical values of the hub immune-related lncRNAs, with a particular focus on their relationship with ejection fraction. Correlation analysis showed that FNDC1-AS1, FHAD1-AS1, SCIRT, LOC101928834, MELTF-AS1, GDNF-AS1, and C10orf71-AS1 were significantly negatively correlated with left ventricular ejection fraction, with correlation coefficients of −0.63, −0.58, −0.58, −0.47, −0.61, 0.43, and −0.63, respectively (all p < 0.05). LOC107985323, DCXR-DT, C3ORF36, and nomoscore were significantly positively correlated with left ventricular ejection fraction, with correlation coefficients of 0.71, 0.48, 0.49, and 0.75, respectively (all p < 0.05), indicating the clinical value of the key immune-related lncRNAs and risk score (Figs. 5A–5K). The mutual correlation between the key immune-related lncRNAs, risk score, and left ventricular ejection fraction was further analyzed, revealing that GDNF-AS1 had the strongest positive correlation with SCIRT among the lncRNAs, while the nomoscore had the strongest negative correlation with FHAD1-AS1 (Fig. 5L).
Figure 5: Correlation analysis between key immune-related lncRNAs and ejection fraction. (A) FNDC1-AS1, (B) FHAD1-AS1, (C) SCIRT, (D) C10orf71-AS1, (E) LOC101928834, (F) MELTF-AS1, (G) GDNF-AS1, (H) LOC107985323, (I) DCXR-DT, (J) C3ORF36, and (K) nomogram score analyzed for their correlation with left ventricular ejection fraction. (L) Correlation analysis performed among key immune-related lncRNAs, nomogram score, and ejection fraction, where red indicates a positive correlation and blue indicates a negative correlation.
To further evaluate the independent predictive value of the key immune-related lncRNAs for left ventricular ejection fraction, univariate and multivariate analyses were performed (Table 3). In the crude model, each unit increase in FNDC1-AS1, FHAD1-AS1, SCIRT, C10orf71-AS1, LOC101928834, MELTF-AS1, and GDNF-AS1 was associated with a 29%, 40%, 12%, 3%, 28%, 19%, and 36% decrease in the left ventricular ejection fraction, respectively, while each unit increase in LOC107985323, DCXR-DT, and C3ORF36 was associated with a 17%, 9%, and 50% increase in the left ventricular ejection fraction, respectively. Similar trends were observed in Model I and Model II after adjusting for other confounding variables (Table 3).
The function of hub immune-related lncRNAs
To investigate the function of key immune-related lncRNAs, GSEA was performed based on their mRNA coexpression, revealing that these lncRNAs were all associated with the activation of immune-related pathways. For instance, FHAD1-AS1 exhibited a positive correlation with endogenous antigen processing and presentation, interferon-beta production, assembly of MHC protein complex, and regulation of cytotoxicity mediated by T-cells. On the other hand, FNDC1-AS1 played a role in the activation of CD8-positive, alpha-beta T-cells, assembly of peptide antigen with MHC protein complex, positive selection of thymic T-cells, and response to interferon-alpha (Suppl. Fig. S4). Afterward, we examined the connection between crucial lncRNAs associated with the immune system and immune infiltration and function. Our analysis revealed a notable association between these lncRNAs and the immune system's activation (Figs. 6A, 6B). For example, FNDC1-AS1 was upregulated in mature/memory/activated B cells, activated CD4/CD8 T cells, gamma delta T cells, CD56 dim natural killer cells, mast cells, monocytes, T follicular helper cells, 2 types of dendritic cells (plasmacytoid, immature), and showed a decreasing expression tendency in type 17 T helper cells (all p < 0.05). Next, WGCNA was used to further investigate the functions associated with the 10 key immune-related lncRNAs during the development of dilated cardiomyopathy. A soft threshold of 11 was chosen using the PickSoftThreshold function, resulting in the most efficient scale-free topology network and connectivity (Suppl. Fig. S5A–S5C). A hierarchical clustering algorithm was used to divide the clustering tree into 8 gene modules (Fig. 6C). The gray module (167 genes) showed the highest positive correlation with FNDC1-AS1 (R = 0.56), FHAD1-AS1 (R = 0.74), SCIRT (R = 0.68), C10orf71-AS1 (R = 0.64), LOC101928834 (R = 0.48), MELTF-AS1 (R = 0.58), and GDNF-AS1 (R = 0.63), while exhibiting the highest negative correlation with LOC107985323 (R = −0.56), DCXR-DT (R = −0.41), C3ORF36 (R = −0.48), and nomoscore (R = −0.85) (Fig. 6D, Suppl. Table S2). Therefore, genes within the gray module were further analyzed. GO and KEGG enrichment analyses revealed that the gray module-related genes participated in various signaling pathways, such as cytokine-cytokine receptor interaction, chemotaxis, negative regulation of cell population proliferation, regulation of immune effector process, and regulation of leukocyte activation (Fig. 6E).
Figure 6: Functional analysis of hub immune-related lncRNAs based on WGCNA. (A) Correlation analysis between immune-related lncRNAs and immune cell infiltration and (B) immune cell function. (C) Gene dendrogram obtained by hierarchical clustering, with 8 modules identified by Dynamic Tree Cut. (D) Correlation analysis between immune-related lncRNAs and identified modules. (E) GO and KEGG enrichment analysis for the gray module gene.
The identification and functional exploration of subtypes based on hub immune-related lncRNAs
Unsupervised k-means clustering analysis was utilized to identify immune-related expression patterns in the immune microenvironment of DCM. The expression of 10 immune-related lncRNAs in the myocardial tissue of 166 DCM patients was used to classify them into different groups. Based on the clustering results, CDF plots, relative changes in CDF curve area, and tracking plots, k = 2 was selected as the optimal value, dividing the 166 patients into two distinct subtypes: subtype 1 with 113 samples and subtype 2 with 53 samples (Figs. 7A–7D). PCA demonstrated that the classification based on the 10 immune-related lncRNAs significantly differentiated subtype 1 from subtype 2 (Fig. 7E).
Figure 7: Construction of dilated cardiomyopathy subtypes based on hub immune-related lncRNAs. (A) Heatmap of the consensus matrix at k = 2 for the similarity between samples. (B) The consensus cumulative distribution function (CDF) curves at k = 2–9, and (C) the relative changes in CDF area to determine the optimal number of clusters. (D) The tracking plot showing the sample classification at k = 2–9. (E) The PCA plots showing the dilated cardiomyopathy specimens categorized into two immune subtypes (C1, C2) based on the expression profiling of hub immune-related lncRNAs.
Boxplot analysis showed that FNDC1-AS1, FHAD1-AS1, SCIRT, C10orf71-AS1, MELTF-AS1, and DCXR-DT were upregulated in subtype 2, while GDNF-AS1 and C3ORF36 were downregulated (Fig. 8A). ssGSEA revealed that immune cell abundance was generally lower in subtype 2 than in subtype 1 (Fig. 8B). Furthermore, the differential expression of 27 immune checkpoint genes was observed between the two subtypes, with TNFRSF14 and TNFRSF4 being upregulated in subtype 2 and the remaining differentially expressed genes being downregulated in subtype 2 (Fig. 8C).
Figure 8: Differences in immune function between immune subtypes. (A) Distribution of hub immune-related lncRNAs, (B) immune cell infiltration, and (C) immune checkpoints between the two immune subtypes. *p < 0.05, **p < 0.01, ***p < 0.001.
These findings suggest that the immune microenvironment differs significantly between the two subtypes identified based on the expression of key immune-related lncRNAs. GSVA using MSigDB revealed that biological functions (GO) such as cell proliferation, transcription factor activation, and DNA polymerization were significantly upregulated in subtype 1 compared to subtype 2. KEGG analysis showed that polysaccharide biosynthesis, the TGF-β signaling pathway, cell apoptosis, cell adhesion/maturity, and transcription factor activation were significantly upregulated in subtype 1 (Figs. 9A, 9B).
Figure 9: Differences in GO (A) and KEGG (B) pathways between immune subtypes.
Previous studies have proven that as a chronic inflammatory disease, DCM has a very complex molecular basis (Merlo et al., 2018; Smith et al., 2020). Pathological cardiac remodeling and cardiac dysfunction during the development of DCM mainly originate from chronic or persistent cardiac inflammation (Zeng et al., 2020; Caragnano et al., 2019). The survival rate of patients greatly depends on timely detection and predictive evaluation. Therefore, the development of molecular diagnosis and prognostic methods related to inflammation is of great significance to the risk stratification of DCM. In view of the fact that immune-mediated inflammatory injury is a key pathogenic factor in the development of DCM (Harding et al., 2023), it is highly important to uncover the significance of immune-associated molecular biomarkers in diagnosing and treating DCM. To fully understand the role of the immune response in DCM, we used ssGSEA to analyze the infiltration of immune cells in the sample. The results showed that the adaptive immune system composed of B cells and T cells was enriched in DCM, while innate immune cells such as dendritic cells and macrophages were significantly decreased in DCM. The difference in this enrichment trend may be determined by the different functions of the cells themselves. It has been reported that adaptive immunity triggered by innate immunity can expand the immune response to enhance inflammatory circulation (Chaplin, 2010). Dendritic cells are important mediators of the protective immune response (Liu and Nussenzweig, 2010). This demonstrates the significant involvement of B cells and T cells in the control of cardiac inflammation and damage. It has been found that cytotoxic T cells and CD4+ T cells enriched in damaged tissues can promote injury and mediate the development of heart failure (Swirski and Nahrendorf, 2018; Bansal et al., 2017; Nevers et al., 2015). In addition, B-cell infiltration can mediate the inflammatory response and aggravate cardiac function injury (Cordero-Reyes et al., 2016). The above results are consistent with our findings, which show that the adaptive immune response is very important for maintaining the inflammatory environment of DCM tissue.
To identify the changes in immune-related pathway molecules in DCM, we obtained 281 immune-related lncRNAs with significant differences in DCM. These characteristic lncRNAs may be involved in the development of DCM. Based on the prediction model constructed by three machine learning algorithms, we finally selected 10 immune-related lncRNAs (C10orf71-AS1, FHAD1-AS1, SCIRT, FNDC1-AS1, MELTF-AS1, LOC101928834, GDNF-AS1, DCXR-DT, C3orf36 and LOC107985323). These lncRNAs showed significant differences between DCM and NF groups (Fig. 2E, Suppl. Fig. S6A). From our data, it appears that DCM has a slightly higher prevalence among males, suggesting that sex differences exist. To investigate whether sex could act as a factor influencing lncRNA selection, we examined the impact of sex differences on these lncRNAs. The findings revealed that sex had no significant effect on the results. This indicates that the outcomes could be equally applicable to both males and females (Suppl. Fig. S6B–C). Although the role and mechanism of the selected characteristic lncRNA in DCM have not been verified, some studies have confirmed the role of some lncRNAs in immune regulation. For example, immune-related FNDC1-AS1 is associated with the prognosis of pancreatic cancer (Ma et al., 2022). MELTF-AS1 is associated with the prognosis of renal clear cell carcinoma (Jiang et al., 2020). In addition, LOC101928834 is associated with poor clinical prognosis of myelodysplastic syndrome (Li et al., 2020b). These findings suggest that there may be some similarities in immunomodulatory responses between cancer and noncancer tissues. By combining the datasets GSE135055 and GSE165303, our analysis encompasses expression profiles from 60 normal myocardial tissues and 68 DCM myocardial tissues. Within these datasets, we have identified several lncRNAs exhibiting significant differences. This serves as preliminary evidence, confirming the effectiveness of our screening results. To evaluate the role of lncRNAs in DCM, we analyzed the ROC curve of the diagnostic model test set including the above 10 lncRNAs. The results showed that the 10 immune-related lncRNAs had good predictive performance. These results were also confirmed in GSE135055. Then, based on these characteristic immune-related lncRNAs, we established a nomogram model to predict the risk of DCM. The high similarity of the calibration curve and DCA analysis results shows that the model has good predictive performance and clinical diagnostic value. The results of validating the diagnostic model with the GSE135055 cohort show that the model has good clinical applicability. In addition, we also found that FNDC1-AS1, FHAD1-AS1, SCIRT, LOC101928834, MELTF-AS1, GDNF-AS1 and C10orf71-AS1 were significantly negatively correlated with the left ventricular ejection fraction, while LOC107985323, DCXR-DT, C3ORF36 and the nomoscore were positively correlated with the left ventricular ejection fraction, indicating that they may play different roles in regulating immune activation. Next, we characterized the biological functions of these key lncRNAs. WGCNA, GO and KEGG analyses showed that these lncRNAs were related to the activation of immune-related pathways, which mainly includes the process of immune effect and the regulation of leukocyte activation. Based on the results of the entire study, the gene with strong clinical discrimination is C10orf71-AS1. Previous research has shown that C10orf71-AS1 is associated with the pathological development of tendinopathy, involving molecular processes and pathways such as focal adhesion, ECM-receptor interaction, and the PI3K-Akt pathway (Huang et al., 2022). Furthermore, this investigation also uncovered that the target genes of C10orf71-AS1 are specifically associated with the contraction of cardiac muscle, subsequent to adrenergic signaling in cardiomyocytes, and the pathway of calcium signaling (Huang et al., 2022). Changes in these functions and signaling pathways are crucial contributors to the progression of DCM (Schultheiss et al., 2019). This suggests that C10orf71-AS1 may exert its influence on the occurrence of DCM by affecting these features. In fact, our GSEA results also revealed that C10orf71-AS1 is associated with the interleukin-1 mediated pathway and fibroblast migration (Suppl. Fig. S4G). Notably, the alterations in IL-1β and fibroblast phenotypes are closely linked to the occurrence and progression of DCM (Liu et al., 2015b; Tsuru et al., 2023). This further suggests that C10orf71-AS1 may independently serve as a discriminator for DCM.
To further elucidate the functional traits of lncRNAs, we created two genotypes based on the expression profile of immune-associated lncRNAs in DCM. In comparison to the C2 subtype, the C1 subtype exhibited greater enrichment of immune cells and higher expression of immune checkpoints. Furthermore, the C1 and C2 subtypes exhibited notable disparities in pathway enrichment, as evidenced by the KEGG and GO analyses. Typing based on different expression levels of immune-related lncRNAs can reflect different immune microenvironments, which may be helpful for the early diagnosis and classification of DCM. Although we have verified the diagnostic value of immune-related lncRNAs in DCM, our research still has some limitations. For instance, within the scope of this study, the confirmation of immune-related lncRNA functionality requires validation across additional verification cohorts. This validation is essential for a more comprehensive understanding of its clinical significance in DCM. Another limitation is that the utilized GEO dataset, although containing crucial clinical information like left ventricular ejection fraction and heart weight, still lacks certain clinical and biochemical details about the donors. For instance, specifics regarding whether patients experienced infections and the concentration of pro-NT-BNP have not been provided. This affects the comprehensive assessment of DCM patient characteristics. Furthermore, this study lacks laboratory data, and external experiments are needed to prove the functional mechanism of lncRNAs.
Overall, our study has identified 10 immune-related lncRNAs that show potential for further investigation in DCM diagnosis and clustering. In addition, according to the expression characteristics of the selected lncRNA, we established two important DCM types. Our study may contribute to the early diagnosis and classification of DCM and provide personalized services for patients.
Acknowledgement: None.
Funding Statement: This study was funded by the Chinese National Natural Science Foundation (No. 12072215), Science and Technology Department of Sichuan Province (2021YFS0120 and 2023NSFSC1640), Chinese Postdoctoral Science Foundation (2022M722278), Chunhui Program of Ministry of Education of China (No. HZKY20220573).
Author Contributions: The authors confirm contribution to the paper as follows: BC and ZJ designed the study. ZJ, ZS and TH collected the GEO information and expression data. KQ and ZJ analyzed data and wrote the manuscript. LX reviewed and edited the manuscript. All authors contributed to the article and approved the submitted version.
Availability of Data and Materials: The datasets generated during and/or analysed during the current study are available from the corresponding author on reasonable request.
Ethics Approval: Not applicable.
Conflicts of Interest: The authors declare that they have no conflicts of interest to report regarding the present study.
Supplementary Materials: The supplementary material is available online at https://doi.org/10.32604/biocell.2023.043864.
References
Ampong I (2022). Metabolic and metabolomics insights into dilated cardiomyopathy. Annals of Nutrition and Metabolism 78: 147–155. https://doi.org/10.1159/000524722 [Google Scholar] [PubMed] [CrossRef]
Bansal SS, Ismahil MA, Goel M, Patel B, Hamid T, Rokosh G, Prabhu SD (2017). Activated T lymphocytes are essential drivers of pathological remodeling in ischemic heart failure. Circulation: Heart Failure 10: e003688. https://doi.org/10.1161/CIRCHEARTFAILURE.116.003688 [Google Scholar] [PubMed] [CrossRef]
Bian W, Wang Z, Li X, Jiang XX, Liu Z, Zhang DM (2022). Identification of vital modules and genes associated with heart failure based on weighted gene coexpression network analysis. ESC Heart Failure 9: 1370–1379. https://doi.org/10.1002/ehf2.13827 [Google Scholar] [PubMed] [CrossRef]
Caragnano A, Aleksova A, Bulfoni M, Cervellin C, Rolle IG et al. (2019). Autophagy and inflammasome activation in dilated cardiomyopathy. Journal of Clinical Medicine 8: 1519. https://doi.org/10.3390/jcm8101519 [Google Scholar] [PubMed] [CrossRef]
Chaplin DD (2010). Overview of the immune response. Journal of Allergy and Clinical Immunology 125: S3–S23. https://doi.org/10.1016/j.jaci.2009.12.980 [Google Scholar] [PubMed] [CrossRef]
Cordero-Reyes AM, Youker KA, Trevino AR, Celis R, Hamilton DJ, Flores-Arredondo JH, Orrego CM, Bhimaraj A, Estep JD, Torre-Amione G (2016). Full expression of cardiomyopathy is partly dependent on B-cells: A pathway that involves cytokine activation, immunoglobulin deposition, and activation of apoptosis. Journal of the American Heart Association 5: e002484. https://doi.org/10.1161/JAHA.115.002484 [Google Scholar] [PubMed] [CrossRef]
Costa MC, Calderon-Dominguez M, Mangas A, Campuzano O, Sarquella-Brugada G et al. (2021). Circulating circRNA as biomarkers for dilated cardiomyopathy etiology. Journal of Molecular Medicine 99: 1711–1725. https://doi.org/10.1007/s00109-021-02119-6 [Google Scholar] [PubMed] [CrossRef]
Du X, Liu M, Su J, Zhang P, Tang F et al. (2018). Uncoupling therapeutic from immunotherapy-related adverse effects for safer and effective anti-CTLA-4 antibodies in CTLA4 humanized mice. Cell Research 28: 433–447. https://doi.org/10.1038/s41422-018-0012-z [Google Scholar] [PubMed] [CrossRef]
Elamm C, Fairweather D, Cooper LT (2012). Pathogenesis and diagnosis of myocarditis. Heart 98: 835–840. https://doi.org/10.1136/heartjnl-2012-301686 [Google Scholar] [PubMed] [CrossRef]
Ellis K, Kerr J, Godbole S, Lanckriet G, Wing D, Marshall S (2014). A random forest classifier for the prediction of energy expenditure and type of physical activity from wrist and hip accelerometers. Physiological Measurement 35: 2191–2203. https://doi.org/10.1088/0967-3334/35/11/2191 [Google Scholar] [PubMed] [CrossRef]
Engebretsen S, Bohlin J (2019). Statistical predictions with glmnet. Clinical Epigenetics 11: 123. https://doi.org/10.1186/s13148-019-0730-1 [Google Scholar] [PubMed] [CrossRef]
Fan J, Li H, Xie R, Zhang X, Nie X et al. (2021). LncRNA ZNF593-AS alleviates contractile dysfunction in dilated cardiomyopathy. Circulation Research 128: 1708–1723. https://doi.org/10.1161/CIRCRESAHA.120.318437 [Google Scholar] [PubMed] [CrossRef]
Felix SB, Beug D, Dorr M (2015). Immunoadsorption therapy in dilated cardiomyopathy. Expert Review of Cardiovascular Therapy 13: 145–152. https://doi.org/10.1586/14779072.2015.990385 [Google Scholar] [PubMed] [CrossRef]
Feng Y, Cai L, Hong W, Zhang C, Tan N et al. (2022). Rewiring of 3D chromatin topology orchestrates transcriptional reprogramming and the development of human dilated cardiomyopathy. Circulation 145: 1663–1683. https://doi.org/10.1161/CIRCULATIONAHA.121.055781 [Google Scholar] [PubMed] [CrossRef]
Flam E, Jang C, Murashige D, Yang Y, Morley MP et al. (2022). Integrated landscape of cardiac metabolism in end-stage human nonischemic dilated cardiomyopathy. Nature Cardiovascular Research 1: 817–829. https://doi.org/10.1038/s44161-022-00117-6 [Google Scholar] [PubMed] [CrossRef]
Harding D, Chong MHA, Lahoti N, Bigogno CM, Prema R, Mohiddin SA, Marelli-Berg F (2023). Dilated cardiomyopathy and chronic cardiac inflammation: Pathogenesis, diagnosis and therapy. Journal of Internal Medicine 293: 23–47. https://doi.org/10.1111/joim.13556 [Google Scholar] [PubMed] [CrossRef]
Hershberger RE, Hedges DJ, Morales A (2013). Dilated cardiomyopathy: The complexity of a diverse genetic architecture. Nature Reviews Cardiology 10: 531–547. https://doi.org/10.1038/nrcardio.2013.105 [Google Scholar] [PubMed] [CrossRef]
Hua X, Wang YY, Jia P, Xiong Q, Hu Y, Chang Y, Lai S, Xu Y, Zhao Z, Song J (2020). Multi-level transcriptome sequencing identifies COL1A1 as a candidate marker in human heart failure progression. BMC Medicine 18: 2. https://doi.org/10.1186/s12916-019-1469-4 [Google Scholar] [PubMed] [CrossRef]
Huang BZ, Yang JJ, Dong XM, Zhuan Z, Liu XN (2022). Analysis of the lncRNA-associated competing endogenous RNA (ceRNA) network for tendinopathy. Genetics Research 2022: 9792913. https://doi.org/10.1155/2022/9792913 [Google Scholar] [PubMed] [CrossRef]
Jiang Y, Gou X, Wei Z, Tan J, Yu H, Zhou X, Li X (2020). Bioinformatics profiling integrating a three immune-related long non-coding RNA signature as a prognostic model for clear cell renal cell carcinoma. Cancer Cell International 20: 166. https://doi.org/10.1186/s12935-020-01242-7 [Google Scholar] [PubMed] [CrossRef]
Lakdawala NK, Winterfield JR, Funke BH (2013). Dilated cardiomyopathy. Circulation: Arrhythmia and Electrophysiology 6: 228–237. https://doi.org/10.1161/CIRCEP.111.962050 [Google Scholar] [PubMed] [CrossRef]
Li H, Chen C, Fan J, Yin Z, Ni L, Cianflone K, Wang Y, Wang DW (2018). Identification of cardiac long non-coding RNA profile in human dilated cardiomyopathy. Cardiovascular Research 114: 747–758. https://doi.org/10.1093/cvr/cvy012 [Google Scholar] [PubMed] [CrossRef]
Li Y, Jiang T, Zhou W, Li J, Li X et al. (2020a). Pan-cancer characterization of immune-related lncRNAs identifies potential oncogenic biomarkers. Nature Communications 11: 1000. https://doi.org/10.1038/s41467-020-14802-2 [Google Scholar] [PubMed] [CrossRef]
Li N, Ma Y, Wang W, Yin CC, Wu W, Sun R, Zhao G, Li S, Wang X (2020b). LOC101928834, a novel lncRNA in Wnt/β-catenin signaling pathway, promotes cell proliferation and predicts poor clinical outcome in myelodysplastic syndromes. Clinical Science 134: 1279–1293. https://doi.org/10.1042/CS20200439 [Google Scholar] [PubMed] [CrossRef]
Liu C, Liu J, Wu D, Luo S, Li W, Chen L, Liu Z, Yu B (2022). Construction of immune-related ceRNA network in dilated cardiomyopathy: Based on sex differences. Frontiers in Genetics 13: 882324. https://doi.org/10.3389/fgene.2022.882324 [Google Scholar] [PubMed] [CrossRef]
Liu Y, Morley M, Brandimarto J, Hannenhalli S, Hu Y et al. (2015a). RNA-seq identifies novel myocardial gene expression signatures of heart failure. Genomics 105: 83–89. https://doi.org/10.1016/j.ygeno.2014.12.002 [Google Scholar] [PubMed] [CrossRef]
Liu K, Nussenzweig MC (2010). Origin and development of dendritic cells. Immunological Reviews 234: 45–54. https://doi.org/10.1111/j.0105-2896.2009.00879.x [Google Scholar] [PubMed] [CrossRef]
Liu Z, Zhao N, Zhu H, Zhu S, Pan S, Xu J, Zhang X, Zhang Y, Wang J (2015b). Circulating interleukin-1β promotes endoplasmic reticulum stress-induced myocytes apoptosis in diabetic cardiomyopathy via interleukin-1 receptor-associated kinase-2. Cardiovascular Diabetology 14: 125. https://doi.org/10.1186/s12933-015-0288-y [Google Scholar] [PubMed] [CrossRef]
Long J, Liu Z, Wu X, Xu Y, Ge C (2016). Identification of disease-associated pathways in pancreatic cancer by integrating genome-wide association study and gene expression data. Oncology Letters 12: 537–543. https://doi.org/10.3892/ol.2016.4637 [Google Scholar] [PubMed] [CrossRef]
Ma Y, He X, Di Y, Liu S, Zhan Q, Bai Z, Qiu T, Corpe C, Wang J (2022). Identification of prognostic immune-related lncRNAs in pancreatic cancer. Frontiers in Immunology 13: 1005695. https://doi.org/10.3389/fimmu.2022.1005695 [Google Scholar] [PubMed] [CrossRef]
Mahon NG, Madden BP, Caforio AL, Elliott PM, Haven AJ, Keogh BE, Davies MJ, Mckenna WJ (2002). Immunohistologic evidence of myocardial disease in apparently healthy relatives of patients with dilated cardiomyopathy. Journal of the American College of Cardiology 39: 455–462. https://doi.org/10.1016/S0735-1097(01)01762-4 [Google Scholar] [PubMed] [CrossRef]
McDonagh TA, Metra M, Adamo M, Gardner RS, Baumbach A et al. (2021). 2021 ESC Guidelines for the diagnosis and treatment of acute and chronic heart failure. European Heart Journal 42: 3599–3726. https://doi.org/10.1093/eurheartj/ehab368 [Google Scholar] [PubMed] [CrossRef]
Merlo M, Cannata A, Gobbo M, Stolfo D, Elliott PM, Sinagra G (2018). Evolving concepts in dilated cardiomyopathy. European Journal of Heart Failure 20: 228–239. https://doi.org/10.1002/ejhf.1103 [Google Scholar] [PubMed] [CrossRef]
Miyamoto SD, Karimpour-Fard A, Peterson V, Auerbach SR, Stenmark KR, Stauffer BL, Sucharov CC (2015). Circulating microRNA as a biomarker for recovery in pediatric dilated cardiomyopathy. Journal of Heart and Lung Transplantation 34: 724–733. https://doi.org/10.1016/j.healun.2015.01.979 [Google Scholar] [PubMed] [CrossRef]
Nevers T, Salvador AM, Grodecki-Pena A, Knapp A, Velazquez F, Aronovitz M, Kapur NK, Karas RH, Blanton RM, Alcaide P (2015). Left ventricular T-cell recruitment contributes to the pathogenesis of heart failure. Circulation: Heart Failure 8: 776–787. https://doi.org/10.1161/CIRCHEARTFAILURE.115.002225 [Google Scholar] [PubMed] [CrossRef]
Nojima T, Proudfoot NJ (2022). Mechanisms of lncRNA biogenesis as revealed by nascent transcriptomics. Nature Reviews Molecular Cell Biology 23: 389–406. https://doi.org/10.1038/s41580-021-00447-6 [Google Scholar] [PubMed] [CrossRef]
Portokallidou K, Dovrolis N, Ragia G, Atzemian N, Kolios G, Manolopoulos VG (2023). Multi-omics integration to identify the genetic expression and protein signature of dilated and ischemic cardiomyopathy. Frontiers in Cardiovascular Medicine 10: 1115623. https://doi.org/10.3389/fcvm.2023.1115623 [Google Scholar] [PubMed] [CrossRef]
Raghow R (2016). An ‘Omics’ perspective on cardiomyopathies and heart failure. Trends in Molecular Medicine 22: 813–827. https://doi.org/10.1016/j.molmed.2016.07.007 [Google Scholar] [PubMed] [CrossRef]
Reichart D, Magnussen C, Zeller T, Blankenberg S (2019). Dilated cardiomyopathy: From epidemiologic to genetic phenotypes: A translational review of current literature. Journal of Internal Medicine 286: 362–372. https://doi.org/10.1111/joim.12944 [Google Scholar] [PubMed] [CrossRef]
Robinson EK, Covarrubias S, Carpenter S (2020). The how and why of lncRNA function: An innate immune perspective. Biochimica et Biophysica Acta (BBA)-Gene Regulatory Mechanisms 1863: 194419. https://doi.org/10.1016/j.bbagrm.2019.194419 [Google Scholar] [PubMed] [CrossRef]
Sanz H, Valim C, Vegas E, Oller JM, Reverter F (2018). SVM-RFE: Selection and visualization of the most relevant features through non-linear kernels. BMC Bioinformatics 19: 432. https://doi.org/10.1186/s12859-018-2451-4 [Google Scholar] [PubMed] [CrossRef]
Schultheiss HP, Fairweather D, Caforio ALP, Escher F, Hershberger RE et al. (2019). Dilated cardiomyopathy. Nature Reviews Disease Primers 5: 32. https://doi.org/10.1038/s41572-019-0084-1 [Google Scholar] [PubMed] [CrossRef]
Smith ED, Lakdawala NK, Papoutsidakis N, Aubert G, Mazzanti A et al. (2020). Desmoplakin cardiomyopathy, a fibrotic and inflammatory form of cardiomyopathy distinct from typical dilated or arrhythmogenic right ventricular cardiomyopathy. Circulation 141: 1872–1884. https://doi.org/10.1161/CIRCULATIONAHA.119.044934 [Google Scholar] [PubMed] [CrossRef]
Swirski FK, Nahrendorf M (2018). Cardioimmunology: The immune system in cardiac homeostasis and disease. Nature Reviews Immunology 18: 733–744. https://doi.org/10.1038/s41577-018-0065-8 [Google Scholar] [PubMed] [CrossRef]
Tsuru H, Yoshihara C, Suginobe H, Matsumoto M, Ishii Y et al. (2023). Pathogenic roles of cardiac fibroblasts in pediatric dilated cardiomyopathy. Journal of the American Heart Association 12: e029676. https://doi.org/10.1161/JAHA.123.029676 [Google Scholar] [PubMed] [CrossRef]
Weintraub RG, Semsarian C, Macdonald P (2017). Dilated cardiomyopathy. Lancet 390: 400–414. https://doi.org/10.1016/S0140-6736(16)31713-5 [Google Scholar] [PubMed] [CrossRef]
Zeng C, Duan F, Hu J, Luo B, Huang B et al. (2020). NLRP3 inflammasome-mediated pyroptosis contributes to the pathogenesis of non-ischemic dilated cardiomyopathy. Redox Biology 34: 101523. https://doi.org/10.1016/j.redox.2020.101523 [Google Scholar] [PubMed] [CrossRef]
Zhang X, Nie X, Yuan S, Li H, Fan J et al. (2019). Circulating long non-coding RNA ENST00000507296 is a prognostic indicator in patients with dilated cardiomyopathy. Molecular Therapy-Nucleic Acids 16: 82–90. https://doi.org/10.1016/j.omtn.2019.02.004 [Google Scholar] [PubMed] [CrossRef]
Zhang XZ, Zhang S, Tang TT, Cheng X (2021). Bioinformatics and immune infiltration analyses reveal the key pathway and immune cells in the pathogenesis of hypertrophic cardiomyopathy. Frontiers in Cardiovascular Medicine 8: 696321. https://doi.org/10.3389/fcvm.2021.696321 [Google Scholar] [PubMed] [CrossRef]
Zhao L, Fu Z (2018). Roles of host immunity in viral myocarditis and dilated cardiomyopathy. Journal of Immunological Research 2018: 5301548. https://doi.org/10.1155/2018/5301548 [Google Scholar] [PubMed] [CrossRef]
Zheng Y, Liu Z, Yang X, Weng S, Xu H et al. (2022). Exploring key genes to construct a diagnosis model of dilated cardiomyopathy. Frontiers in Cardiovascular Medicine 9: 865096. https://doi.org/10.3389/fcvm.2022.865096 [Google Scholar] [PubMed] [CrossRef]
Zhou J, Tian G, Quan Y, Kong Q, Huang F, Li J, Wu W, Tang Y, Zhou Z, Liu X (2023). The long noncoding RNA THBS1-AS1 promotes cardiac fibroblast activation in cardiac fibrosis by regulating TGFBR1. JCI Insight 8: e160745. https://doi.org/10.1172/jci.insight.160745 [Google Scholar] [PubMed] [CrossRef]
Cite This Article
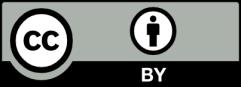