Open Access
ARTICLE
PSTCNN: Explainable COVID-19 diagnosis using PSO-guided self-tuning CNN
1 School of Computing and Mathematical, University of Leicester, Leicester, LE1 7RH, UK
2 Huai’an Tongji Hospital, Huai’an, 223000, China
3 Department of Signal Theory, Networking and Communications, University of Granada, Granada, 52005, Spain
* Corresponding Author: YU-DONG ZHANG. Email:
# These authors contributed to the work equally and should be regarded as co-first authors
(This article belongs to the Special Issue: Efficient Evolutionary and Swarm Intelligence Algorithms for Biological Applications)
BIOCELL 2023, 47(2), 373-384. https://doi.org/10.32604/biocell.2023.025905
Received 05 August 2022; Accepted 04 September 2022; Issue published 18 November 2022
Abstract
Since 2019, the coronavirus disease-19 (COVID-19) has been spreading rapidly worldwide, posing an unignorable threat to the global economy and human health. It is a disease caused by severe acute respiratory syndrome coronavirus 2, a single-stranded RNA virus of the genus Betacoronavirus. This virus is highly infectious and relies on its angiotensin-converting enzyme 2-receptor to enter cells. With the increase in the number of confirmed COVID-19 diagnoses, the difficulty of diagnosis due to the lack of global healthcare resources becomes increasingly apparent. Deep learning-based computer-aided diagnosis models with high generalisability can effectively alleviate this pressure. Hyperparameter tuning is essential in training such models and significantly impacts their final performance and training speed. However, traditional hyperparameter tuning methods are usually time-consuming and unstable. To solve this issue, we introduce Particle Swarm Optimisation to build a PSO-guided Self-Tuning Convolution Neural Network (PSTCNN), allowing the model to tune hyperparameters automatically. Therefore, the proposed approach can reduce human involvement. Also, the optimisation algorithm can select the combination of hyperparameters in a targeted manner, thus stably achieving a solution closer to the global optimum. Experimentally, the PSTCNN can obtain quite excellent results, with a sensitivity of 93.65% ± 1.86%, a specificity of 94.32% ± 2.07%, a precision of 94.30% ± 2.04%, an accuracy of 93.99% ± 1.78%, an F1-score of 93.97% ± 1.78%, Matthews Correlation Coefficient of 87.99% ± 3.56%, and Fowlkes-Mallows Index of 93.97% ± 1.78%. Our experiments demonstrate that compared to traditional methods, hyperparameter tuning of the model using an optimisation algorithm is faster and more effective.Keywords
Cite This Article
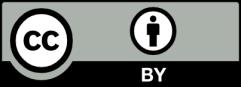