Open Access
ARTICLE
Performance of Lung Cancer Prediction Methods Using Different Classification Algorithms
Department of Computer Engineering, Engineering and Architecture Faculty, Kastamonu University, Kastamonu, 37200, Turkey
* Corresponding Author: Yasemin Gültepe. Email:
(This article belongs to the Special Issue: Machine Learning-based Intelligent Systems: Theories, Algorithms, and Applications)
Computers, Materials & Continua 2021, 67(2), 2015-2028. https://doi.org/10.32604/cmc.2021.014631
Received 04 October 2020; Accepted 14 December 2020; Issue published 05 February 2021
Abstract
In 2018, 1.76 million people worldwide died of lung cancer. Most of these deaths are due to late diagnosis, and early-stage diagnosis significantly increases the likelihood of a successful treatment for lung cancer. Machine learning is a branch of artificial intelligence that allows computers to quickly identify patterns within complex and large datasets by learning from existing data. Machine-learning techniques have been improving rapidly and are increasingly used by medical professionals for the successful classification and diagnosis of early-stage disease. They are widely used in cancer diagnosis. In particular, machine learning has been used in the diagnosis of lung cancer due to the benefits it offers doctors and patients. In this context, we performed a study on machine-learning techniques to increase the classification accuracy of lung cancer with 32 × 56 sized numerical data from the Machine Learning Repository web site of the University of California, Irvine. In this study, the precision of the classification model was increased by the effective employment of pre-processing methods instead of direct use of classification algorithms. Nine datasets were derived with pre-processing methods and six machine-learning classification methods were used to achieve this improvement. The study results suggest that the accuracy of the k-nearest neighbors algorithm is superior to random forest, naïve Bayes, logistic regression, decision tree, and support vector machines. The performance of pre-processing methods was assessed on the lung cancer dataset. The most successful pre-processing methods were Z-score (83% accuracy) for normalization methods, principal component analysis (87% accuracy) for dimensionality reduction methods, and information gain (71% accuracy) for feature selection methods.Keywords
Cite This Article
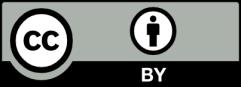