Open Access
ARTICLE
Stock Price Prediction Using Predictive Error Compensation Wavelet Neural Networks
1 Graduate School of Science Engineering and Technology, Istanbul Technical University, Istanbul, Turkey
2 Department of Computer Engineering, Istanbul Technical University, Istanbul, Turkey
* Corresponding Author: Ajla Kulaglic. Email:
Computers, Materials & Continua 2021, 68(3), 3577-3593. https://doi.org/10.32604/cmc.2021.014768
Received 14 October 2020; Accepted 09 March 2021; Issue published 06 May 2021
Abstract
Machine Learning (ML) algorithms have been widely used for financial time series prediction and trading through bots. In this work, we propose a Predictive Error Compensated Wavelet Neural Network (PEC-WNN) ML model that improves the prediction of next day closing prices. In the proposed model we use multiple neural networks where the first one uses the closing stock prices from multiple-scale time-domain inputs. An additional network is used for error estimation to compensate and reduce the prediction error of the main network instead of using recurrence. The performance of the proposed model is evaluated using six different stock data samples in the New York stock exchange. The results have demonstrated significant improvement in forecasting accuracy in all cases when the second network is used in accordance with the first one by adding the outputs. The RMSE error is 33% improved when the proposed PEC-WNN model is used compared to the Long Short-Term Memory (LSTM) model. Furthermore, through the analysis of training mechanisms, we found that using the updated training the performance of the proposed model is improved. The contribution of this study is the applicability of simultaneously different time frames as inputs. Cascading the predictive error compensation not only reduces the error rate but also helps in avoiding overfitting problems.Keywords
Cite This Article
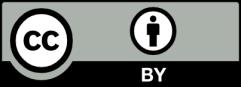