Open Access
ARTICLE
Brain Tumor Classification Based on Fine-Tuned Models and the Ensemble Method
1 Department of Information Technology, Malaysia University of Science and Technology, Selangor, 47810, Malaysia
2 Department of Information and Engineering Le2i Laboratory, University of Burgundy Dijon, 21078, France
3 Department of Information Technology Faculty of Computing and Information Technology King Abdulaziz, University Jeddah, 21589, Saudi Arabia
* Corresponding Author: Neelum Noreen. Email:
Computers, Materials & Continua 2021, 67(3), 3967-3982. https://doi.org/10.32604/cmc.2021.014158
Received 02 September 2020; Accepted 06 December 2020; Issue published 01 March 2021
Abstract
Brain tumors are life-threatening for adults and children. However, accurate and timely detection can save lives. This study focuses on three different types of brain tumors: Glioma, meningioma, and pituitary tumors. Many studies describe the analysis and classification of brain tumors, but few have looked at the problem of feature engineering. Methods are needed to overcome the drawbacks of manual diagnosis and conventional feature-engineering techniques. An automatic diagnostic system is thus necessary to extract features and classify brain tumors accurately. While progress continues to be made, the automatic diagnoses of brain tumors still face challenges of low accuracy and high false-positive results. The model presented in this study, which offers improvements for feature extraction and classification, uses deep learning and machine learning for the assessment of brain tumors. Deep learning is used for feature extraction and encompasses the application of different models such as fine-tuned Inception-v3 and fine-tuned Xception. The classification of brain tumors is explored through deep- and machine-learning algorithms including softmax, Random Forest, Support Vector Machine, K-Nearest Neighbors, and the ensemble technique. The results of these approaches are compared with existing methods. The Inception-v3 model has a test accuracy of 94.34% and achieves the highest performance compared with other recently reported methods. This improvement may be sufficient to support a significant role in clinical applications for brain tumor analysis. Furthermore, this type of approach can be used as an effective decision-support tool for radiologists in medical diagnostics as a second opinion based on the magnetic resonance imaging (MRI) analysis. It may also save valuable time for radiologists who have to manually review numerous MRI images of patients.Keywords
Cite This Article
Citations
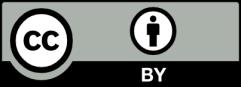