Open Access
ARTICLE
Automatic Vehicle License Plate Recognition Using Optimal Deep Learning Model
1 College of Computer Engineering and Sciences, Prince Sattam Bin Abdulaziz University, Al-Kharj, Saudi Arabia
2 Department of Computer Science & Engineering, IcfaiTech, ICFAI Foundation for Higher Education, Hyderabad, India
3 Assistant professor Research, Research Center, Lebanese French University, Erbil, 44001, Iraq
4 Department of Entrepreneurship and Logistics, Plekhanov Russian University of Economics, Moscow, 117997, Russia
5 Department of Logistics, State University of Management, Moscow, 109542, Russia
6 Department of Computer Applications, Alagappa University, Karaikudi, India
* Corresponding Author: K. Shankar. Email:
Computers, Materials & Continua 2021, 67(2), 1881-1897. https://doi.org/10.32604/cmc.2021.014924
Received 27 October 2020; Accepted 23 November 2020; Issue published 05 February 2021
Abstract
The latest advancements in highway research domain and increase inthe number of vehicles everyday led to wider exposure and attention towards the development of efficient Intelligent Transportation System (ITS). One of the popular research areas i.e., Vehicle License Plate Recognition (VLPR) aims at determining the characters that exist in the license plate of the vehicles. The VLPR process is a difficult one due to the differences in viewpoint, shapes, colors, patterns, and non-uniform illumination at the time of capturing images. The current study develops a robust Deep Learning (DL)-based VLPR model using Squirrel Search Algorithm (SSA)-based Convolutional Neural Network (CNN), called the SSA-CNN model. The presented technique has a total of four major processes namely preprocessing, License Plate (LP) localization and detection, character segmentation, and recognition. Hough Transform (HT) is applied as a feature extractor and SSA-CNN algorithm is applied for character recognition in LP. The SSA-CNN method effectively recognizes the characters that exist in the segmented image by optimal tuning of CNN parameters. The HT-SSA-CNN model was experimentally validated using the Stanford Car, FZU Car, and HumAIn 2019 Challenge datasets. The experimentation outcome verified that the presented method was better under several aspects. The projected HT-SSA-CNN model implied the best performance with optimal overall accuracy of 0.983%.Keywords
Cite This Article
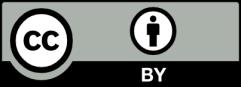